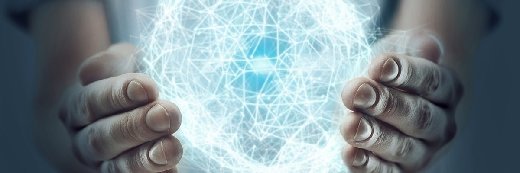
peshkova - Fotolia
How predictive and prescriptive analytics impact the bottom line
With all the data organizations collect now, they need a good way to analyze it, and that's where predictive and prescriptive analytics can be useful.
In a digitally transformed world, the combination of data and analytics is critical to maintaining a competitive advantage and business relevance. To achieve this goal, enterprises collect vast volumes of data and derive valuable insights from them. This knowledge can be anything from ascertaining customer satisfaction to identifying operational discrepancies.
The capability of business intelligence and analytics is continually evolving. In a highly competitive business world, analytics plays a key role in identifying trends and patterns to make quick and informed business decisions. Predictive and prescriptive analytics are two important methods in business-analytics solutions. Mordor Intelligence research suggests that the predictive and prescriptive analytics market (valued at $8.14 billion in 2019) is expected to grow at a compound annual rate of 22.53% to reach $27.57 billion by 2025.
As artificial intelligence (AI) and machine learning evolve and play a more significant role in data and analytics, smart algorithms can now pull both prescriptive and predictive insights from the data. Both approaches give insight and foresight to enable smart decision-making; they incorporate data mining, machine learning and statistical modeling to deliver deep insight into customers and overall operations.
Predictive and prescriptive analytics: A refresher
Business managers use predictive analytics, as the name suggests, to predict the future by analyzing and identifying patterns in historical data with ML and statistical models. The insights derived from this data are then used to make better decisions and improve outcomes. The keystones of predictive analytics are predictive modeling, decision analysis and optimization and transactional profiling. This approach exploits patterns in historical and transactional data to identify opportunities and associated risks.
Predictive analytics leverages AI and machine learning algorithms to build predictive models. These models are then used to analyze data sets to identify underlying patterns to anticipate future outcomes. For example, banks analyze mortgage applicants' data, including their credit score, employment status, income and savings-to-debt ratio to predict if they would be low- or high-risk borrowers. These "predictions" also determine the size of the loans and the financial institution's interest rate. Furthermore, AI and machine learning algorithms are also used to identify patterns that possibly indicate fraud.
On the other hand, prescriptive analytics is often described as the final phase of business analytics because it helps tweak predictive analytical models that predict what will happen if companies maintain their current course of action. It's a type of data analytics that factors data about available resources, possible situations, past performance, current performance and more to suggest a course of action or strategy.
Prescriptive analytics goes beyond predicting options to suggest a range of prescribed actions and the potential consequences of each action. It can also recommend the best course of action for each specified outcome. A self-driving car, for example, drives on its own by making millions of calculations during each trip. That's prescriptive analytics in action.
Unlike predictive analytics, prescriptive analytics is an abstract form of data analytics that helps companies explore "what if" scenarios and infer outcomes based on multiple variables. For example, airlines leverage prescriptive analytics to set airline ticket prices based on several possible factors.
While relatively complex to administer, prescriptive analytics is worth the effort, as it can have a significant impact on your bottom line. Whether it's to boost production, streamline operations or optimize customer experiences, prescriptive analytics helps organizations make "smart" decisions.
Business perspective: The pros and cons
Both predictive and prescriptive analytics are advanced forms of analytics that have a role to play in business intelligence. When the two are combined with machine learning, data mining and modeling, a company can make game-changing decisions. Both are equally and independently important to achieve better business outcomes.
Predictive analytics is applied to a wide range of business strategies and continues to play a vital role in advertising and marketing recommendations engines. These insights provide business executives and managers with the decision-making tools they need to achieve different goals like manufacturing optimization, revenue forecasting, cross-selling and up-selling opportunities.
The primary disadvantage here: Data-driven decision-making demands a significant amount of (relevant) data covering a wide range of activities. All this information isn't always easy to come by. Furthermore, when anticipating human behavior, it's difficult for smart algorithms to consider variables that aren't straightforward. For example, think about the impact the weather has on people's moods and how it influences customer purchasing behavior.
As humans evolve, so should these predictive models to help better anticipate human behavior. When companies fail to update their models, it opens the door to events like the subprime mortgage crisis of 2008. In that scenario, invalid models predicted the likelihood of mortgage customers repaying loans without considering the possibility that housing prices could fall.
Meanwhile, prescriptive analytics eliminates the immediate uncertainty that comes with changing conditions. That's why it's utilized to increase efficiency, improve productivity, mitigate risk and enhance customer loyalty.
The key disadvantage here: Prescriptive analytics isn't foolproof. However, it's a huge benefit if one knows what questions to ask and the best way to react to the answers. It's also critical to note that invalid input assumptions lead to inaccurate output results.
When used correctly, organizations are better placed to make highly analyzed, well-informed decisions that have the highest impact. This is because prescriptive analytics can simulate the probability of different predefined outcomes, reducing uncertainty and risk. This approach also helps companies understand worst-case scenarios and prepare for them accordingly.
The right skills and knowledge
With the right skills and knowledge, businesses can take the following steps to develop and deploy the right business analytics strategy.
- Clearly define the problem in a manner that's easily quantified. It will all depend on specific goals, and the problem can have multiple possible scenarios and problem statements.
- Optimize the data collection capabilities. This requires the right infrastructure to collect data from multiple sources, to clean it and to store it in a centralized, secure location. Engineers might be tasked with building systems that leverage databases like Apache Cassandra or MongoDB to aggregate statistical data.
- Identify what kind of data will be collected to get actionable insights. This is essential to ensure the wrong kind of information is not being collected.
- Collect data from multiple sources. This process should be automated to quickly collect large amounts of information with little to no human intervention.
- Analyze the data. Implement both predictive and prescriptive analytics protocols to derive the most value from the data. Viewing the data from multiple perspectives helps eliminate biases.
- Extract recommendations or answers to questions based on the analysis.
Predictive analytics will help a business understand where it's headed. When prescriptive analytics is added to the mix, a business can take insights to the next level by transforming informed processes and automating suggested actions. Properly implemented, both help businesses make better decisions that improve performance while optimizing resources to the core.
What's the best data analytics approach for an organization? There's no right answer to this question, as it's relative to each business model and each business's short- and long-term goals. Depending on the specific business needs, a business can use these methods individually or together to achieve its objectives.
About the Author
Jaya Shankar Byrraju is an IT professional with over 12 years of professional experience. He has a Bachelor of Science degree in mathematics, electronics and computer science, and a master's degree in management information systems, specializing in the design, development and use of enterprise analytic platforms. He has held diverse roles from architecture, strategy and governance, to technical design, development and administration in a number of organizations.