What is data governance and why does it matter?
Data governance is the process of managing the availability, usability, integrity and security of the data in enterprise systems, based on internal standards and policies that also control data usage. Effective data governance ensures that data is consistent and trustworthy and doesn't get misused. It's increasingly critical as organizations face expanding data privacy regulations and rely more and more on data analytics to help optimize operations and drive business decision-making.
A well-designed data governance program typically includes various roles with different responsibilities: a senior executive who oversees the program, a governance team that manages it, a steering committee or council that acts as the governing body, and a group of data stewards. They work together to create the standards and policies for governing data, as well as implementation and enforcement procedures that are primarily carried out by the data stewards. Ideally, executives and other representatives from an organization's business operations take part, in addition to the IT and data management teams.
Data governance is a core component of an overall data management strategy. But organizations need to focus on the expected business benefits of a governance program for it to be successful, starting in the early days of an initiative, independent consultant Nicola Askham wrote in a September 2023 blog post. Eric Hirschhorn, chief data officer at The Bank of New York Mellon Corp., made the same point in a session during the 2022 Enterprise Data World Digital conference. "Outcomes can't just be good governance," he said. "Outcomes have to be running better businesses."
This comprehensive guide to data governance further explains what it is, how it works, the business benefits it provides, best practices and the challenges of governing data. You'll also find an overview of data governance software and related technologies that can aid in the governance process. Throughout the guide, hyperlinks point to related articles that cover the topics being addressed in more depth.
Why data governance matters
Without effective data governance, data inconsistencies in different systems across an organization might not get resolved. For example, customer names are sometimes listed differently in sales, logistics and customer service systems. If that isn't addressed, it could complicate data integration efforts, causing operational problems in those departments, and create data integrity issues that affect the accuracy of business intelligence (BI), enterprise reporting and data science applications. In addition, data errors might not be identified and fixed, further affecting analytics accuracy.
Poor data governance can also hamper regulatory compliance initiatives. That could cause problems for companies that need to comply with the increasing number of data privacy and protection laws, such as the European Union's GDPR and the California Consumer Privacy Act (CCPA). An enterprise data governance program typically includes the development of common data definitions and standard data formats that are applied in all business systems, boosting data consistency both for business uses and to help meet regulatory requirements.
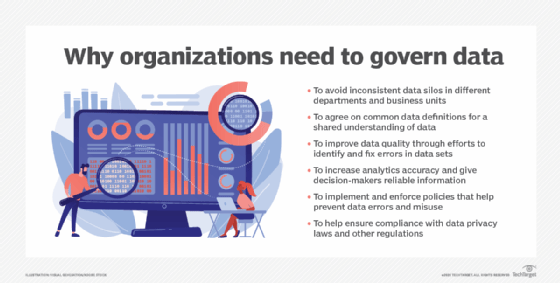
Data governance goals and benefits
A key goal of data governance is to break down data silos in an organization. Such silos commonly build up when individual business units deploy separate transaction processing systems without centralized coordination or an enterprise data architecture. Data governance aims to harmonize the data in those systems through a collaborative process, with stakeholders from the various business units participating.
Another data governance goal is to ensure that data is used properly, both to avoid introducing data errors into systems and to block potential misuse of personal data about customers and other sensitive information. That can be accomplished by creating uniform policies on the use of data, along with procedures to monitor usage and enforce the policies on an ongoing basis. In addition, data governance can help to strike a balance between data collection practices and privacy mandates.
Besides more accurate analytics and stronger regulatory compliance, the benefits that data governance provides include the following:
- Improved data quality.
- Lower data management costs.
- Increased access to needed data for data scientists, other analysts and business users.
- More-informed business decisions based on better data.
- Ideally, competitive advantages and increased revenue and profits.
Who's responsible for data governance?
In most organizations, various people are involved in the data governance process. That includes business executives, data management professionals and IT staffers, as well as end users who are familiar with relevant data domains in an organization's systems. These are the key participants and their primary governance responsibilities.
Chief data officer. The chief data officer (CDO) -- if there is one -- is often the senior executive who oversees a data governance program and has high-level responsibility for its success or failure. The CDO's role includes securing approval, funding and staffing for the program; playing a lead role in setting it up; monitoring its progress; and acting as an advocate for it internally. If an organization doesn't have a CDO, another C-suite executive will usually serve as an executive sponsor and handle the same functions.
Data governance manager and team. In some cases, the CDO or an equivalent executive -- the director of enterprise data management, for example -- might also be the hands-on data governance program manager. In others, organizations appoint a data governance manager or lead specifically to run the program. Either way, the program manager typically heads a data governance team that works on the program full time. Sometimes more formally known as the data governance office, it coordinates the process, leads meetings and training sessions, tracks metrics, manages internal communications and carries out other management tasks.
Data governance committee/council. The governance team usually doesn't make policy or standards decisions, though. That's the responsibility of the data governance committee or council, which is primarily made up of business executives and other data owners. The committee approves the foundational data governance policy along with associated policies and rules on things like data access and usage, plus the procedures for implementing them. It also resolves disputes, such as disagreements between different business units over data definitions and formats.
Data stewards. The responsibilities of data stewards include overseeing data sets to keep them in order. They're also in charge of ensuring that the policies and rules approved by the data governance committee are implemented and that end users comply with them. Workers with knowledge of particular data assets and domains are generally appointed to handle the data stewardship role. That's a full-time job in some companies and a part-time position in others. There can also be a mix of IT and business data stewards.
Data architects, data modelers and data quality analysts and engineers are usually part of the governance process, too. In addition, business users and analytics teams must be trained on data governance policies and data standards to help prevent them from using data in erroneous or improper ways.
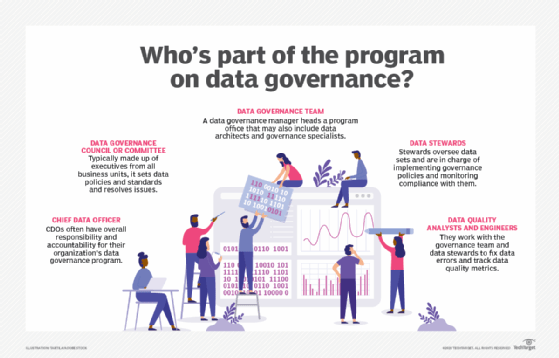
Components of a data governance framework
A data governance framework consists of the policies, rules, processes, organizational structures and technologies that are put in place as part of a governance program. It also spells out things such as a mission statement for the program, its goals and how its success will be measured. Decision-making responsibilities and accountability for the various functions that will be part of the program are specified in the framework, too. An organization's governance framework should be documented and shared internally, so it's clear to everyone involved -- upfront -- how the program will work.
On the technology side, data governance software can be used to automate aspects of managing a governance program. While data governance tools aren't a mandatory framework component, they support key functions in the governance process, including the following:
- Program and workflow management.
- Collaboration.
- Development of governance policies.
- Process documentation.
- Data mapping and classification.
- Creation of data catalogs and business glossaries.
The software can also be used in conjunction with data quality, metadata management and master data management (MDM) tools to aid governance efforts.
Data governance implementation
Data governance should be a strategic initiative for organizations. The steps to take in creating a data governance strategy include the following to-do items as starting points:
- Identify data assets and existing informal governance processes.
- Increase the data literacy and skills of end users.
- Decide how to measure the success of the governance program.
Before implementing a data governance framework, another required initial step is identifying the owners or custodians of different data assets across an enterprise and getting them -- or designated surrogates -- involved in the governance program. The CDO, executive sponsor or dedicated data governance manager then takes the lead in creating the program's structure. This includes working to staff the data governance team, identify data stewards and formalize the governance committee.
Once the structure is in place, the real work of governing data begins. The data governance policies and data standards must be developed, along with rules that define how data can be used by authorized personnel. In addition, a set of controls and audit procedures are needed to ensure ongoing compliance with internal policies and external regulations and to guarantee that data is used in a consistent way across applications. The governance team should also document where data comes from, where it's stored and how it's protected from misuse and security attacks.
As mentioned in the previous section, data governance initiatives usually also include the following elements:
- Data mapping and classification. Mapping the data in systems helps document data assets and how data flows through an organization. Different data sets can then be classified based on factors such as whether they contain personal information or other sensitive data. The classifications influence how data governance policies are applied to individual data sets.
- Business glossary. A business glossary contains definitions of business terms and concepts used in an organization -- for example, what constitutes an active customer. By helping to establish a common vocabulary for business data, business glossaries can aid governance efforts.
- Data catalog. Data catalogs collect metadata from systems and use it to create an indexed inventory of available data assets that includes data lineage details, search functions and collaboration tools. Information about data governance policies and automated mechanisms for enforcing them can also be built into data catalogs.
Best practices for managing data governance initiatives
Because data governance typically imposes restrictions on how data is handled and used, it can become controversial in organizations. A common concern among IT and data management teams is that they'll be seen as the "data police" by business users if they lead data governance programs. To promote business buy-in and avoid resistance to governance policies, experienced data governance managers and industry consultants recommend that programs be business-driven, with data owners involved and the data governance committee making the decisions on standards, policies and rules.
Training and education on data governance is a necessary component of initiatives. In particular, business users and data analysts must be familiar with data usage rules, privacy mandates and their own responsibility to help keep data sets consistent. Ongoing communication with corporate executives, business managers and end users about the progress of a data governance program is also a must. That can be handled through a combination of reports, email newsletters, workshops and other outreach methods.
Other data governance best practices to adopt include applying data security and privacy rules as close to the source system as possible, putting appropriate governance policies in place at every level of an organization and reviewing governance policies on a regular basis.
Gartner analyst Saul Judah has recommended an adaptive data governance approach that applies different governance policies and styles to individual business processes. He also has listed these seven foundations for successfully governing data and analytics applications:
- A focus on business value and organizational outcomes.
- Internal agreement on data accountability and decision rights.
- A trust-based governance model that relies on data lineage and curation.
- Transparent decision-making that hews to a set of ethical principles.
- Risk management and data security included as core governance components.
- Ongoing education and training, with mechanisms to monitor their effectiveness.
- A collaborative culture and governance process that encourages broad participation.
Professional associations that promote best practices in data governance processes include DAMA International and the Data Governance Professionals Organization. The Data Governance Institute, an organization founded in 2003 by consultant Gwen Thomas, has published a data governance framework template and a variety of guidance on governance best practices. Some of the information is openly available on its website, while other materials can be accessed only by paid members. Similar guidance is also available elsewhere -- for example, in the DataManagementU online library maintained by consulting firm EWSolutions.
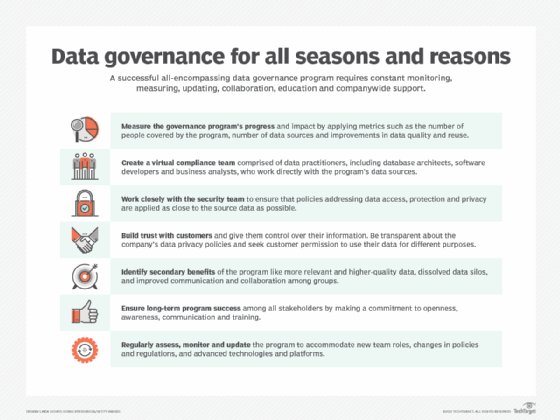
Data governance challenges
Often, the early steps in data governance efforts can be the most difficult because different parts of an organization commonly have diverging views of key data entities, such as customers or products. These differences must be resolved as part of the data governance process -- for example, by agreeing on common data definitions and formats. That can be a fraught and fractious undertaking, which is why the data governance committee needs a clear dispute-resolution procedure.
The following are some other common data governance challenges that organizations face.
Demonstrating its business value. Without upfront documentation of a data governance initiative's expected business benefits, getting it approved, funded and supported can be a struggle. In her September 2023 blog post, Askham said business executives need to understand at the outset of a governance program why the organization is investing in it and what's in it for them. Establishing the business drivers "makes it much easier to engage with and sell [an] initiative to senior stakeholders," she wrote.
On an ongoing basis, demonstrating business value requires the development of quantifiable governance metrics, particularly on data quality improvements. That could include the number of data errors resolved on a quarterly basis and the revenue gains or cost savings that result from them. In addition, common data quality metrics measure accuracy and error rates in data sets plus related attributes, such as data completeness and consistency. Other kinds of metrics that can also be used to show the value of a governance program include data literacy levels and awareness of data management principles among business users.
Securing sufficient resources and skills. As part of funding a governance program, organizations need to ensure that the required resources are assigned to it, from the leadership level on down. Getting the right participants involved is also crucial. As Askham wrote, "Appointing the wrong people to key roles can cause the wheels to come off any well-thought-out initiative pretty quickly." In some cases, it might be necessary to hire experienced workers to staff the data governance team or to bring in outside consultants to help with an initiative.
Governing data in the cloud. As organizations deploy more applications in the cloud and move existing ones there, cloud providers manage some aspects of data security and compliance with data privacy regulations. But companies are still responsible for data governance as a whole, and the same issues apply in the cloud as with on-premises systems. For example, under the concepts of data residency and data sovereignty, different data sets might need to be stored in particular geographic regions and managed according to the laws of individual countries to avoid privacy compliance issues. That can prevent a company from consolidating data in a single location and governing it in a uniform way.
Supporting self-service analytics. The shift to self-service BI and analytics has created new data governance challenges by putting data in the hands of more users in organizations. Governance programs must make sure data is accurate and accessible but also ensure that self-service users -- business analysts, executives and citizen data scientists, among others -- don't misuse data or run afoul of data privacy and security restrictions. Streaming data that's used for real-time analytics further complicates those efforts.
Governing big data. The deployment of big data systems also adds new governance needs and challenges. Data governance programs traditionally focused on structured data stored in relational databases, but now they must deal with the different types of data -- structured, unstructured and semistructured -- that big data environments typically contain. A variety of data platforms, including Hadoop and Spark systems, NoSQL databases and cloud object stores, are now common, too. Also, sets of big data are often stored in raw form in data lakes and then filtered as needed for analytics uses, further complicating data governance. The same applies to data lakehouses, a newer technology that combines elements of data lakes and the traditional data warehouses used to hold structured data for analysis.
Managing expectations and internal changes. Data governance is often a slow-moving process, so program leaders need to set realistic expectations on progress. Otherwise, business executives and users might start to question whether a program is on the right track. Many governance initiatives also involve significant changes, including both operational and cultural ones. That could lead to internal problems and employee resistance if a solid change management plan isn't built into a governance program.
Key data governance pillars
Data governance programs are underpinned by several other facets of the overall data management process. Most notably, these facets include the following:
- Data stewardship. As discussed earlier, a data steward is responsible for a portion of an organization's data. Data stewards also help implement and enforce data governance policies. Often, they're data-savvy business users who are subject matter experts in their domains. Data stewards collaborate with data quality analysts, database administrators and other data management professionals. They also work with business units to identify data requirements and issues.
- Data quality. One of the biggest driving forces behind data governance activities is creating high-quality data. Data accuracy, completeness and consistency across systems are crucial hallmarks of successful governance initiatives. Data cleansing, also known as data scrubbing, fixes data errors and inconsistencies; it also correlates and removes duplicate instances of the same data elements to harmonize how customers or products are listed in different systems. Data quality tools provide those capabilities through data profiling, parsing and matching functions, among other features.
- Master data management. MDM is another data management discipline that's closely associated with data governance processes. MDM initiatives establish a master set of data on customers, products and other business entities to help ensure that the data is consistent in different systems across an organization. As a result, MDM naturally dovetails with data governance. Like governance programs, though, MDM efforts can create controversy in organizations because of differences between departments and business units on how to format master data. In addition, MDM's complexity has limited its adoption. To make it less onerous, there has been a shift toward smaller-scale MDM projects driven specifically by data governance goals.
Data governance is also related to information governance, which focuses more broadly on how information is used overall in an organization. At a high level, data governance can be viewed as a component of information governance, but they're generally considered to be separate disciplines with similar aims.
Data governance use cases
Effective data governance is at the heart of managing the data used in operational systems, as well as the BI and data science applications fed by data warehouses, smaller data marts and data lakes. It's also a particularly important component of digital transformation initiatives, and it can aid in other corporate processes, such as risk management, business process management, and mergers and acquisitions.
As the importance of data and its uses in organizations continue to expand, and new technologies emerge, data governance processes are likely to be applied even more widely. Already, high-profile data breaches and laws like GDPR and CCPA have made building privacy protections into data governance policies a central part of governance efforts. There's also a growing need to govern the data used and created by machine learning algorithms, generative AI tools and other AI technologies. Gartner has predicted that 60% of organizations won't realize the expected business value of AI applications by 2027 because of governance shortcomings.
Data governance vendors and tools
Numerous vendors offer data governance tools. These include major IT vendors, such as IBM, Oracle, SAP and SAS Institute Inc., as well as data management specialists like Alation, Ataccama, Collibra, Informatica, OneTrust, Precisely, Quest Software, Rocket Software, Semarchy, Syniti and Qlik's Talend subsidiary. In most cases, the governance tools are part of larger suites that also incorporate metadata management features and data lineage functionality. In addition, vendors are now embedding machine learning algorithms, natural language processing capabilities and AI-driven automation to streamline various data governance tasks, such as policy enforcement, data classification and documentation of new data assets.
Many of the data governance and metadata management platforms include data catalog software, too. It's also available as a standalone product from Alation, Alex Solutions, Atlan, Data.world, Hitachi Vantara, IBM, OvalEdge and numerous other vendors, as well as cloud platform market leaders AWS, Google and Microsoft.
Craig Stedman is an industry editor who creates in-depth packages of content on business intelligence, analytics, data management and other types of technologies for TechTarget Editorial.
Jack Vaughan, a former senior news writer at TechTarget, contributed to this article.