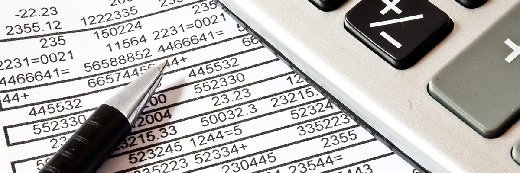
marrakeshh - Fotolia
Why consider an augmented data catalog?
Automated and augmented data catalogs have been around for a few years, but adoption is still lagging. Find out why an enterprise may consider investing in the technology.
Augmented data management is trending, and augmented data catalogs are a big part of that movement. The main difference between an augmented data catalog and a non-augmented one lies in improved automation, but this comes at a cost of more setup complexity.
Enterprises need to weigh these tradeoffs in choosing one or the other. They also need to consider how well different kinds of augmentation could fit into and enhance their data processing pipelines. Data-driven organizations could see big payoffs when augmented initiatives are done well.
A data catalog can help organize all the data within an organization. An augmented data catalog takes this one step further by automating various parts of the process, breaking the cycle of the one-off tasks. This is beneficial to businesses because it offers more flexibility and the obvious time-savings, said Jacek Żmudziński, senior marketing specialist at Future Processing, a software development consultancy.
Gartner estimated organizations that offer a curated catalog of internal and external data will realize twice the business value from their data and analytics investments. It also predicted 80% of data lake projects will fail to deliver value due to challenges in inventory and curating data through next year.
A big hurdle to this is the manual effort required to curate and manage the data catalogs. Gartner predicted 60% of data catalogs that do not use machine learning to assist in finding and inventorying data across a distributed environment will fail to be delivered on time.
Old wine in new bottles
Organizations have been creating metadata repositories since the 1980s -- called data dictionaries -- to characterize the technical properties of SQL databases. In the 1990s, these evolved into data repositories that extended the types of metadata collected to include useful definitions, operational characteristics and provenance information.
Over time, that gave rise to data catalogs through better tools for automating discovery and management.
The idea of augmented data catalogs suggests increasing the use of machine learning to automate more of this process. Helping data teams make sense of data sets that may be available to them is complex. Common steps involved in the data catalog process include:
- discover what data exists and how fields relate to each other;
- enrich and annotate data;
- organize and govern data; and
- make it easy for teams and applications to consume data.
An augmented data catalog suggests the notion of automating more, if not all, of these processes on an ongoing basis.
Ivan Kot, director of customer acquisition at Itransition, a software development consultancy, said an augmented data catalog can use machine learning to take on a range of manual tasks associated with data handling, ranging from consolidation and metadata discovery to curation and enrichment. This can significantly improve the accuracy and consistency of data management and better prepare data for further analysis.
Creating new context
The key differentiator for an augmented data catalog is that it can automate processes that would be otherwise impractical using traditional automation techniques.
For example, a non-augmented data catalog categorizes all technical details about an organization's data and processes used to produce and manage data. However, non-augmented data catalog tools usually only provide a singular view of data lineage by only documenting details about data on a physical level, said Emily Washington, executive vice president of product management at Infogix, a data governance platform.
Understanding technical data lineage helps the IT community identify the root cause of data quality issues and locate any data out of compliance. However, technical lineage can neglect some types of context that business users require. An augmented data catalog incorporates business lineage by utilizing machine learning technologies to automate the manual tasks involved in cataloging data.
By automating metadata and data lineage ingestion, an augmented data catalog can automatically profile data to discover patterns and descriptors needed to classify metadata. Automating lineage ingestion can then infer missing technical lineage and automatically generate relationships with different business glossaries.
This automation drastically reduces the time needed to curate a data catalog, while enabling users to visualize how data connects to business processes and operations.
"Ultimately, an augmented tool specifically helps with automating the creation of a catalog, while providing additional context for business users to understand the business details around enterprise data," Washington said.
Adding appropriate context
An augmented data catalog can also organize data sets to be more consumable by novices, said Florin Tufan, CEO at Soleadify, a search engine for business data. For example, a traditional data catalog is less structured and might list addresses in ways that make it easy to mail to people but hard to analyze.
Tufan said he has seen many databases with thousands of strings that look like "20735 Stevens Creek Blvd CA 95014 Cupertino." It may require an expert hours of work to tease these strings apart to perform a simple analysis, such as breaking down prospects by state.
"It's hard to do any sort of analysis or intelligence, because you'd need structure and predictability," he said.
In contrast, augmented data catalogs do a better job at maintaining a predictable taxonomy and structure across data sources.
Data catalogs also usually contain a high volume of redundant data points, which will make it much easier to manipulate and analyze the data. An augmented data catalog is more likely to include an address both as a complete string and broken down into state, city, county and street. An augmented data catalog also might automate the creation of confidence scores that make life easier for building machine learning models.
'A matter of time'
Washington noted many organizations have not upgraded due to cost constraints or because of the time it takes to retrain employees.
The biggest benefit to a non-augmented data catalog is they are simple to set up, especially for one-off projects.
"Non-augmented data sets are a straightforward choice for the short term," Tufan said.
But, he cautioned, that it's a choice most companies regret and correct as soon as more sophisticated use cases are needed.
"It's just a matter of time until non-augmented data sets need to be augmented, and the 'time' needed gets shorter and shorter with increased AI data adoption," Tufan said.