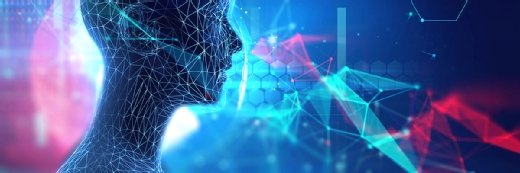
Getty Images
Exploring Data De-Identification in Healthcare
A healthcare data expert discusses the nuances of data de-identification in healthcare, including HIPAA compliance, connected devices, and the role of AI.
Adequately de-identifying healthcare data is critical for health systems, payers, and other stakeholders to ensure HIPAA compliance. However, the advent of newer technologies, such as artificial intelligence (AI) and connected devices, has created questions about ensuring patient privacy while enabling data sharing and access to improve care and drive medical breakthroughs.
Suraj Kapa, MD, a cardiac electrophysiologist with Mayo Clinic and chief medical officer for healthcare data privacy startup TripleBlind, sat down with HealthITAnalytics to help shed light on de-identification in healthcare and its relationship with HIPAA compliance, AI, and connected devices.
GOING BEYOND PATIENT DATA EXTRACTION
When discussing data de-identification in healthcare, it’s important to understand why it’s such a hot topic before diving into the question of how to enable it.
“I think for most, even clinicians and lay people, the understanding of why we want to de-identify is pretty self-evident because of the entire principle of HIPAA and trying to avoid [exposing] an individual's personal health information, which for a wide variety of insurance and other reasons should remain private, needs to remain private,” Kapa said.
At its most basic, de-identification refers to the principle of being unable to re-identify a person based on the information in their medical record, which often involves removing or hiding information such as the individual’s name, date of birth, gender, or address.
Beyond this basic level of de-identification to obscure explicitly personal information, healthcare stakeholders need to be aware of additional information and levels of identifiability to protect patient information.
“There's actually a level beyond that of potentially identifiable information,” Kapa noted. “Things like having somebody's IP address or the unique device ID associated [with] their pacemaker. Or, say, their medical record number, which might only be visible within the organization where that patient's being seen.”
In theory, this information could still be used to re-identify someone fairly easily, albeit with some additional work and effort. These levels of de-identification continue until the individual is no longer readily identifiable, Kapa indicated.
“In other words, there are additional safeguards and controls that go beyond the mere extraction of personally identifiable information,” he said. “So fine, you eliminate the medical record number, you eliminate the name, you eliminate the address, you eliminate all this other stuff from individual records. However, say you're running a large analytic function across, say, the US, on patients with a specific type of cancer and trying to understand what we call social determinants of health.”
In this example, Kapa explained that income level might impact the choice of chemotherapy regimen, leading to one billionaire receiving a particular treatment for a specific type of cancer in the last year. By virtue of association with elements like treatment regimen chosen, cancer type, and timeframe, along with information that could be available outside the health setting, like on a social media platform, this individual could become potentially identifiable.
“So, when we think about de-identification, there's really two aspects to it,” Kapa stated. “One is, I think, the things people most talk about or think about, which is the extraction of specific elements that can be associated with a specific individual or in tandem can be brought to a specific individual.”
“Then there's a second aspect,” he continued, “Which is how a combination of factors within a data set or group of data sets can be used to home in on one specific individual. And that is not done solely by virtue of extracting specific information, but also by limiting how the intersection of queries distributed to a data set will result in homing in on any specific individual.”
AI, HEALTH DATA, AND HIPAA COMPLIANCE
In the healthcare setting, data extraction considerations have a role to play in HIPAA compliance, but evolving data analytics technologies, such as AI, present new challenges and opportunities for HIPAA-compliant data de-identification.
“[AI] is exactly where people can potentially run into issues when they use traditional definitions of de-identification,” Kapa indicated. “Because yes, you've extracted the name, the medical record number, and factors like that out of an image, [for example,] out of a CT scan of a patient’s head that you're using to identify face [or] neck cancer. But the thing is, it's fairly well known that you can do reconstructions from CTs. You can actually allow for reconstruction of facial characteristics.”
Because AI enables higher degrees of image reconstruction than traditional analytics frameworks, the tech allows for a more robust re-identification schema, he explained. This could theoretically help a user re-identify a patient through something like a reverse Google image search without any other potentially identifiable information.
This necessitates going beyond the extraction of identifiable information in two ways.
“Number one is how do you obfuscate the core data enough, whether by mathematically transforming it, cryptographically transforming it, or otherwise, in order to effectively make it, essentially, at an individual data point level, non-visible to the data user or to other people who might try to obtain access to that data or intercept that data,” Kapa stated.
“Number two, [is] how do you ensure that the analytic operation being done is not one that has its core purpose as the identification of cohorts of individuals?” he said. “And I would say, for each of those processes, there's both technical considerations and considerations that are based on compliance standards and some degree of manual approaches.”
Ensuring that the data are not replicated in multiple data groups so that individuals cannot intercept or use them maliciously is critical. This is where the concept of privacy enhancing technologies (PETs) comes into play. These technologies, many of which are AI-based, help users and organizations preserve privacy throughout the data lifecycle.
PETs allow for limitations on the movement of data, rather than just on the extraction of specific data identifiers, while allowing for analytic operations to be performed on that data, Kapa explained. This helps limit risk and allows one data element to be used in multiple ways without jeopardizing patient privacy.
While this doesn’t eliminate risk entirely — Kapa noted that due diligence in terms of understanding the algorithmic processes being run on the data and strong communication between data user and data owner is vital — these technologies and approaches highlight the potential value that providers and patients can receive by enabling access to data via AI-driven de-identification.
“Since the dawn of medicine, the dawn of healthcare, the understanding of the value of sharing data and collaborating over data to realize improved health outcomes has been clear,” Kapa stated. “The reality is, I think it's hard to get people to dispute the importance of actually sharing the data. But in this day and age, especially with as much data as we're producing, by as many elements about a person as we're looking at, the open sharing of such data [while] considering the privacy risk to the individual has become paramount.”
AI has significant promise to help researchers gain insights into disease processes and improve treatments, but this cannot be achieved without widespread access to multiple, diverse datasets that represent broad swaths of populations, he continued.
Doing so while weighing privacy risks to individuals is another aspect of this process that PETs can help bolster.
“[PETs] actually allow for the large-scale, diverse collaboration between entities, while preserving and offering promises around mitigation of the risk of re-identification, [which] can allow for the patients and the providers to actually gain benefit by potentially finding that next new drug that acts on this specific gene that this specific patient has that otherwise would never have been identifiable without seeing a broad enough dataset,” Kapa explained.
Without access to these data, this collaboration and analysis will be severely limited, and progress could stall. However, accessing large, diverse datasets requires a broader understanding of healthcare data de-identification moving forward.
“That's kind of where considering just the traditional ‘let's obfuscate or extract the individual identifiers’ [approach to de-identification] is going to limit the potential growth and explosion of digital health opportunities in terms of how it can potentially translate to actual measurable outcomes,” he said.
Considering both the privacy of individuals and access to data is the only way to achieve these growth opportunities, but doing so becomes more complicated when considering connected devices and how HIPAA impacts the data they transmit, Kapa noted.
THE COMPLEXITIES OF CONNECTED DEVICES
When one thinks about connected devices in terms of transmitting personal information to a hospital, there is a ‘contract’ between a hospital and a patient, Kapa explained. Seeing how HIPAA impacts the data being transferred by that device is relatively straightforward.
However, as with de-identification, there are complexities when considering connected devices and HIPAA compliance.
Kapa used the example of getting a chest X-ray to illustrate his point. In such a scenario, the data broker, or the one who stores the data, could be the health system where the X-ray was performed or a third party, which creates additional considerations for HIPAA compliance.
“Where [this process] becomes more complicated is when the data broker ends up being a company or a different entity that's doing broad storage of that data,” he said. “I think it's established that there are still processes related to FDA approval of diagnostic devices and how that data is stored, [but] there needs to be some agreement about the storage of the data between that patient and that system.”
The issue becomes even more complex when one considers that the patient’s data is not just stored at their doctor’s office, but is more broadly distributed within personal cloud-based devices that only certain people have access to, potentially larger databases owned by a specific company, or other cloud-based vendors, as well as on-premises elements within a connected device itself, Kapa noted.
These data then cannot be accessed without a specific interaction with that device because there’s no other way to move that information. From there, the question of how to intersect all of these data points and assets to get a more holistic understanding of a particular individual or patient becomes salient.
According to Kapa, this presents a significant challenge as more healthcare occurs at home, which facilitates the need for data to be collected in much more diverse ways in many more database types with various data standards for information to be gathered about just one individual.
“So, how do you identify person X when group A stores everybody according to medical record number one, group B stores everything according to a personal cloud, and there's no identifiable information otherwise within it because that person just has a password to that cloud, but there's no actual identifiable information otherwise?” Kapa said. “And then to C, where they actually have identifiable information, and you need to cross-reference across all of these to get that holistic understanding. So it is a complex consideration. It's not insurmountable; it's a technical consideration of how you align all of these together in order to create this alignment between these different datasets.”
At the end of the day, however, all of these considerations circle back to reconciling older privacy frameworks, like HIPAA, with newer technologies that can expand privacy protections and evolve the idea of what it means to identify something in healthcare.
“Healthcare is, by necessity, a conservative beast. We don't want healthcare to be super innovative, on the edge…because you don't want to create risk when it comes to people's individual health. Period,” Kapa stated. “So, we want that level of sure certainty about how things work, why they work as they do, and have that appropriate framework to justify the use of novel things, whether it be a drug, technology, et cetera.”
Further, he explained that when discussing something like de-identification in healthcare, it requires the education and alignment of various groups, such as clinicians, researchers, regulatory bodies, lawyers, and expert certifiers of HIPAA compliance, alongside the evolution of legal frameworks to define what it means to appropriately de-identify healthcare data.
Kapa cited an example that highlights the need to evolve the understanding of healthcare data de-identification within HIPAA.
“If you read traditional HIPAA law where it says, okay, you just need to obfuscate the 18 common identifiers, but the 18th identifier is any combination of data that can potentially identify an individual… this [theoretically] ends up becoming way too broad,” he said.
He compared the scenario to a game of 20 questions, in which someone could glean the correct answer after three questions, while another person may not get it after all 20. This creates additional issues within the context of de-identification of healthcare when trying to decide what the leverageable standard for fully and completely de-identifying data is so that it can’t be re-identified.
Kapa indicated that these challenges might be addressed by rapidly evolving technical and legal considerations that go beyond stripping name, date of birth, and other identifiers out of records.
“Aligning the thinking of people who are purposefully and reasonably conservative in their thinking based on laws that have been set since the eighties, that's going to be required in order to actually [be successful, and] so that these frameworks aren't mired in this old thinking that actually subjects the data to even higher risk because you're going by older frameworks that aren't considering newer technologies that actually expand the level of protections, even if they don't seem to perfectly coincide with the older frameworks of how to do things,” he concluded.