Data lake governance: Benefits, challenges and getting started
A data lake that isn't well governed might become more of a swamp. Explore the key benefits and challenges of data governance in a data lake, plus initial steps to take.
A successful data governance program applies policies, standards and processes to create high-quality data and ensure that it's used appropriately across an organization. Data governance initially focused on structured data in relational databases and traditional data warehouses, but things have changed. If your organization has a data lake environment and wants to get accurate analytics results from it, you also need to engage in proper data lake governance as part of your overall governance initiative.
But data lakes pose various challenges across all the disciplines of enterprise data management, including data governance. Some top governance benefits are described below, along with the challenges of effectively governing data lakes.
What is a data lake?
A data lake is a data platform that holds vast amounts of raw data, often including a variety of structured, unstructured and semistructured data types. It's usually built on Hadoop, Spark and other big data technologies.
While most data warehouses store data in relational tables, a data lake uses a flat architecture. Each data element is assigned a unique identifier and tagged with a set of metadata tags. As a result, a data lake is less structured than a data warehouse. Data typically is left in its native format and classified, organized and filtered as needed for specific analytics uses, not when it's loaded into the data lake.
Data lake vs. data swamp
If a data lake isn't well managed and governed, it can become more of a swamp than a lake. Data is dumped into the platform without suitable oversight and documentation, making it difficult for data management and governance teams to keep track of what's in the data lake. That can cause problems with data quality, consistency, reliability and accessibility.
As a result, data scientists, data engineers and other end users might not be able to find relevant data for analytics applications. Even worse, a data swamp might lead to analytics errors and, ultimately, bad business decisions. Data security and privacy protections might not be applied properly, putting an organization's data assets -- and its business reputation -- at risk. To avoid such a swampy situation, governing a data lake environment is a must.
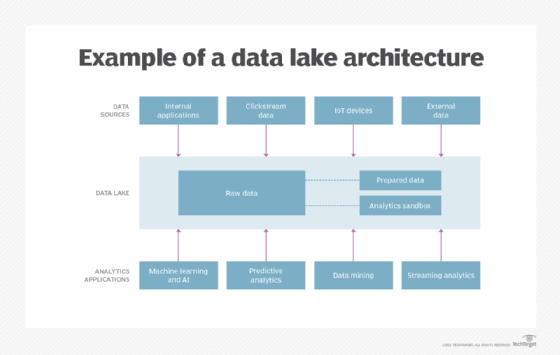
Benefits of data lake governance
Effective data governance enables organizations to improve data quality and maximize the use of data for business decision-making, which can lead to operational improvements, stronger business strategies and better financial performance. That applies to governing data lakes as it does with other types of systems. Some of the specific benefits that data lake governance provides include the following:
- Increased access to relevant data for advanced analytics. In a well-governed data lake, it's easier for data scientists and other members of analytics teams to find the data they need for machine learning, predictive analytics and other data science applications.
- Less time spent preparing data for analytics uses. While the data in a data lake commonly is left in its raw form until it's needed for specific applications, the data preparation process can be shortened in a governed environment. For example, upfront data cleansing reduces the need to fix data errors and other issues later on.
- Lower IT and data management costs. By preventing a data lake from sprawling out of control, the data processing and storage resources it requires can be reduced. Overall data management needs can also be decreased by improving data accuracy, cleanliness and consistency.
- Improved security and regulatory compliance on sensitive data. Customer analytics to aid in marketing and sales is a common use case for data lakes. As a result, they usually include sensitive information about customers. Strong governance of a data lake helps ensure that such data is properly secured and doesn't get misused.
Data lake governance challenges
Companion data management disciplines to data governance include data quality, metadata management and data security, all of which factor into data lake governance. Here are some common data governance challenges encountered in a data lake implementation.
- Identification and maintenance of the correct data sources. In many data lake implementations, the source metadata isn't captured or isn't available at all, making the validity of the data lake's contents questionable. For example, the system of record or the business owner of data sets might not be listed, or obviously redundant data might be causing issues for data analysts. At a minimum, the source metadata for all the data in a data lake should be recorded and made available to users to provide insight into its provenance.
- Metadata management issues. Metadata gives context to the content of data sets and is an important component in making data understandable and usable in applications. But many data lake implementations ignore the need to apply the correct data definitions to the collected data. Also, since raw data is often loaded into a data lake, many organizations don't include the steps needed to validate the data or apply organizational data standards to it. This lack of proper metadata management makes the data in a data lake less useful for analytics.
- Lack of coordination on data governance and data quality. Not coordinating data lake governance and data quality work can result in poor-quality data getting into a data lake. That can lead to inaccurate results when the data is used for analytics and to drive business decisions, causing a loss of confidence in the data lake and a general distrust of data across an organization. Effective data lake implementations involve data quality analysts and engineers working closely with the data governance team and business data stewards to apply data quality policies, profile data and take necessary actions to improve its quality.
- Lack of coordination on data governance and data security. In this case, data security standards and policies that aren't applied properly as part of the governance process can cause issues with access to personal data protected by privacy regulations and other types of sensitive data. Although data lakes are intended to be a rather open source of data, there's a need for security and access control measures, and the data governance and data security teams should work together during the data lake design and loading processes and ongoing data governance efforts.
- Conflict among business units that use the same data lake. Different departments might have different business rules for similar data, which can result in an inability to reconcile data differences for accurate analytics. Having a robust data governance program with an enterprise view of data policies, standards, procedures and definitions, including an enterprise business glossary, can reduce the issues that arise when multiple business units use one data lake. If an organization has multiple data lakes, each one should be included in the data lake governance process and have business data stewards assigned to it.
How to get started on governing a data lake
As with governing data in other types of systems, some common first steps to take on data lake governance include the following:
- Document the business case for governing a data lake, including data quality metrics and other ways to measure the benefits of the governance work.
- Find an executive or business sponsor to help get approval and funding for the governance effort.
- If you don't already have a data governance structure in place, create one that includes a governance team, data stewards and a data governance committee made up of business executives and other involved data owners.
- Work with the governance committee to develop data standards and governance policies for the data lake environment.
Another good initial step is building a data catalog to help end users locate and understand the data stored in a data lake. Or, if you already have a catalog for other data assets, it could be extended to include the data lake. A data catalog captures metadata and creates an inventory of available data that users can search to find what they need. You can also embed information about your organization's data governance policy in a catalog, along with mechanisms to enforce rules and restrictions.
The value of a data lake can be enhanced significantly by including strong data governance combined with metadata management, data quality and data security processes in the design, loading and maintenance of the environment. Active participation by experienced professionals in all of those areas is also crucial. Otherwise, your data lake might indeed become more of a data swamp.
Editor's note: This article was updated in June 2024 to provide a better reader experience.
Anne Marie Smith, Ph.D., is an information management professional and consultant with broad experience across industries.