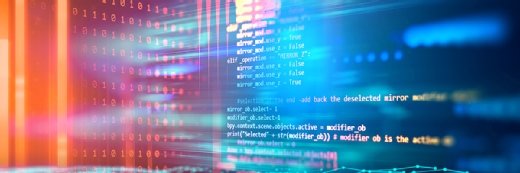
Getty Images/iStockphoto
Cleaning Up the EHR Master Patient Index to Boost Patient Matching
Integrating a simple spell-checking algorithm into the EHR could mitigate patient matching issues, especially during COVID-19.
EHR master patient index (MPI) data errors, such as typing and formatting errors, are a leading cause of patient matching issues and user search inconsistencies. Data standardization, “deep cleaning,” and making EHR searching similar to internet searching could reduce EHR and master patient index (MPI) errors, according to a recent article published in the Journal of AHIMA.
MPI data errors, such as typing and formatting errors, are a leading cause of patient matching issues and user search inconsistencies, according to James Hoover, co-chair of the health data integrity committee at California Health Information Management Association.
In 2019, approximately 18 percent of patient EHRs were considered duplicates. As a result, roughly one in five patients have incomplete health records.
Inconsistent patient matching creates a handful of problems for the patient and the provider. Although an error such as a misspelling or misheard word seems small, these errors could result in a duplicate record. During COVID-19, a duplicate record could mean a missing positive test result.
“Users must enter just the right amount of text—but not too much, or the record you are seeking will not be found because it does not match exactly,” explained Hoover.
“Enter too little, and too many results are returned. Wildcard matching (using a partial string ‘SMI’ to match a longer string ‘SMITH’ for example), a little finesse, and data in just the right fields might yield the patient records being sought if the user has the time, skill, and a bit of luck. EHRs assume (mostly) perfect data, and the responsibility to find the patient records is on the user,” Hoover continued.
Hoover said EHRs should identify typing errors and provide correct search results. This can be done by “deep cleaning.” Deep cleaning ensures the data inside search fields are not as typed but as the user intended. In other words, deep cleaning would ensure search results account for typos or spelling errors.
Hoover said a standard spell-checking EHR algorithm could flag typing errors that lead to a high number of patient matching issues.
Standardizing data elements, such as phone numbers and addresses, is critical to patient matching, and US Postal Service (USPS) formatting could be the solution, Hoover said. According to a 2019 study, USPS standardization could boost matching by up to 3 percent.
Next, Hoover pointed at the vast difference between an internet search engine and an EHR search engine. Google, Yahoo, and other internet search engines grasp context and categories, such as music or sports, while MPI searches are limited to name matching.
“With hundreds of billions of webpages, the scale of the web is five to six orders of magnitude above EHR search, and the search domain of EHR is limited strictly to patient demographics,” Hoover explained.
“Yet EHR search has much more difficulty finding patients with much less data, even when the subject of the search is present. The dichotomy of web versus EHR search performance is striking. Health information technology (IT) must improve.”
Hoover also noted how simple an internet search engine is to utilize, compared to the immense EHR training a clinician undergoes, mostly due to “fault tolerance” and the lack of one simple EHR search interface.
“Highly tunable, each field has distinct fault tolerance sensitivity,” wrote Hoover. “In practice, date of birth (DOB) should be less sensitive to variations than the last name field. Properly tuned, poorly spelled words can match given enough surrounding data.”
MPI data that can also identify nicknames and name misspellings, such as internet searches, would provide more flexibility to aid clinicians.
Due to the complexity of an EHR, Hoover acknowledged there would never be an error-free MPI. However, health IT developers can mitigate patient matching duplicates moving forward.
“Modern pattern matching techniques are better suited to the characteristics of MPI data,” Hoover said. “Pattern matching on text fragments rather than entire words works well for several classes of healthcare IT problems. There is an opportunity to improve health data quality at the same time that patient safety, satisfaction, and revenue cycle are improved.”