What is clinical AI?
Clinical AI deploys artificial intelligence in clinical settings, specifically to support medical decision-making and patient care. Its purpose is to improve patient outcomes within the medical care setting, through increasing the speed and accuracy of diagnoses and automating administrative tasks.
A clinical setting is a healthcare environment where medical professionals actively engage in caring for patients, as opposed to medical research or training. Clinical settings include hospitals, healthcare centers, urgent care clinics and rehabilitation centers. The AI used in these environments is tailored for practical medical care and treatment, not for broader healthcare bureaucracy. The kinds of professionals using clinical AI would include doctors, nurses and other medical practitioners.
AI has been introduced into various aspects of clinical care, such as interpreting radiology scans and analyzing ECG readings. By training the AI model on relevant patient data, clinical AI can be used to identify patterns and spot early signs of disease that may not yet be visible to the human eye. Other uses of AI may be more administrative in nature, such as creating automated records of doctor-patient conversations and highlighting the most important details within clinical paperwork for easier discovery.
The difference between clinical AI and healthcare AI
Since clinical AI specifically refers to AI tools and systems that directly support medical decision-making and patient care, it can be understood to be a component of healthcare AI. However, healthcare AI and clinical AI are not synonymous. While clinical AI is a valuable example of the many ways AI is used in healthcare, it is only one piece of the puzzle.
This article is part of
AI in healthcare: A guide to improving patient care with AI
- Which also includes:
- Health IT (health information technology)
- precision medicine (PM)
- telehealth (telemedicine)
Healthcare AI is the umbrella category under which clinical AI falls. Healthcare AI refers not just to the use of AI in clinical settings, but to the use of AI in all areas of the industry, including financial and administrative applications. Examples of other areas where healthcare AI may be used include the following:
- Insurance processing.
- Hospital resource management.
- Healthcare marketing.
- Medical research.
- Population health management.
- Supply chain optimization.
So, while all clinical AI is healthcare AI, not all healthcare AI is clinical AI.
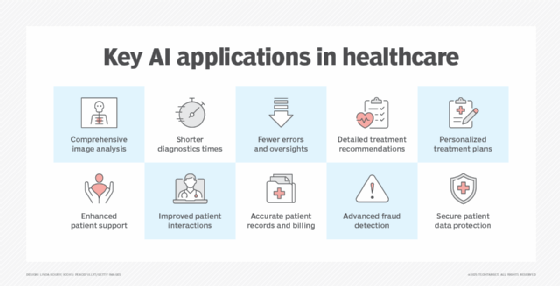
What are some examples of clinical AI?
Clinical AI is focused on improving patient outcomes, so its current and future applications involve many of the day-to-day processes that make up patient care. The intention is to equip medical practitioners with more accurate information at faster speeds, so they can take more effective action and provide greater quality of care.
This is still an evolving field. However, clinical AI is already being used in the following areas:
Medical Imaging
Doctors will often use scans such as MRIs and X-rays to better capture a patient's condition. These images are then analyzed to determine the best medical protocol. With clinical AI, radiology and cardiology teams can better detect the early signs of tumors, heart disease and other issues that may not be obvious to the human eye. Since AI compares the patient's personal scan with thousands of other data points, it may be able to detect patterns and identify diseases at earlier stages, which means earlier intervention and typically more effective treatment.
Other examples of clinical AI in medical imaging include the following:
- Detecting fractures in bone X-rays.
- Flagging suspicious areas in mammograms as part of breast cancer screenings.
- Reviewing cardiac function on MRIs of the heart.
Patient Monitoring
Many patients treated in a clinical setting will be connected to various monitors to track their vitals, such as a heart rate monitor. While medical practitioners will review these routinely, clinical AI can also be deployed to monitor these statistics and send out an alert if they move outside of an approved range. This is not a replacement for human review, but an extra monitoring process to minimize the risk of any patient getting overlooked.
This data monitoring can also have longer-term use, such as tracking trends in a patient's data that may reveal something deeper about their condition. This pattern detection can also be used to flag potential complications or deterioration before they happen, so doctors can take preemptive action.
Clinical Decision Support
When a patient enters a clinical setting for treatment, there are several key stages to their care. The first is in the initial intake and risk assessment, which is where doctors evaluate the patient's care needs and determine how best to begin treatment. Currently, many doctors use a clinical decision support system to help them with this work: doctors can input the patient's medical history and check for any potential health risks that they should be aware of.
They can also verify if they need to conduct any specific screenings for that patient, which may not have been flagged simply from the patient's current medical needs. This contributes to a more well-rounded view of the patient so that their healthcare can be more comprehensive. With clinical AI, these services would be improved by cross-referencing across much greater volumes of patient data.
Another key stage is treatment planning, which includes any medication prescriptions. In these instances, clinical AI can support the doctor by checking for any potential drug interactions that may occur between the newly prescribed medication and their current prescriptions. AI can also use patient data to suggest appropriate dosages and even recommend specific treatments, based on existing evidence. While the doctor will always make the final decision, AI can be a useful way to double-check details and increase speed and confidence in decision-making.
Documentation
Medical care produces a vast amount of paperwork that tracks all aspects of the patient's care, for both care and billing purposes. This documentation is very important, but can be time-consuming to record, especially when the medical practitioner has many patients who all need hands-on care. Today, clinical AI is being implemented to automate much of this paperwork creation so that doctors can reduce the amount of time they spend on reporting and increase the amount of time they spend on actual caregiving.
In practice, this means using AI to transcribe real time doctor-patient conversations and then convert these transcripts into the right format for clinical notes. These will still need manual review but enable the doctor to speed up their documentation. These auto-generated reports can then also be set up to highlight the most relevant data for follow-up action. Since they are digitized, it is easy to search them as well for key information.