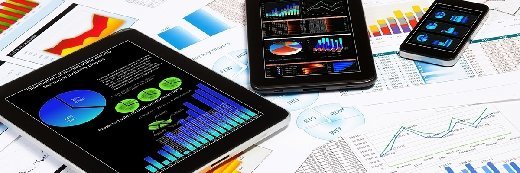
Sergey Nivens - Fotolia
Data governance for self-service analytics best practices
Establish a strong data governance strategy that will support your self-service analytics environment by including these best practices in your organization's process.
Data governance practices were conceived in an era when small teams of skilled analysts were responsible for the bulk of BI and analytics. Since those early days, data governance has been complicated by the rise in quantity and processes around data.
While these efforts promise to unlock the value of data, they also come with increased risks, which can impact an enterprise's bottom line. Especially in a self-service BI environment, it's important to establish best practices for data governance to avoid those risks.
What data governance means for self-service BI
Without simple data governance for self-service analytics, users are likely to turn to shadow IT.
"If people cannot effectively leverage the data they need because of an antiquated governance mechanism, then they often find a way to just get it to Excel," said Daren Thayne, CTO at Domo, a cloud BI service. Once that happens, the data governance strategy that data management teams thought was in place goes out the door.
Rosaria Silipo, principal data scientist at KNIME, has explored the challenges of good governance firsthand as her team grows self-service analytics.
"The only way to keep the data quality at a sufficient level and the different data pieces compatible with each other is to enforce governance strategies," she said.
Others discovered that good governance practices can keep costs down. Without guardrails, citizen data scientists can accidentally rack up a substantial cloud bill for a seemingly simple query that was structured the wrong way.
"IT should assess which queries are too costly, time-consuming or processor-intensive," said Matt Bertram, CEO and SEO strategist at EWR Digital, a digital marketing agency.
Bertram's team has explored ways to empower business owners to better monitor marketing campaign ROIs while IT team members keep a watchful eye and help as required.
Governance as code
One useful strategy is to apply the same principles of infrastructure as code to governance processes. In this model, all policies, processes and practices are explicitly captured as code, which can be versioned and managed using a source code repository like GitHub. This makes it easy to automate all aspects of data governance in line with the data engineering tasks required for analytics.
This can reduce the risk of errors in manually configuring the data flows for a new BI dashboard.
"Data teams using inefficient, manual processes often find themselves working frantically to keep up with the endless stream of analytics updates and the exponential growth of data," said Christopher Bergh, founder and CEO of DataKitchen, a DataOps consultancy and platform provider.
Augment the context
"In traditional analytics, the consumer of the data can more reliably be expected to understand the context of the data, its timeliness and its quality," said Craig Stewart, CTO at SnapLogic, a data automation and integration company.
In a self-service situation, it becomes more important that the data available to the user is top quality, up to date and in a format that they can rely on and understand. Because of this, data governance and the availability of metadata is important so users have data resources that are easily understandable and available.
"Self-service BI needs to provide the user with information on how data should be applied in a business context; otherwise it can be interpreted incorrectly, in a way that can cause harm to the business," said Peggy Tsai, vice president of data solutions at BigID, a data intelligence firm.
She recommends standardizing business definitions and having full documentation of data usage, including the proper business context of how the data should be used in a calculation or metric.
Transparency and clear communication
"Data governance is as much about mindset as it is about policies -- without buy-in from every level of an organization, even the best governance policies will fall by the wayside," said Triveni Gandhi, data scientist for responsible AI at Dataiku, an AI collaboration platform.
Transparency and clear communication are at the core of any successful data governance strategy. Policies need to be clearly and effectively communicated to employees in all departments. If employees aren't aware of their company's policies, they can't be expected to effectively enact them.
Promote data understanding
Users of a self-serve analytics system must know how data is collected and calculated, and what each piece of data represents, said Robert Izquierdo, head of product management at Target Media Partners.
"If you don't realize the difference between exit and bounce rates, you're going to make website changes that can damage your conversions," Izquierdo said.
Google Analytics, one of the largest self-serve analytics platforms around, clearly communicates the meaning of data points it provides. Google Analytics publishes definitions for the data it provides and makes these publicly accessible through its support pages so users understand how to analyze the data available to them.
Trust but verify
Data governance for self-service analytics should use data to monitor and label how the data is being used.
"Sometimes this means correcting an incorrect use of data, but it can also mean finding a new insight that would have been lost to most of the organization in an email attachment somewhere," said Ben Schein, vice president of data curiosity at Domo.
Users who are closer to the business engaged with the data might be able to identify data quality issues that a machine or data scientist might have missed. A smartly governed self-service environment should also be able to delineate between certified data and insights -- for example, insights approved by the finance department -- and user-generated analysis.
"You want your users generating new content and exploring the data, but you need to ensure users understand when they are looking at an innovative exploration or an official metric," Schein said.
Establish responsibility and ownership
It must be clear who owns which part of the data; who is responsible to keep it up to date and in the right shape; and who is in charge of extracting what information, shaping it and delivering it.
"Responsibility and ownership are the most important thing in data governance," Silipo said.
Data can and will change, processes will be different, and the responsible parties must take consequent actions to make sure the data repository and extracted BI are maintained at the highest standards.
Plan for change
Data is constantly changing, and your data governance model needs to be flexible to adapt.
"It's tempting to think of governance as something you can 'set and forget' -- you do the upfront work to define it once, and reap the benefits thereafter," said Benn Stancil, co-founder and president of Mode, an analytics platform. "Unfortunately, that's not realistic."
Data models change every time your business changes, and a strategy of data governance for self-service analytics must account for that.