Top 12 business intelligence challenges to manage
BI teams face various technical and project management challenges on deployments. Here are the top BI challenges, with advice on how to address them.
As businesses of all sizes rush to make sense of the growing volumes of data they're collecting, they face a variety of business intelligence challenges that complicate efforts to make BI processes productive, effective and useful.
These challenges are shaped by multiple factors, including diverse data infrastructures, data management issues, new types of BI capabilities and varying levels of data literacy in the workforce. On the one hand, BI teams must ensure that proper data governance and security protections are put in place; on the other, they need to demonstrate how BI can benefit workers, including those who are less data literate.
Another set of BI challenges centers around changes in the ways that business intelligence tools are used in organizations to guide business decisions.
"Traditional BI typically involves curated data and applications driven by IT," said Porter Thorndike, principal product manager at Cloud Software Group's IBI division. The traditional approach provides information to business users via BI dashboards, reports and portals with well-defined workflows, Thorndike said. In contrast, modern BI initiatives are often driven by business units using self-service BI tools to hunt for insights and create data visualizations, dashboards and reports themselves.
This article is part of
What is business intelligence (BI)? A detailed guide
In many cases, the challenges start with getting approval and funding for a business intelligence program and developing a solid BI strategy that meets business requirements and can deliver the promised ROI. In addition to conventional querying and reporting, BI strategies often need to incorporate mobile BI, real-time BI and analytics, augmented analytics and other specialized applications. That further increases the deployment and management challenges.
As they address all the issues, BI and data managers need to strike the right balance between self-service agility and good governance. Faster time to insight can provide a competitive advantage. But that needs to be balanced against data security and privacy concerns and the risk that business users might perpetuate inaccurate findings. As Thorndike put it, "Is the speed at which those insights are generated worth acting upon knowing that some of the insights could be faulty?"
Here's a more detailed look at the top business intelligence challenges for enterprises, plus advice from BI practitioners on how to avoid and overcome them.
1. Integrating data from different source systems
The growth in data sources means many organizations need to pull together data for analysis from a variety of databases, big data systems and business applications, both on-premises and in the cloud. The most common way is to deploy a data warehouse as a central location for BI data. Other approaches are more agile -- for example, using data virtualization software or BI tools themselves to integrate data without loading it into a data warehouse. But that's a complicated process.
Other types of data integration challenges require technical tradeoffs. For example, Sameer Dixit, senior vice president of engineering for data, analytics and AI/machine learning at consulting services firm Persistent Systems, said his team ran into problems refreshing Microsoft Power BI reports on the fly from a customer's Google BigQuery cloud data warehouse. The team found it could refresh the reports in memory instead, but Dixit said performance was constrained by the hardware being used.
Dixit's team also faced challenges in harmonizing different file formats used by another client's source systems. The team explored creating a separate extract, transform and load (ETL) mapping for each file, but that would have taken too much time, he said. Instead, the team wrote a Java application to bring all the files into a common format that could be processed efficiently in a single ETL job.
2. Data quality issues
BI applications are only as accurate as the data they're built on. Users need to have access to high-quality data before beginning any BI projects, said Soumya Bijjal, vice president of product management at Aiven, an open source data infrastructure platform provider.
But, Bijjal added, in the rush to aggregate data in BI systems, many organizations neglect data quality or think they can just fix errors after BI teams or users collect data for analysis. "Data quality is one of the most crucial aspects of BI that is often overlooked," she said.
The root cause of poor data quality can be a lack of understanding about the importance of proper data management among users. Bijjal recommended that, when organizations deploy BI tools, they create a data collection process that involves everyone in thinking about how to ensure data is accurate. A data management strategy that provides a solid foundation to track the entire data lifecycle -- from raw data to prepared BI data sets -- is also a must.
3. Data silos with inconsistent information
Siloed systems are another common business intelligence challenge. Data completeness is a necessity for effective BI, but Bijjal said it's difficult for BI tools to access siloed data with varying permission levels and security settings. BI and data management teams must break down data silos and harmonize the data in them to have the desired impact on business decision-making, she added.
Many organizations struggle with that due to a lack of internal data standards in different departments and business units.
"This is one of the hardest things to overcome because a lot of definitional work needs to be done spanning business functions," said Cameron Cross, partner, technology and experience at consulting firm West Monroe Partners. For one client project, his team had to assemble senior business leaders in a room and get them to agree on fundamental data definitions, such as what constitutes a pair of eyeglasses.
Inconsistent data in silos can lead to multiple versions of the truth, said Garegin Ordyan, vice president of data and analytics at data integration software vendor Fivetran. Business users then see different results for KPIs and other business metrics that are labeled similarly in separate systems. To avoid that, Ordyan recommended starting with a well-defined data modeling layer and clear definitions for each KPI and metric.
4. Managing the use of self-service BI tools
Uncontrolled self-service BI deployments in different business units can lead to a chaotic data environment with silos and conflicting analytics results that create confusion in the minds of business executives and other decision-makers.
Thorndike said most modern BI tools have a data and security architecture that provides a protected place for user-generated analytics to be stored and shared. He recommended that BI and data management teams curate data sets in data warehouses or other analytics repositories upfront to help avoid inconsistences.
"We've found that the key to enriching the self-service experience is to expose these tools to curated data and content, which users can leverage to create much better data flows and mashups," Thorndike said.
5. Creating solid data models for BI applications
Ineffective self-service BI and analytics initiatives often stem from insufficient data modeling, said Tracy Colameco, vice president of analytics and information management at Cervello, a subsidiary of consulting firm Kearney. This can lead to inefficiencies when data isn't structured in a way that empowers users to access and use it easily. BI and data engineering teams "often lack the necessary business context to model data effectively, resulting in data that is not readily usable or actionable for end users," Colameco said.
She recommends BI teams create a business translator role to help bridge the gap between business concepts and technical execution. Unlike a business analyst, who primarily gathers requirements, a business translator deeply understands business needs and technical frameworks. This can help ensure data is modeled based on the user's understanding and experience.
Another good practice is to look at how to use generative AI (GenAI) tools to translate logical data models into physical ones. This can make it easier to optimize the data structure for source systems and improve how it's provisioned for self-service access in BI systems.
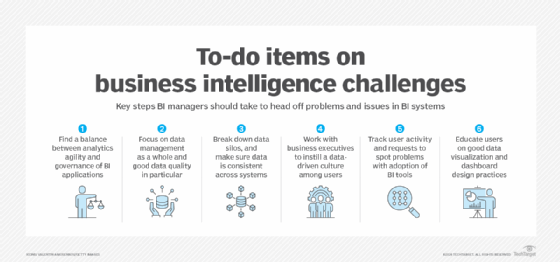
6. Bad data visualization and dashboard design practices
Data visualizations often go wrong, making it hard to decipher the information they're trying to illustrate. Similarly, a BI dashboard or report is only valuable if it's easy for users to navigate and understand the data being presented. But organizations often focus on getting BI data and the analytics process right without thinking about design and UX.
Dixit said BI managers should involve a UX designer to develop an intelligible look for dashboards and reports with a visual interface that isn't cluttered. BI teams should also promote good data visualization design practices, particularly in self-service BI environments. Those steps are especially important for mobile BI applications on smartphones and tablets with small screen sizes.
7. Justifying new BI investments
Growing or improving a BI program requires making a case that the additional investment will improve the company's bottom line. But this often comes as an afterthought for BI teams.
As a result, many teams struggle to effectively measure the ROI of their initiatives, said Sandy Estrada, vice president of client solutions at Cervello. This can arise from a lack of clearly defined KPIs at the outset of BI projects, insufficient resources for tracking metrics or not focusing on ROI in the first place.
One of the crucial steps to measure ROI is to agree with business stakeholders on KPIs and other metrics for each BI project before it's launched. This ensures alignment on the project's goals and success criteria. It also provides benchmarks for tracking progress and evaluating the overall business impact.
8. Connecting BI insights to business actions
When developing BI applications, where data is presented is almost as important as how it's presented, said Karel Callens, CEO and co-founder at Luzmo, a data analytics platform provider. Ideally, you want to surface data in the operational applications where business activities take place. This can help BI teams create an iterative loop between data analytics, business actions and outcomes.
For example, embedded analytics tools enable BI to be integrated into business systems rather than being deployed as a separate tab, page or tool. In some cases, a single metric delivered in the right context can significantly increase BI's business impact. In other cases, a user might want to consider a range of metrics and analytics in uses such as data exploration.
Another challenge lies in balancing efforts to put everything business users want in one place to improve accessibility without stripping away important details for data-literate users. GenAI can help democratize access through natural language query prompts. It is also critical to make it easy to customize BI insights for different types of users.
9. Delivering the right data to decision-makers
A related challenge is customizing BI insights for different users, Callens said. Doing so can help BI teams deliver more personalized user experiences. "Have BI appear when you need it with the insights that are relevant to you at that moment in time, given your role," he said.
But the ever-increasing amount of data generated and collected by organizations complicates efforts on what insights to share and in what format, depending on the BI use case. Superfluous data can be counterproductive in decision-making. That makes presenting only relevant insights a must to enable effective data-driven decisions. "Oversaturating users with information can happen quite quickly, leading to decision paralysis," Callens said.
10. Combining different BI and analytics technologies
"There's still quite a wide range of technologies used for business intelligence efforts," said Michael Berthold, CEO at data science platform vendor Knime. In addition to traditional data warehouses, for example, BI data is now sometimes stored in data lakes or newer data lakehouses that combine elements of the other two platforms. Also, AI tools are increasingly being incorporated into the BI process.
This leads to difficulties in accessing data, integrating different tools and keeping up with how to use the latest technologies. It can also result in siloed knowledge and skills gaps across an organization, plus other potential issues. "This is not a new problem, but it's further complicated by the integration of AI models, which more often than not run on someone else's cloud, opening up organizations to data leakage risks and exploding consumption costs," Berthold said.
One option for BI teams to consider: Low-code development tools can help abstract away the complexity of coding languages and other data technology to simplify the adoption and use of new tools.
11. A lack of trust in AI tools deployed for BI uses
Various types of AI are now used as part of business intelligence platforms. At the same time, many organizations are early in establishing effective governance for their AI initiatives. "The net effect is lack of trust in data usage, insufficient confidence in AI-generated insights and inadequate tracking and monitoring," said Briana Ullman, global AI product manager at analytics vendor SAS. As a result, integrating AI into core business processes, including BI applications, remains a significant challenge.
This can show up as BI practitioners struggle to successfully implement AI tools and corporate executives grapple with how to get their AI strategy right. The latter is crucial, though: Ullman recommends that organizations adopt a comprehensive AI strategy to prioritize AI governance, mitigate risk or reputational harm, and safeguard customer trust.
12. Regulatory compliance on data security and privacy
Regulations that organizations need to comply with as part of BI initiatives can vary widely depending on the industry, geographic region and specific technology being used. This includes data protection laws like GDPR or CCPA, industry-specific regulations like HIPAA for healthcare, and general standards for data security and privacy, such as Federal Risk and Authorization Management Program and the Cybersecurity Executive Order.
"For companies operating under these strict regulatory and compliance frameworks, keeping up with the latest changes and ensuring compliance can be daunting," Ullman said. Incorrect configuration settings and inadequate security practices in BI applications can lead to vulnerabilities, data breaches and improper use of sensitive information.
As a result, BI programs need to include strong compliance processes. Ullman recommends implementing continuous usage monitoring and auditing, ensuring data governance and data privacy, and maintaining required legal and compliance expertise. In addition, organizations should ensure that BI software vendors provide regular reports, certifications and audit trails to confirm ongoing compliance of their tools.
More tips on overcoming business intelligence challenges
BI managers should also create a process for maintaining and updating metrics, data models and dashboards, Ordyan said. That includes routinely looking at what's being used and what isn't and removing items that are no longer needed. "If you don't delete the things that aren't being used, you're going to find yourself in a situation where you have too much to maintain for no good reason," he warned.
An effective BI strategy also needs to meet different requirements across an organization.
"Low BI tool adoption frequently results from an ivory tower approach, where organizations attempt to implement a single, one-size-fits-all solution for all users," Estrada said. This approach fails to account for the diverse information needs and varying levels of data literacy within the organization.
Estrada recommends considering diverse tools and approaches to meet users where they are. This starts by understanding how they think about and use data in their current roles and identifying their data literacy levels and the support they require. It's also important to identify and support their preferred tools and formats, whether they're dashboards, spreadsheets or reports.
Other general advice for successfully managing challenges includes ensuring that your underlying BI architecture can scale and accommodate new tools as needed and that different users have the right tools for their skill levels. For example, augmented analytics features in BI software can help users find relevant data, prepare it for analysis, run natural language queries and create data visualizations.
Editor's note: This article was updated in November 2024 to improve the reader experience.
George Lawton is a journalist based in London. Over the last 30 years, he has written more than 3,000 stories about computers, communications, knowledge management, business, health and other areas that interest him.