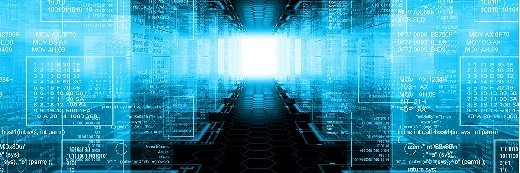
michelangelus - Fotolia
Data catalog management for analytics fraught with unique demands
Data catalogs for analytics applications demand detailed assessment of user needs, cross-functional teams, ready access, continuous improvement and a self-sustaining system.
Data cataloging techniques that help improve analytics workflows are undergoing notable changes as companies increasingly launch and expand more data science initiatives. And when it comes to data catalog management, the trick is to find an appropriate balance between organizational processes and the right tools.
"Data cataloging -- whether for reasons of agility or monetization or compliance reasons -- is an extremely important topic for most enterprises," said Shekhar Vemuri, CTO of IT consultancy Clairvoyant. When implementing data catalog management best practices, the key is to identify and acknowledge the organizational as well as technological issues.
Within a company, there's typically very little systemic knowledge of the location, origins and purpose of existing data, Vemuri added, resulting in longer-than-expected data cataloging projects. The problem is compounded by different representations and interpretations of data across business units. Confusion often ensues in consolidating data sets and building data catalogs based on different versions of a company's treasure trove of data.
Vemuri believes data catalog management should be two-dimensional: a broad and deep assessment of a company's data landscape as well as an iterative approach to continuous improvement of the process that allows for new discoveries of how business units might want to use the data. "There is no way to catalog an enterprise's data in a manual fashion," Vemuri noted.
When manual labor counts
By creating an all-encompassing catalog that allows for rapid scalability and efficient cataloging of data, it's possible to quickly scan large numbers of systems and repositories, deliver insights that feed into existing domain models and represent key entities companywide. The data catalog management team can then focus on manually consolidating and categorizing data that's similar but not the same.
"There won't be a perfect catalog ever," Vemuri said. Nonetheless, data catalogs can be highly effective, but that means an ongoing companywide effort that involves business users, data analysts and IT teams working together to prioritize data sets for consolidation and cataloging based on business needs and strategies.
In some cases, a master data management (MDM) program that harmonizes data about customers, products and other business entities is a prerequisite to data cataloging.
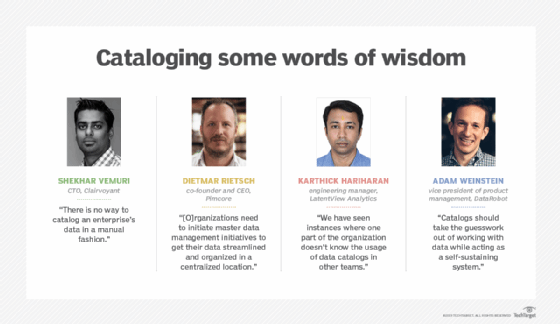
"In order to effectively deploy data catalogs, organizations need to initiate master data management initiatives to get their data streamlined and organized in a centralized location ... for insights moving forward," said Dietmar Rietsch, co-founder and CEO at product information management platform maker Pimcore GmbH.
Companies need to think about the underlying data models currently deployed and how they can be consistently defined. When that's done, Rietsch said, MDM can help drive data cataloging workflows and associated data governance programs.
Pimcore worked with a well-known U.S. food manufacturer that consolidated its product data management and reduced its heterogeneous software stack from about 15 content management systems to one homogeneous digital experience platform. This consolidation, Rietsch added, makes it easier for the entire marketing department to find and access relevant data and insights.
Cataloging for analytics apps
Many enterprises have adopted data cataloging to handle reporting, but quick experimentation used in data science applications requires a different approach, said Karthick Hariharan, engineering manager at consultancy LatentView Analytics. That's because traditional data cataloging doesn't readily accommodate the diverse use cases inherent in operational, real-time and advanced analytics.
Some of LatentView's enterprise clients have data distributed across different on-premises and cloud platforms. About 75% of data and metrics flowing into these systems are not cataloged nor up-to-date simply because the business logic and key performance indicators (KPIs) are embedded in different data pipelines built and deployed for different analytics use cases.
"It becomes very critical to mine all these details nested within a complex chain of SQLs and document it in such a way [that] it can be searched and discovered by analysts and business users," Hariharan explained. Better knowledge of KPIs, data lineage and granularity through the use of a data catalog can help analysts accelerate implementation without reinventing the wheel. That greatly helps consolidate the process and avoid redundant, obsolete and trivial computations.
Good governance and sustaining adoption
Change management is the biggest roadblock to instituting a governance program, Hariharan said, particularly an organization's attitude and approach to data. Since governance and best practices demand accountability and security, the significance of undergoing a change must be explained to every stakeholder.
One practice is to conduct workshops and identify "champions" who could mitigate any process issues proactively. It's also important to evaluate custom or proprietary tools and frameworks that the enterprise can roll out without disrupting business processes. Hariharan also recommended running periodic audits on knowns and unknowns about data, platforms and processes.
When rolling out new KPIs, launching new data platforms and introducing machine learning features, companies need to enforce data standards. Documentation and cataloguing must be consciously included as part of every deliverable, Hariharan said. ROI can be measured over time by closely tracking the time spent on data discovery for every project. It's also useful to identify and automate processes to proactively emit metadata to a central catalog.
"We have seen instances where one part of the organization doesn't know the usage of data catalogs in other teams," Hariharan said. Short-term experimentation and results-oriented thinking can sometimes compromise long-term planning.
Hariharan suggested open sources tools, such as Apache Atlas, to help lower the barriers to cataloging complex applications. Other relevant metadata implementations and tools to explore include Netflix's Metacat, Uber's Databook, Lyft's Amundsen, LinkedIn's WhereHows and Data Hub, and WeWork's Marquez.
Identify critical use cases
A common mistake in data catalog management is failure to address the diverse needs of various catalog users, said Adam Weinstein, vice president of product management at AI platform maker DataRobot. A compliance team, for example, may work on solving regulatory obligations by tracking lineage via a data catalog, but that may not satisfy the daily needs of business analysts who want to quickly query data for ad-hoc analytics. "As a result," Weinstein surmised, "different flavors of catalog are deployed that are tailored to each of these uses cases."
Standard catalog tooling can help, but Weinstein believes the highest-performing teams can use the same catalog for different processes. He recommended that work be done in online systems where code and insights can be searched and shared, which helps bring people with differing viewpoints about data together around a common problem.
In the end, the same set of data can be used for legal reporting, increasing productivity or improving the customer experience. "Catalogs," Weinstein said, "should take the guesswork out of working with data while acting as a self-sustaining system."