Three key ingredients of any data lake implementation
A data lake implementation can provide enormous flexibility for analyzing all types of external and internal data -- but not without these three requirements.
GRAPEVINE, Texas -- Like any other tool or technology, the data lake -- a storage repository and processing engine -- has its pros and cons. One of its oft-touted benefits is that it can ingest data without compromising the data format, providing data scientists with greater flexibility in the kinds of questions they can ask.
"Think of the data lake as your question development environment: You don't know what you don't know," said Nick Heudecker, a Gartner analyst. The data lake allows you to discover what you don't know, as one question suggests another.
A drawback? Without the proper skills, integration and data governance, a data lake implementation can quickly turn into a data management nightmare. Heudecker held up those three characteristics as the keys to a healthy data lake during his session on the technology at the recent Gartner Business Intelligence and Analytics Summit.
Skills
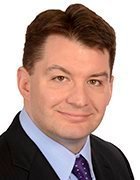
Data scientists are a requisite ingredient for any data lake inquiry. "They have reasonably good domain understanding, lower IT skills, but you're hiring them for their quantitative skills," Heudecker said.
But data scientists aren't the only skill set needed for a data lake implementation. Heudecker also pointed to:
- Data engineers, who operationalize the findings of the data scientist and work closely with the business;
- Business experts, who provide context;
- Software engineers, who focus on the nuts and bolts of the data lake implementation; and
- Citizen data scientists, while not required, who can act as a "force multiplier for the data scientist," even though they aren't skilled enough to take on the role completely.
"Data science is a team sport," Heudecker said. "If you want a successful data lake, you'll have to surround it with a successful team."
Integration
IT departments need to consider how to pull data -- from internal and, increasingly, external sources -- into the data lake, which will mean integrating the lake with the rest of the IT infrastructure.
Doing so requires that the initial cataloguing and indexing of the data as well as data security be done right, Heudecker said. Additionally, CIOs will also have to consider where insights will be consumed. Some data lake technologies -- such as Hadoop -- may not "support high levels of concurrency and multi-tenancy," Heudecker said. "They may not work very well against your chosen [business intelligence] platform or dashboard tool."
Consumption of the analysis could happen outside of the data lake in something like a MySQL, SQL Server or MongoDB database, according to Heudecker.
Data governance and data quality
Data governance and data quality are keys to ensuring the right discoveries are made, but the standards and application of them are more nuanced than in a traditional environment. Too much can hamper the kind of discovery the data lake concept was built for; too little can mean serious trouble for the organization.
To draw the fine line, Heudecker recommended IT departments think about data cardinality, or how the data relates to other data, and data lineage, or "what you've done with the data, where it came from, who changed it and why," he said. "I think you can forgo other elements of governance, at least while you're in the data lake environment."
Heudecker called data quality "a major challenge" in the data lake. He said IT departments should create catalogues and "socialize" data sets as a way of communicating from one employee to the next their relative data quality and what they can be used for.
Before diving into a data lake implementation, IT departments should consider what outcomes the business is after, how the data lake will help achieve those outcomes and if the necessary skills exist to get them there.
"You don't have to invest millions of dollars into this infrastructure. You can start in the cloud, you can start with tools that are readily and freely available, and if you don't have a data science team today, you can start to craft that team in conjunction with your data lake implementation," Heudecker said.