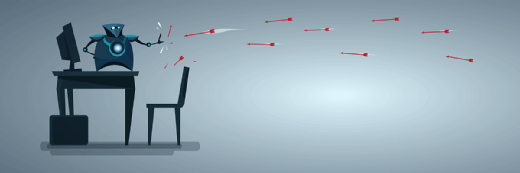
Role of AI in cybersecurity and 6 possible product options
Cyberthreats loom large in this modern IT environment. Explore the six most common roles of AI in cybersecurity and the products synthesizing them.
Companies are turning more to AI to aid in their security efforts in modern IT environments. The exponential growth of data, devices, processing power, algorithms and networked systems -- valuable assets for any business competing in the 21st century -- comes with newer risks and vulnerabilities. Citing data security, infrastructure protection and cloud security as the fastest-growing areas of security spending, Gartner estimated companies will spend some $137 billion on cybersecurity risk management in 2019, according to a December 2018 report.
With this new reality, companies have realized reactive measures are not enough; they must not only scale and automate threat response programs, but develop proactive measures as well.
AI capabilities are powered by a range of techniques, such as machine learning, deep learning, computer vision and natural language processing, to detect patterns and make inferences. In the cybersecurity domain, the role of AI in cybersecurity is to recognize patterns of user, data, device, system and network behavior to distinguish anomaly from normal. It also helps admins analyze massive data and investigate a tsunami of new threat types, as well as respond faster and address threats preemptively.
Based on Kaleido Insights research and analysis across the cybersecurity market and vendors, below are six common use cases, featuring a few of the numerous vendors paving the way for the next generation of cybersecurity products.
1. Human security analyst and SOC augmentation
One of the most common use cases for the role of AI in cybersecurity is support for human analysts. After all, AI is unlikely to ever replace experienced security analysts. It is far more likely to augment humans in areas where machines excel, such as analyzing big data, eliminating fatigue and freeing up humans from tedious tasks so they can utilize more sophisticated skills, like creativity, nuance and expertise. In some cases, analyst augmentation involves incorporating predictive analytics into security operations center (SOC) workflows for triage or querying big data sets.
Darktrace's Cyber AI Analyst is a software program that supports human analysts by only surfacing high-priority events. Meanwhile, it queries massive data and pivots across networks to gather context for investigations, conduct them and sort out low-priority cases. Trained on data sets developed over thousands of deployments by analyzing how Darktrace's own expert analysts investigate alerts, Cyber AI Analyst uses a variety of machine learning, deep learning and mathematical techniques to crunch n-dimensional data, generate thousands of queries at machine speed and investigate all parallel threats simultaneously.
2. New attack recognition
While malware or other types of threat detection have been around for years -- often matching suspect code with signature-based systems -- AI is now shifting techniques toward inferencing to predict new attack types. By analyzing massive amounts of data, event types, sources and outcomes, AI techniques are able to identify novel forms and types of attacks. This is critical because attack techniques continue to evolve alongside other advancements.
FireEye Inc. offers a promising example of new attack recognition in its MalwareGuard product. It uses machine learning algorithms to find new, morphed or advanced attacks where a signature either has not been created or does not yet exist. Its engine utilizes both private and public data sources, including some 17 million deployed endpoint security agents, attack analyses based on more than 1 million attack response hours spent, and adversarial intelligence collected across a global and multilingual network of security analysts.
3. Behavioral analytics and risk scoring
The behavioral analysis techniques that have been pioneered in less critical areas, such as advertising, are now making their way toward critical use cases for identity authentication and antifraud. Here, AI algorithms mine massive user and device behavioral patterns, geolocation, login parameters, sensor data and countless other data sets to derive a score or likelihood that users are who they say they are.
Mastercard's NuData Security is one platform that takes advantage of multifactor big data analysis to assess risk and develop a dynamic profile of each event for endpoint and user security. The company uses machine and deep learning to analyze four areas:
- Behavioral data: browser type, traffic changes, surfing speed and time on page.
- Passive biometrics: a unique user's typing speed, device angle, keystrokes and pressure.
- Device intelligence: the specific device's known versus new connections, locations and network interactions.
- Behavioral Trust Consortium: Mastercard's big data repository that analyzes billions of data points at the population level.
4. User-based threat detections
From rogue insiders to privilege abuse and admin misuse to nefarious actors, humans represent significant and diverse vectors of cyber-risk. As a result, AI techniques are emerging to detect shifts in how users interact within IT environments and characterize their behaviors in the context of an attack.
LogRhythm Inc. is honing in on user-based threat detection using CloudAI, a fully integrated add-on to its LogRhythm NextGen SIEM Platform. Specifically, the company maps disparate user accounts -- VPN, work email, personal cloud storage -- and related identifiers, such as username and email address, to the actual user's identity in order to build comprehensive behavioral baselines and user profiles. Moreover, CloudAI is designed to evolve over time for both current and future threat detection. Analysts train the system in the normal course of investigation and collect data from across the platform's extended customer footprint for threat training. CloudAI can also configure models to self-heal through continuous tuning without manual intervention.
Vectra AI Inc. takes a differentiated approach to this use case by analyzing attack lifecycles. Using some 60 machine learning models to analyze all behaviors an attacker could perform across an attack lifecycle -- including remote access tools, hidden tunnels, backdoors, reconnaissance tools, credential abuse and exfiltration -- its Cognito platform claims to flip the traditional approach to user-based threat detection on its head by providing the defender with multiple opportunities to detect an attacker.
5. On-device detection across endpoint kill chain
The rise of mobile devices in the enterprise has ushered in a new era of cybersecurity threats and altered the nature of endpoint security. Whereas enterprises typically managed traditional endpoints, like laptops, today's "system admin" of mobile is the end user. Whether an employee, consumer or hacker, this individual dictates downloads, applications, communication channels and network interactions. Further, apps are generally in their own containers, limiting traditional patch management. What this fundamentally different configuration amounts to is that attackers aim to be persistent by delivering exploits for root access, compromising the full device, while effectively sidestepping enterprise networks. As a result, mobile endpoint protection must secure the entire kill chain -- from phishing attempts to fake applications or networks to all manner of different malicious attack types. Here, admins apply machine learning across each of these attack vectors, rather than deploying different detection systems for each, in order to predict the likelihood that any given point interaction threatens systemic takeover.
Zimperium, a firm specializing in mobile endpoint security, uses machine learning to deliver on-device detections across the entire mobile kill chain, monitoring all malware, phishing, device, app and network interactions. While not running machine learning models on device today, Zimperium deploys machine learning-based detection on device derived via cloud-based deep learning techniques. In use across more than 70 million devices, it monitors anonymized data from all vectors across all malware, phishing, device, app and network interactions, using the cloud to analyze specific attack pathways, identify noise from signal, run test scenarios and deploy classifiers to improve logic and algorithms, which are then applied to on-device detection. This feed-refine-feed-refine feedback loop is critical to best detect current and new threat types -- across the entire kill chain -- before they attack or achieve persistent takeover.
6. Proactive security in disconnected environments
As data and devices permeate the physical world, the ability to secure and reduce mean time to detect and mean time to respond becomes a question of connectivity and compute power. Increasingly complex technical infrastructures mean greater demand for the safety, security and efficiency of their operations, which serve as table stakes to realize the value of data in mission-critical environments, such as aviation, energy, defense and maritime. More computationally intensive AI applications remain nascent in these environments, but new techniques are emerging to facilitate machine learning-based security for script, file, document and malware analysis via on-premises support.
SparkCognition, which bills itself as an AI company rather than a security company, supports applications in disconnected environments. In a current deployment with a 911 dispatch center, a place that tends to operate in a disconnected environment due to the sensitive information it hosts, SparkCognition's DeepArmor runs via an on-site management console. Specifically, DeepArmor uses machine learning for static file analysis of some 20,000 unique file features to determine likelihood of malicious activity within a few seconds. While admins must perform model updates manually in these environments, DeepArmor is signature-free, meaning it does not require daily signature scans.
The role of AI in cybersecurity is expanding
Of course, there are additional, more minor use cases for applying machine learning and deep learning to cybersecurity needs, including the following:
- big data query generation and analysis
- threat proliferation and spread detection
- autonomous response
- agent consolidation and deployment across other security tools
- threat blocking automation
- malware classification
- attack classification (unknown, insider, persistent)
- false positive reduction
- product self-healing
- machine data comprehension (over 800 different device types)
- encrypted traffic analysis
- policy compliance analysis
- cyber-risk insurance
- cyber-risk due diligence augmentation (pre-mergers and acquisitions)
For all its potential, machine learning is not a silver bullet -- it is a just a tool. AI depends on data, and in a security context, this doesn't just mean big data. It means multilingual, real-time data and, most importantly, good data. Its success demands collaboration between security experts and data scientists.
Despite lofty marketing claims, the reality is enterprise security landscapes are vast, dynamic networks that admins must constantly monitor, audit and update based on ongoing, unpredictable, internal and external threat vectors. AI introduces a variety of promising enhancements to abilities to detect, investigate and respond to threats, but it's the human-plus-technology combination that can truly manage the full spectrum of threats within an ever-evolving security landscape.