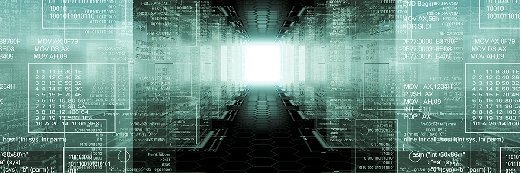
michelangelus - Fotolia
Why you should consider a machine learning data catalog
A machine learning data catalog can benefit an enterprise in a variety of ways, from increasing access to necessary data to keeping your data sources up to date.
The phrase "data is an asset" is something of a corporate cliché. However, it is increasingly true as companies in industry after industry undergo programs to digitize their businesses.
It is obvious that Netflix is a highly digital business with data at its heart, but what about more down-to-earth enterprises like manufacturing or energy? Even in the oil industry, the talk these days is of the digital oilfield where vast amounts of sensor data about the operation of an oil platform is captured and analyzed so field production can be tweaked and tuned in real time.
In order to extract value from your data, though, you first have to know what you have and where it is, and this seemingly obvious starting point is a major hurdle in a large corporation. A global company will have many separate applications with data on finance, sales, human resources, production, distribution, marketing and any number of industry-specific topics. Data about a subject like customers or product makes crop up in many systems, sometimes in different forms and not always consistently.
Ideally, there would be a place that listed all applications, what data is stored in which applications and how they all link together -- a sort of treasure map to your corporate data. A good analogy for this setup would be an index of books in a library: a place that documents what books are in the library, the author and publisher, where to find them and even who has checked out which books. However, building such a catalog of this labyrinth of data is a thorny and long-standing problem.
I first worked on a data catalog project in 1984 when working at Exxon, documenting applications and systems in what now seems like an ancient piece of software from IBM. Thirty-six years later, IBM is still in the data catalog business, and over the years many rival products have been developed to tackle the same issue. The market for data catalogs has remained a niche affair, with the entire market worth a trifling $200 million or so, depending on which analyst report you read.
The challenge with data catalogs
The key problem with data catalogs has remained the same as what I encountered in 1984 -- keeping the wretched thing up to date. As soon as you document the applications, who owns them and what data they have, new systems spring up around the company. Sometimes key data comes from third parties and is not in your control, and sometimes you gain hosts of new systems when your corporation acquires or merges with another.
Whoever is in charge of the data catalog has a thankless task. People running projects putting in new systems usually have no incentive to tell the data catalog team what they are doing, so it inevitably becomes out of date. When business users consult the data catalog and discover it does not have an up-to-date list of data assets, they start to distrust it and go elsewhere to find what they need. As this happens, the data catalog is used less, is updated less accurately and becomes even less trustworthy in a vicious cycle of neglect.
What is a machine learning data catalog?
Over the last few years, some vendors have adapted techniques to automate data catalogs with machine learning. Just as Google has tools that comb the web and index what is out there, a machine learning data catalog is hooked up to the numerous source systems that a corporation has.
A machine learning data catalog crawls and indexes data assets stored in corporate databases and big data files, ingesting technical metadata, business descriptions and more, and automatically catalogs them.
This process is ongoing rather than a one-off project. Provided the data catalog software is granted access to the various databases and files around the corporation, it can revisit metadata stored around the enterprise on a regular basis, just as Google finds newly created websites and indexes them.
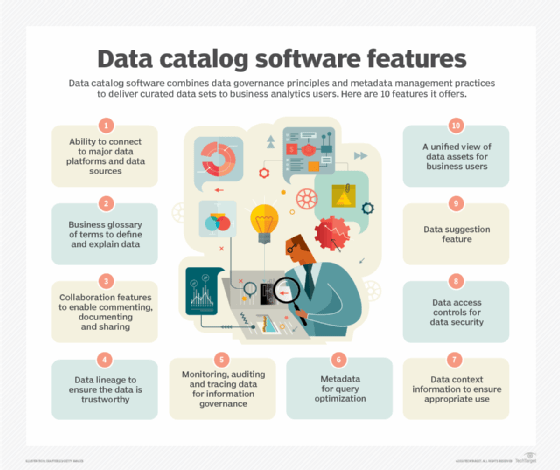
Benefits of a machine learning data catalog
The better machine learning data catalogs have pre-built connectors to the metadata of the major database vendors, making the indexing process reasonably efficient. They often have intuitive user interfaces, allowing business users to ask questions about corporate in natural language, rather as they might with a Google search -- e.g. "Where is sales revenue data for last year?"
This application of machine learning and automation holds out genuine hope that the biggest challenge of keeping data catalogs up to date is finally a problem that can be tackled.
Leading vendors
Vendors in the machine learning data catalog market include Alation, Unifi and Cambridge Semantics. There are also automated catalog capabilities from a number of other vendors including Infogix, Collibra, Informatica, Oracle and IBM.
Challenges of a machine learning data catalog
Vendors still have issues to contend with. Back in 1984, corporate data was all neatly tucked away in the corporate mainframes in a data center. Now, increasing amounts of key corporate data are being held in cloud databases or hybrid setups. Machine learning data catalog software has to keep track of corporate data in the cloud as well as data lakes, not just within the relatively well-understood corporate relational databases.
Nonetheless, the development of machine learning data catalogs has the potential to solve a problem that has persisted for decades, and to finally fulfill the promise that data catalogs have long promised.