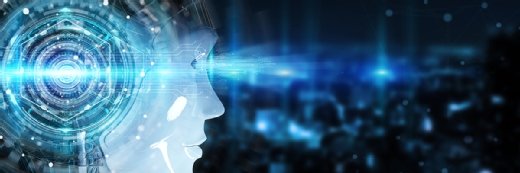
sdecoret - stock.adobe.com
6 machine learning applications for data center optimization
AI and machine learning have the potential to revolutionize data center operations. They can manage facilities more efficiently by optimizing power consumption and monitoring.
Machine learning and artificial intelligence are popular buzzwords for today's IT professionals, but in the case of your organization's data centers, they hold real promise.
ML software actively predicts situations faster than you or your team might notice them and maybe even solves them quicker. These systems are a logical extension of today's hybrid data center environment and are a growing part of data center infrastructure.
According to a 2022 Uptime Institute survey, 57% of data center operators trust AI and machine learning to perform routine operational decisions; many still only use it for power and cooling optimization and anomaly detection.
Seventy-three percent of respondents to a 2023 Uptime Institute survey believe AI-based software will reduce facility staffing levels, and 25% of those respondents believe it'll happen in five years. AI and machine learning in data centers can optimize much of your overall operations, including planning and design, workloads, uptime and cost management.
Use cases for machine learning in data centers
Machine learning can learn from scenarios and data sets to formulate immediate reactions instead of requiring human intervention or relying on a limited set of preprogrammed actions. This technology can help you learn more about your data center's systems, manage them more efficiently and prevent unexpected downtime. Here are a few use cases where machine learning and AI can optimize data center performance.
1. Creating more efficient data centers
Companies can use machine learning to autonomously manage their data center's physical surroundings with real-time modifications done by the software to the physical facility and data center architecture instead of software alerts.
Google, Meta and Microsoft use AI and machine learning to monitor energy and performance optimization at their data centers.
2. Reducing risk in operations
Preventing downtime is crucial for data center operations. Machine learning can predict and prevent downtime. Data center machine learning software monitors real-time performance data from critical equipment -- such as power management and cooling systems -- and predicts when the hardware might fail. This enables operators to perform preventive maintenance on these systems and prevent costly outages.
Machine learning-based risk analysis improves data center uptime by modeling different configurations that increase resiliency; identifying opportunities for preventive maintenance; and identifying potential cybersecurity risks before they manifest.
3. Decreasing customer churn with smart data
Companies can use machine learning in data centers to understand their customers better and to predict consumer behavior. As an extension of customer success programs, machine learning can analyze the mountains of information in data centers that go unused after collection.
In connecting machine learning software with a customer relationship management (CRM) system, the AI-powered data center could search for and retrieve data stored in a historical database that is traditionally not used for CRM. This enables the CRM system to formulate different strategies that lead to generation or customer success.
4. Managing power and energy consumption
Organizations can manage energy with machine learning and immediately see significant gains. For example, Siemens' data center infrastructure management software, Datacenter Clarity LC, uses AI-powered tools to analyze individual servers to detect anomalies and opportunities for optimization.
Machine learning and AI tools discover and reroute workloads from less efficient servers to more energy- and work-efficient servers with lower utilization rates. Operators receive a notice to replace the older server. This notice gives operators time to upgrade the server before it becomes outdated.
5. Analyzing system logs
Most data center systems generate logs as they work, but they're not useful to operators if they are not managed. Machine learning can centralize and analyze the logs and create easy-to-use reports that are valuable to the data center's team. Open source technology, such as Elasticsearch and paid options from Splunk, can analyze and ingest data that machine learning routines gather.
6. Performing root cause analysis
When there is a performance error, operators must be able to quickly identify the root cause and fix it. AI predictive engines, like Hewlett Packard Enterprise's InfoSight, provide tools to identify and solve errors in near real time across an organization's on-premises data center and cloud setups.
AI and machine learning strategies to optimize data centers
Data center hardware and software are becoming more intricate as technology evolves. These demands affect IT staff and operations across the IT infrastructure.
AIOps and MLOps
AIOps and MLOps describe the use of AI and machine learning in IT operations to automatically identify and resolve IT operational issues. These approaches are multilayer platforms that apply machine learning, analytics and data science to IT operations at the enterprise level, whether that's on premises or in a data center.
AIOps breaks down data silos to aggregate and analyze IT data from service and operation management tools and applications. Identified patterns determine if it might handle the fix automatically or alert IT teams.
MLOps is the development of machine learning and its use to increase automation. MLOps also deploys, maintains and monitors machine learning models.
SecOps and DevOps
SecOps and DevOps are related terms that refer to AI and machine learning in cybersecurity and technology development activities. Previous automation tools didn't reduce the security analyst's workload; they increased it. SecOps with AI reduces errors and makes the analyst's job more efficient and holistic. Analysts must find what causes an alert, but the SecOps tools can identify the errors more proactively.
In DevOps, AI tools automate and optimize the software development and delivery process. AI automates testing and deployment processes, improves software development accuracy and quality as well as optimizes resource usage and asset allocation -- like cloud infrastructure. When code passes testing, it automatically integrates into the production codebase.
AI can suggest code to developers as they work, which reduces the time it takes to create new features or products. AI can also perform root cause analysis on errors in the code during development which enables DevOps teams to identify the error and take corrective action.
Julia Borgini is a freelance technical copywriter and content marketing strategist who helps B2B technology companies publish valuable content.