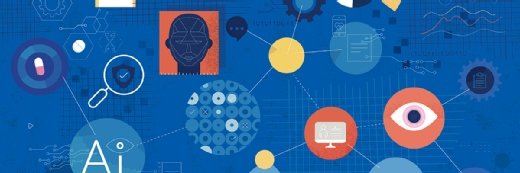
DrAfter123/DigitalVision Vectors
Retraining Improves Performance of ICU Predictive Analytics Model
The performance of an existing clinical decision support tool to predict readmission or death after discharge from the ICU improved following retraining and recalibration.
In a study published in Critical Care Medicine, researchers found that retraining and recalibration of a machine learning (ML)-based clinical decision support tool to predict readmission or death within seven days after discharge from the intensive care unit (ICU) significantly improved prediction performance, highlighting the importance of external validation and retraining before applying models in new settings.
According to the study, many ML models have been developed for application in the ICU, but relatively few of these have been subjected to external validation, making their performance unknown in settings outside those within which they were trained.
To help close this research gap, the researchers sought to assess the performance of an existing decision support tool based on an ML model predicting readmission or death within seven days after ICU discharge before, during, and after retraining and recalibration.
The Pacmed Critical model is certified in the European Union and designed to assist clinicians in determining the optimal moment to discharge patients from the ICU to a clinical ward. The model was developed and validated on EHR data collected between 2004 and 2021 from the Amsterdam UMC, a tertiary care center in The Netherlands. Upon internal validation, the model achieved an area under the receiver operating characteristic curve of 0.78.
To retrain the model, the researchers leveraged EHR data collected from 10,052 ICU admissions from 2011 to 2019 at the Leiden UMC, another tertiary care center in The Netherlands, with the same pipeline and modeling techniques as those used for the original model.
The research team assessed model performance using a temporal validation design four times: before retraining, after the first round of retraining, after the second round, and after the third and final round of retraining.
For the first round of retraining, the ML model was trained on data from 2011 to 2015. Data from 2011 to 2017 was used in the second round, and all Leiden UMC data from 2011 to 2019 was used for retaining in the final round.
From there, validation was performed on the 2018–19 Leiden UMC cohort.
Predictive performance was measured in terms of the area under the receiver operating characteristic curve (AUC) and calibration. Of the 10,052 discharged patients from the ICU at the validation site, 577 patients, or 5.7 percent, experienced readmission or death within seven days following ICU discharge.
Overall, the original model had an AUC of 0.72 when applied to the 2018-19 validation data cohort. The retrained models, however, showed improved discriminative performance, with an AUC of 0.79 for temporal validation rounds one, two, and three using the same validation data.
The researchers also found that initially, the calibration of the original model was poor, which contributed to a lack of generalizability despite similarities between patient populations, healthcare context, and model specification. However, retraining with a focus on disease severity monitoring and ICU specialty improved predictive performance.
These findings show that external validation and retraining are key steps of the ML development process that clinicians should consider before applying models to new settings, the research team concluded.
This is just one study evaluating the use of AI models and data analytics to improve ICU operations.
Last year, leadership from the University of Colorado Hospital shared how they deployed an advanced analytics tool to help detect when the hospital’s ICU was nearing maximum capacity, enabling them to move patients to less strained areas.