data scientist
A data scientist is an analytics professional who is responsible for collecting, analyzing and interpreting data to help drive decision-making in an organization. The data scientist role combines elements of several traditional and technical jobs, including mathematician, scientist, statistician and computer programmer. It involves the use of advanced analytics techniques, such as machine learning and predictive modeling, along with the application of scientific principles.
As part of data science initiatives, data scientists often must work with large amounts of data to develop and test hypotheses, make inferences and analyze things such as customer and market trends, financial risks, cybersecurity threats, stock trades, equipment maintenance needs and medical conditions.
In businesses, data scientists typically mine data for information that can be used to predict customer behavior, identify new revenue opportunities, detect fraudulent transactions and meet other business needs. They also do valuable analytics work for healthcare providers, academic institutions, government agencies, sports teams and other types of organizations.
Data scientist was first used as a job title in 2008, simultaneously at Facebook and LinkedIn; four years later, a Harvard Business Review article famously called it "the sexiest job of the 21st century." The demand for data science skills has grown significantly over the years, as companies look to glean useful information from increasing volumes of big data and take advantage of artificial intelligence (AI) and machine learning technologies to enable new types of analytics applications.
Roles and responsibilities of data scientists
Data scientists play the lead role in data science applications in organizations. They're commonly tasked with finding information that enables more effective marketing campaigns, improved customer service, stronger supply chain management and better business decisions and strategies overall. To do so, they analyze sets of quantitative and qualitative data, depending on the needs of specific applications.
They might also be asked to explore data without being given a specific business problem to solve. In that scenario, a data scientist needs to understand both the data and the business well enough to formulate questions, do the analysis work and deliver insights to business executives on possible changes to business operations, products or services.
The basic responsibilities of a data scientist include the following activities:
- gathering and preparing relevant data to use in analytics applications;
- using various types of analytics tools to detect patterns, trends and relationships in data sets;
- developing statistical and predictive models to run against the data sets; and
- creating data visualizations, dashboards and reports to communicate their findings.
In many organizations, data scientists are also responsible for helping to define and promote best practices for data collection, preparation and analysis. In addition, some data scientists develop AI technologies for use internally or by customers -- for example, conversational AI systems, AI-driven robots and other autonomous machines, including key components in self-driving cars.
Characteristics of an effective data scientist
The personal characteristics and soft skills required by data scientists include intellectual curiosity, critical thinking, a healthy skepticism, good intuition, problem-solving abilities and creativity. The ability to collaborate with other people is critical, too. Data scientists typically work on a data science team that also includes data engineers, lower-level data analysts and others, and the role often involves working with various business teams on a regular basis.
Many employers expect their data scientists to be strong communicators who can use data storytelling capabilities to present and explain data insights to business executives, managers and workers. They also need leadership capabilities and business savvy to help steer data-driven decision-making processes in an organization.
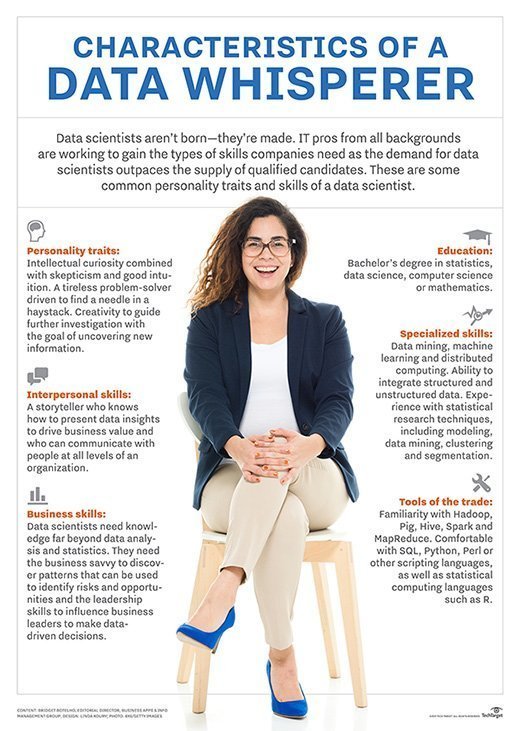
Qualifications and required skills
Data scientists must be able to complete a wide range of complex planning, modeling and analytical tasks in a timely manner. Given that, the job requires knowledge of various data science tools and libraries; big data platforms, such as Spark, Kafka, Hadoop and Hive; and programming languages that include Python, R, Julia, Scala and SQL.
Technical skills required for the job include data mining, predictive modeling, machine learning and deep learning, as well as upfront data processing and data preparation. The ability to work with a combination of structured, semistructured and unstructured data is often a requirement, too, especially in big data environments that contain different types of data. Experience with statistical research and analytics techniques such as classification, clustering, regression and segmentation -- is also a must. In some cases, expertise in natural language processing (NLP) is another prerequisite.
Examples of necessary skills listed in job postings include the following:
- expertise in all phases of data science, from initial data discovery through data cleansing and model selection, validation and deployment;
- knowledge and understanding of common data warehouse and data lake structures;
- experience with using statistical approaches to solve analytics problems;
- proficiency in popular machine learning frameworks;
- familiarity with common data science and machine learning techniques, such as decision trees, K-nearest neighbors, naive Bayes classifiers, random forests and support vector machines;
- experience with techniques for both qualitative and quantitative analysis;
- the ability to identify new opportunities to apply machine learning and data mining tools to business processes to improve their efficiency and effectiveness;
- experience with public cloud platforms and services;
- familiarity with a wide variety of data sources, including databases and big data platforms, as well as public or private APIs and standard data formats, like JSON, YAML and XML;
- the ability to aggregate data from disparate sources and prepare it for analysis;
- experience with data visualization tools, such as Tableau and Power BI;
- the ability to design and implement reporting dashboards that can track key business metrics and provide actionable insights; and
- the ability to do ad hoc analysis and present the results in a clear manner.
Education, training and certifications
Most data science jobs require at bare minimum a bachelor's degree in a technical field. More commonly, though, data scientists have an advanced degree in statistics, data science, computer science or mathematics. In the 2021 version of an annual survey on machine learning and data science conducted by Google subsidiary Kaggle, 47.7% of the 3,600-plus respondents employed as data scientists said they had a master's degree, while another 15% had a doctorate.
By comparison, 30.1% had a bachelor's degree, according to the survey. But Kaggle, which runs an online machine learning and data science community, noted that the percentage of respondents with undergraduate degrees only has increased in recent years. That might reflect the strong demand for data scientists in organizations. (The 2022 survey results released publicly by Kaggle don't include education data.)
Both prospective and experienced data scientists can also take advantage of boot camps and online courses offered by educational platforms such as Coursera, Udemy and Kaggle itself. In addition, there are various certification opportunities available through universities, technology vendors and industry groups.
Retraining professionals who work in other positions or fields to become data scientists is another option for organizations. That might include database developers and software programmers, as well as traditional scientists and other experts in particular disciplines.
Data scientist salaries
Because the desired combination of analytics skills, personality traits and experience is still somewhat hard to find, qualified data scientists generally can command six-figure salaries, at least in the U.S. According to job posting site Indeed, the average data scientist salary in the U.S. was $144,959 as of October 2022, based on about 3,800 reported salaries. Indeed said the average pay was $122,591 for data scientists with less than a year of experience and $167,038 for those with three to five years of experience.
Job search and company reviews site Glassdoor ranked data scientist No. 3 on its "50 Best Jobs in America for 2022" list, which is based on a combination of median base salary, job satisfaction levels and available openings. As of October 2022, Glassdoor's data showed median total compensation of $124,100 for U.S.-based data scientists, including base salary plus bonuses and other payments. That increased to an average of $159,957 for a lead data scientist and $162,262 for a senior data scientist.
Data scientist vs. data analyst
The role of data scientist is often confused with that of data analyst. But while there is overlap in many of the job responsibilities and required skills, there are also some significant differences between data scientists and data analysts.
The duties of a data analyst can vary depending on the company. In general, though, they don't have the full level of technical skills that data scientists need, and they might also be less experienced. They still collect, process and analyze data, as well as creating visualizations and dashboards to report findings; some data analysts also design and maintain the databases and other data stores used in analytics applications.
However, data analysts often support the work of data scientists and are overseen by them in analytics initiatives. The additional responsibilities and expectations of data scientists also amount to much higher salaries. The median compensation in the U.S. is $71,645 for a data analyst and $102,831 for a senior data analyst, according to Glassdoor. Indeed similarly lists an average base salary of $71,072 and a $2,000 bonus for data analysts.
Data scientists vs. citizen data scientist
In addition to skilled data scientists, many organizations now rely on citizen data scientists to do some analytics work. They can include business intelligence (BI) professionals, business analysts, data-savvy business users and other workers who get involved in data science initiatives. The differences between the two groups include the following:
- Education. While data scientists usually have relevant degrees, citizen data scientists might have a wide variety of educational backgrounds and little or no formal training in data science. But they typically have gained experience with analytics tools and systems that enables them to create models and do relatively complex analysis work.
- Coding. Citizen data scientists generally rely on software that includes prebuilt analytical modeling tools, drag-and-drop features and user-friendly algorithms to perform standard analyses. That doesn't prevent them from discovering useful patterns or data points, but professional data scientists are able to create complex custom algorithms and approach data analysis in more advanced ways.
- Salary. As noted above, data scientist is a high-paying job. On the other hand, citizen data scientists could be hobbyists or volunteers who aren't paid anything extra beyond their regular salaries, although some receive additional compensation for the data science work they do.
Major areas of data science
The key aspects of a data scientist's job include the following disciplines:
- Data preparation. The first step in data science applications is to collect and prepare the data that will be analyzed. Data preparation is the process of gathering, cleansing, organizing, transforming and validating data sets for analysis. Data scientists often work together with data engineers during the data prep phase.
- Data analytics. Analyzing data to identify trends, correlations, anomalies and other useful information is the main purpose of data science initiatives. Overall, the analytics work done by data scientists is aimed at improving business performance and helping organizations gain a competitive advantage over business rivals.
- Data mining. As part of data analytics efforts, this involves working to uncover patterns and relationships in large data sets. Data mining typically is done by applying advanced algorithms to the data that's being analyzed. Data scientists then use the results generated by the algorithms to create analytical models.
- Machine learning. Increasingly, data mining and analytics are driven by machine learning, in which algorithms are built to learn about data sets and then find the desired information in them. Data scientists are responsible for training and overseeing machine learning algorithms as needed. Deep learning is a more advanced form that uses artificial neural networks.
- Predictive modeling. Data scientists commonly also must be able to create predictive models of different business scenarios to analyze potential outcomes and behavior. For example, models can be built to predict how different customers likely will respond to marketing offers or to assess the possible indicators of diseases.
- Statistical analysis. Data science work also involves the use of statistical analysis techniques to analyze data sets. Statistical analysis is a core facet of what data scientists do to explore data and find underlying trends and patterns for analysis and interpretation.
- Data visualization. The findings of data science applications are usually organized into charts or other types of data visualizations so business executives and workers can easily understand them. In many cases, data scientists combine multiple visualizations into reports, interactive dashboards or detailed data stories.
Challenges that data scientists face
Although they have what's considered to be one of the best jobs available, data scientists still experience some challenges and complications. Data science work is generally complex because of its advanced nature and the large amount of data that often must be analyzed. Also, because data scientists aren't always given specific analytics questions to answer or directions on how to focus their research, it sometimes can be hard to ensure that what they do meets business needs.
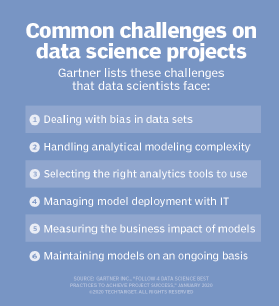
Gathering relevant data for analytics applications can be difficult, too, especially in organizations with data silos that are isolated from other IT systems. Incorrect or inconsistent data can erroneously skew the results of analytics models; to avoid that, rigorous data profiling and cleansing is required upfront to identify and fix data quality issues. Overall, data preparation is time-consuming: A common maxim is that data scientists spend 80% of their time finding and preparing data and only 20% analyzing it.
Identifying and addressing biases in data science applications is another big challenge, both in the data being analyzed and in algorithms and analytical models. Maintaining models and ensuring that they're updated when data sets or business requirements change can also be problematic. And analytics workloads might be hard to handle if companies don't invest in a full data science team.