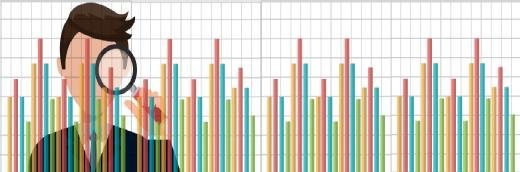
Fotolia
Collaborative analytics benefits enterprise data analysis
Sometimes, having more eyes on a problem can help solve it a little bit faster. Experts talk about the enterprise benefits of implementing collaborative analytics.
Data collaboration and collaborative analytics is still a newer concept. The idea is to bring community to analytics. The main benefits are that collaborative analytics can bring diverse expertise to the process and grow a shared understanding of results.
Making these programs work in practice requires striking a balance among new tools, people and processes for cultivating shared understanding. In some ways, this is simply an extension of trends around data integration.
What is collaborative analytics?
Collaborative analytics represents the usage side of data transformation, said Juan José López Murphy, technical director and data science practice lead at Globant, a digital transformation consultancy. This helps teams avoid misunderstandings that come from back-and-forth decisions between siloed areas to better align efforts and provide a coherent assessment of what's going on within the entire enterprise.
Most major business problems often cross domain, departmental and technology boundaries, which makes it hard to solve by only one kind of expertise, Murphy said. While companies try to have coordinated actions so they can grow and improve, they often miss having a coordinated understanding of what's going on. Collaborative analytics can bridge this gap.
How it helps data teams
"Collaborative analytics facilitates faster speed to insight," said Traci Gusher, partner of data, analytics and AI at KPMG.
It also has the potential to uncover a broader inventory of data that might be available with the variety of experiences different people have with sources of data. A broad perspective helps organizations stumble onto new ideas that might otherwise not surface.
"There is not one single data scientist who knows it all," said Rosaria Silipo, principal data scientist at Knime.
Problems that look unsolvable to a single data scientist can be broken apart, and the separate parts can be solved by a team.
There is also a kind of Ikea effect, where people who participate in building the analytics models are more likely to trust the results.
"Enterprises benefit from collaborative analytics by knowing that data users in the organization all contributed to creating, building and documenting the analytics," said Peggy Tsai, vice president of data solutions at BigID, a data intelligence platform.
What tools to use
A collaborative analytics implementation needs to include a common interface and workspace where users can access data and KPIs. This workspace must make data easily discoverable and accessible by providing a contextual business view of information in a familiar format for the business, explained Radhakrishnan Rajagopalan, senior vice president and global head of customer success, data and intelligence at Mindtree, a technology consulting and digital transformation company.
It's useful to compare it to a marketplace that facilitates the creation and sharing of data products.
A collaborative workspace must enable transparent data manipulation. More importantly, it should enable data visualization so users can analyze different facets of the information. Once the data is analyzed, data teams should have the ability to create other versions and share with colleagues for collaboration, review and usage.
Collaborative analytics in practice
Quotient Technology, which develops tools for analyzing consumer spend across channels, developed a collaborative analytics platform to coordinate sales, customer success, and analytics and insight teams.
"To drive collaboration, teams must have shared measurable incentives around something key to the business, such as client satisfaction, revenue growth or other performance metrics," said Blake Burrus, senior vice president of analytics at Quotient Technology.
This ensures each team gets utility that drives adoption and usage, while also creating the incentive for collaboration around a shared goal.
Teams may work in different applications that are integrated on the back end to help drive them toward a shared goal. Their shared KPI lies in improving performance as measured by return on marketing spend.
"We're all committed to providing winning outcomes for our clients and everyone's workflows to that goal," Burrus said.
The human element
The human element is one of the most important aspects of getting a collaborative analytics program to work in practice. Collaborative analytics requires cultivating data literacy, openness and a willingness to learn, Murphy said.
Data literacy helps participants make a distinction among what the data says, what it inspires people to believe and the hypothesis they need to corroborate before making a decision. Building openness is the process of fostering a sense of humility that helps realize the value each member can bring to the table in terms of experience, domain knowledge, technical approaches and perspectives.
"Oftentimes, particularly in IT contexts, we see the idea of 'technical' people conflated with programmers or numerical analysts of some kind, without realizing that every department and perspective has their technical background and approach," Murphy said.
It is also crucial to foster a willingness to learn that encourages people to share dissenting opinions.
"As humans, we sometimes hesitate to put forward ideas as a member of a team that might be too risky or far-fetched," Gusher said.
People also allow their perspectives to be swayed or adjusted based on the group they work with. Teams that fundamentally have different backgrounds, skills and perspectives on problems can ultimately create more holistic insights and solutions.
Gusher has found it useful to adopt structure, such as consistent methods for approaching data analysis, tools that support effective collaboration and templated team roles that represent different perspectives. This can include business or domain knowledge, analytical approach depth, and data acquisition and engineering skills.
"Team members need roles and defined purposes, or else these teams become the Wild West and start stepping on each other without generating the creative value that these teams are intended to drive," Gusher said.
The AI element
Once the basic tools, people and processes are in place, AI can help drive insight, vet data and suggest next steps.
"AI works mostly behind the scenes by enriching the content and reach of the available information," Murphy said.
One way AI can help collaborative analytics is suggesting pieces of information that could be relevant or should be considered on a given topic using a recommender of sorts but focusing on data sources and particular slices or visualizations.
AI can also fill in the gaps of data that teams would like to have but can't collect until it's too late or too costly. AI can also help build what-if scenarios and play out different strategies and outcomes to make the best decisions by combining the knowledge of the entire team involved as best as possible.