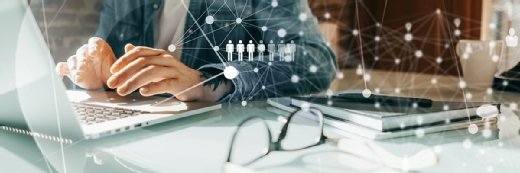
Rymden - stock.adobe.com
How to analyze data in 7 steps for better business decisions
There's no such thing as too much data. Learn how to analyze that data for insights that can give your business a boost.
Information inundates organizations -- information that some often ignore to the detriment of a business. But data-driven companies understand the importance of gathering and analyzing that data.
The goal of data analysis is to give businesses actionable insights from the large amounts of data they receive every day. These insights can help organizations make decisions, solve problems, understand the needs of customers and identify future trends.
Companies can use business intelligence tools to integrate different data sources, perform advanced data analysis, build interactive business dashboards, generate actionable insights and streamline decision-making.
Learn how to get the most out of your data with BI tools.
Why is data analysis important?
Data analysis is a subset of data analytics. Analysis involves gathering, cleaning, analyzing and interpreting massive amounts data to find patterns and other insights. It also includes the organization, tools and techniques used for in-depth analysis -- including the tools to communicate the results, such as data visualization tools.
Data analysis provides insights that tell companies where to focus their efforts. These insights can help businesses improve products and services, customer experience and overall brand image. Analyzing data offers companies evidence to support business decisions and deliver better customer support.
What are the different types of data analysis?
There are several data analysis methods organizations can use to uncover actionable insights. The main data analysis techniques include:
- Text analytics. Also known as text mining, text analysis uses machine learning and natural language processing to organize and extract insights from unstructured text data. Text analysis interprets trends and patterns to offer in-depth and targeted views about why something is happening or might have happened.
- Statistical analysis. Statistical analysis involves data collection, analysis, modeling, interpretation and presentation using dashboards. It answers the question, "What happened?" Statistical analysis can be broken down into two subcategories: descriptive analysis and inferential analysis. Descriptive analysis works with complete or selections of summarized numerical data. It doesn't try to make predictions about future trends. Instead, it draws insights from past data by manipulating it in ways that make it more meaningful. Inferential analysis analyzes samples derived from complete data. In this type of analysis, an analyst can draw different conclusions from the same data set by selecting different samples.
- Diagnostic analysis. Diagnostic analysis uses insights gleaned from statistical analysis to identify patterns in data. It answers the question, "Why did this happen?" This type of analysis enables analysts to use patterns uncovered in older data to solve current challenges.
- Predictive analysis. By using patterns discovered in data from the past as well as current events, analysts can predict future events. It answers the question, "What's most likely to happen?"
Read about how machine learning and predictive analytics work better together.
- Prescriptive analysis. This is the most advanced form of analysis. Prescriptive analysis combines all of a company's data and analytics to offer the best course of action. It analyzes many scenarios, predicts the outcome of each and decides which action a company should take based on the findings.
How to analyze data
To get the most of their data, organizations need to implement a data analysis process. This process includes:
- establishing goals
- collecting, cleaning and analyzing data
- visualizing data in dashboards
Here are seven steps organizations should follow to analyze their data:
- Define goals. Defining clear goals will help businesses determine the type of data to collect and analyze.
- Integrate tools for data analysis. These tools can be integrated via APIs and one-click integrations. However, organizations may need a developer's help to set up the API.
- Collect the data. Organizations need to gather all the data into one location so it can be analyzed. Quantitative and qualitative data can both be stored in Excel. Businesses also use APIs and integrations to connect their data sources directly to data analysis tools.
- Clean the data. To get accurate results companies must clean their data before analyzing it. Remove things such as punctuation, special characters, HTML tags and duplicate records.
- Analyze the data. Organizations should use data analysis software and other tools to help understand the data. Some data analysis tools include Rapid Miner, Chartio, Metabase, Redash, Excel, Python, R, Looker and Microsoft Power BI.
- Draw conclusions. Businesses should do a deep dive into the data to gain actionable insights. Then interpret those results to determine the best courses of action.
- Visualize the data. Organizations should present the information in a way that others can read and understand. And there are many data visualization techniques. Organizations can use dashboards to aggregate the data, making it easy to identify trends and patterns. Many analysis tools have in-built dashboards. Businesses can also use graphs, maps, charts, bullet points and other methods to present the information. Visualizing the data helps companies derive insights by enabling them to compare data sets and observe relationships.
Benefits to data analysis
Organizations can reap several benefits from analyzing data, including:
- Improved websites. Businesses can gather data about customers by analyzing their interactions with their websites. Organizations can then use this information to improve website performance, enhancing the customer experience.
- Customized shopping experience. Businesses might suggest products that customers are likely to buy based on their past purchases. This is possible by uncovering sales trends based on customer preferences, online shopping experiences and other patterns.
- Customer retention. Data analysis enables sales managers to identify the best types of customers. Determining which customers are not as profitable as others enables organizations to decide the best promotional strategies or whether to let customers go.
- Competitor research. Data analysis helps companies research the competition. It can provide insights about competitors' strengths, weaknesses, marketing strategies and sales tactics. Organizations can also analyze their competitors' negative reviews to decide how to outperform them.
- Improved employee performance. Monitoring employee performance enables HR departments to understand how workers spend their time and identify ways to improve productivity.
- Improved security. Monitoring data can help financial institutions and other companies that process online transactions improve security. For example, credit card companies can use insights to help eliminate fraud. By monitoring consumer behavior, businesses can detect suspicious transactions, flag accounts, and catch and/or prevent fraud.
- Improved operational efficiency. Collecting and analyzing supply chain data can show the source of production delays or bottlenecks. It can also help organizations predict where future problems may occur.
- Improved inventory management. Businesses can make better decisions about how much inventory is required to meet demand and keep their stock levels to a minimum.