How to create a winning AI strategy for your business
To deliver real business value, AI projects must be aligned with organizational goals. Here is a 10-step program for developing an effective AI strategy, plus sample templates.
Enterprise executives increasingly embrace artificial intelligence, yet their ability to harness AI remains elusive in many cases.
Consider some figures from the 2024 report "Scaling AI Initiatives Responsibly," published by research firm IDC. It found that organizations with mature AI practices -- dubbed AI Masters -- still have a 13% failure rate on average. Those considered AI Emergents have an even higher failure rate, at 20%.
There are multiple reasons for those failure rates, according to the report and numerous executive advisors. Reasons range from poor data quality to cultural aversion to AI use.
To avoid or minimize failure rates, executives need to be more mindful and intentional -- in other words, more strategic -- about where, when and how they use AI in their organizations.
"Too many think they just need to implement the right technology, then the benefits will come along. But to succeed with AI, they need to do a lot of work in advance," said Nick Kramer, leader of Applied Solutions at SSA & Company, a global consulting firm advising companies on strategic execution.
This article is part of
What is enterprise AI? A complete guide for businesses
Kramer said execs also need to industrialize AI, that is, incorporate it into products, services, roadmaps, workflows and workplace culture.
What is an AI strategy and why is it important?
An AI strategy pulls together the various components and competencies required to accomplish business objectives related to the use of AI technologies. These include the following:
- The ability to implement successful AI deployments of all kinds, including generative AI.
- The ability to enable those deployments to produce ongoing benefits.
- The ability to manage AI within the enterprise to minimize risks and maximize returns on investment.
A well-crafted strategy informs what actions the organization, its leaders and other employees need to take to ensure their uses of AI are effective, efficient and optimal.
Research firm Gartner has identified four pillars of a successful AI strategy: vision, value, adoption and risk. Others list additional and different pillars, such as technology, data, data governance and workforce.
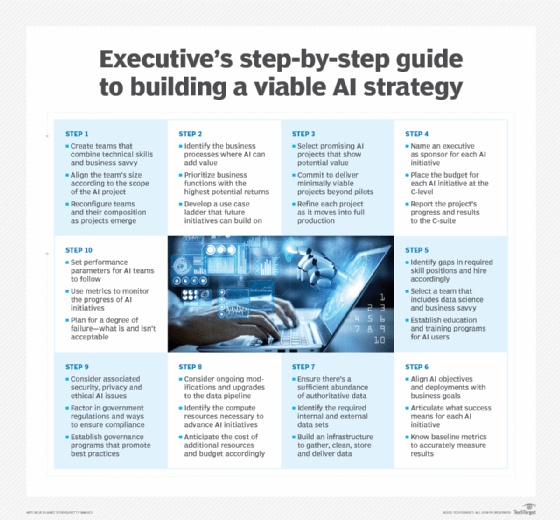
10 components of a successful AI strategy
To ensure they have both the foundation and the pathway to succeed, experts said business and IT leaders should devise an AI strategy that addresses the following 10 components.
1. Readiness assessment
Although many organizations have been using classical machine learning for a decade or more to handle repetitive tasks and support automation, AI -- and particularly generative AI (GenAI) -- remain relatively new technologies.
As such, many organizations do not have the necessary technology or knowledge to identify and seize upon the opportunities that come with AI. Nor do they have the necessary skills and know-how to identify and mitigate the risks.
"So first assess organizational AI readiness and capabilities," said Manjeet Rege, professor and chair of the Department of Software Engineering and Data Science at the University of St. Thomas and director of its Center for Applied Artificial Intelligence.
"Evaluate the organization's current state before embarking on any further AI initiatives. Look at your existing data infrastructure, technical capabilities, employee skills, and the strengths and weaknesses around your existing AI implementations," Rege added. "This will identify areas that need improvement and inform the development of a plan to address [deficits]."
2. A strong data program
Data has become a key asset for most organizations, and access to enough high-quality data is essential for AI success. Yet many organizations still struggle with the state of their data.
The "2024 Global AI Trends Report" from data platform maker Weka found that 35% of the 1,400 AI practitioners it surveyed cited storage and data management as the primary infrastructure issues hindering AI deployments.
The IDC report similarly found that both AI Masters and AI Emergents cited data-related issues, such as data access limitations, change management, data expiration and insufficient data, as reasons for failures with their AI initiatives.
A strong data governance program is thus essential for a successful AI strategy, said Steve Ross, director of cybersecurity, Americas at S-RM, a global corporate intelligence and cybersecurity consultancy.
"Review policies and procedures for data, and map out what needs to be done to improve," he said, adding that a robust data program should address security, privacy and quality standards, among other considerations.
3. An AI culture
Executives who see AI as only a technical revolution do so at their own peril, warned Kramer, noting that it's as much -- if not more so -- about people. People are the ones to identify opportunities, devise risk mitigations, set guardrails and establish policies that will govern the use of AI.
Therefore, organizations must ensure they know what knowledge and skills they need in what roles to enable, adopt and embrace AI, he said. They also need to make sure they have in place a solid change management plan for AI products and services.
"AI is more about the people than any other technology I've seen since the last widescale industrial automation in the 1970s and '80s, so make sure you put people at the center of the AI revolution," he added.
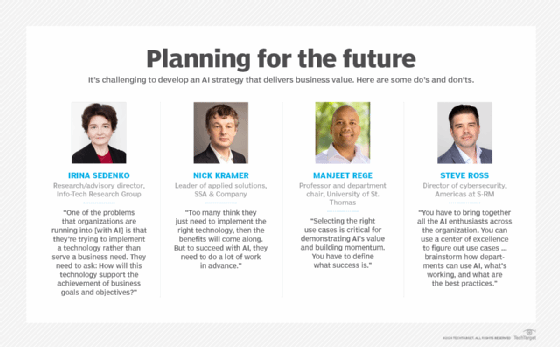
4. Alignment with business objectives
Despite frequent warnings in IT circles not to pursue technology for technology's sake, that is happening in many organizations today, said Irina Sedenko, research and advisory director in the data and analytics practice at Info-Tech Research Group.
"One of the problems that organizations are running into is that they're trying to implement a technology [i.e., AI] rather than serve a business need," Sedenko said.
Instead, executives -- CIOs and AI directors working alongside functional leaders -- need to link their AI strategy with the overall business strategy.
"They need to ask: How will this technology support the achievement of business goals and objectives," Sedenko said.
To do that, execs need to not only have a clear business strategy, they also need to understand how AI is changing their specific industry and how AI will present new opportunities and challenges to their sector and their individual business.
"The business need should always come first," she added. "It's not saying, 'Let's build a chatbot,' but rather asking 'What are we trying to achieve?' and knowing when AI, or AI with another technology such as [robotic process automation], or AI with a business process redesign may be the solution -- and when it is not."
5. A mechanism for prioritizing AI initiatives
"The state of AI in early 2024" report from management consulting firm McKinsey & Company found that AI adoption spiked over the prior year, driven in large part by adoption of GenAI. According to the report, the percentage of organizations that had adopted AI in at least one business function jumped from 55% in 2023 to 72% in 2024.
Moreover, McKinsey's research showed that a growing number of organizations have adopted AI in multiple business functions, with half of its survey respondents saying their organizations have adopted AI in two or more business functions in 2024 -- up from less than a third of respondents in 2023.
Organizations need to not only tie their AI initiatives to business goals, they also should have a way to prioritize which projects to pursue first, Rege said.
"Selecting the right use cases is critical for demonstrating AI's value and building momentum," he said, advising CIOs and their business unit counterparts to identify those that lead to short- and medium-term benefits and prioritize those that are expected to yield the biggest bang for the buck.
6. Metrics to determine, measure success
Execs also need to establish metrics, key performance indicators (KPIs) and other yardsticks to measure the value that AI initiatives are producing, experts said.
As Rege explained: "You have to define what success is."
Many are not doing this yet, said Lee Davidson, who as chief data and analytics officer for investment research company Morningstar leads the firm's AI strategy.
"Strategy officers and CFOs are struggling to quantify the benefits and how to measure value," he added.
Davidson acknowledged that this is a challenging step, given that "we don't know what the end state is going to be [for some AI projects]."
Consequently, executives might need to be flexible on some aspects -- such as the timeline for seeing a target return on investment, he said. That, though, does not mean executives should forgo all measures. AI project leaders still need to be held accountable for producing value.
7. A pathway for executing
Prioritization should be the precursor to execution, and as such, organizations need to create a structure to funnel promising projects through to development -- just as they have done for other tech-driven initiatives, Kramer said.
"That's when you get into how you start to deliver on vision and alignment," he explained, noting that enterprise leaders should ensure that their culture and incentives support innovation and experimentation rather than a take-no-risks approach.
Furthermore, organizations should have a rigorous intake and evaluation framework "to make sure the technology is right for the problem," Kramer said. Similarly, organizations should have a structure for converting ideas into controlled experiments that, if successful, get turned into minimum viable products and eventually full-fledged products "where the right people are brought in at the right time."
8. A plan for 'industrializing' AI initiatives
Organizations should be considering how they'll scale their AI capabilities and treat AI similar to other tech products and services that are subject to iterative improvements -- a key component with AI models, as they require continuous modeling, retraining and refinement as business needs change and new data is available.
"It's about industrializing," Kramer said, explaining that this component involves "hardening security, understanding what technology architecture is required to scale, having change management in place, and incorporating [AI] into DevOps so you're looking at AI with a product mentality."
Others said this step should also include plans for determining whether and when to build or buy; similarly, organizations need guidelines for exploring and evaluating AI vendors and the products, services, technologies and talent needed to support the organization's AI roadmap.
9. An AI center of excellence
AI initiatives require experts from multiple areas, such as IT, data teams, business units and AI specialists. This creates a strong incentive for organizations to bring them all together in a center of excellence, Sedenko said.
The size and number of the teams required depends on the organization and the scope of its AI initiatives. Executives should adjust the number of teams and their composition as projects emerge.
"You have to bring together all the AI enthusiasts across the organization," Sedenko added. "You can use a center of excellence to figure out use cases, different applications of AI, brainstorm how departments can use AI, what's working, and what are the best practices."
10. Governance
The McKinsey report, which gleaned responses from nearly 1,400 global executives, asked respondents about the risks they see with AI. They identified the following, from most often to least cited:
- Inaccurate results.
- Intellectual property infringement.
- Cybersecurity.
- Personal/individual privacy.
- Regulatory compliance.
- Explainability.
- Equity and fairness.
- Workforce labor displacement.
- Organizational reputation.
- National security.
- Environmental impact.
- Political stability.
- Physical safety.
That's a significant list -- and it's not even all the possible risks. Other reports have found that IT and business leaders are also highly concerned about the responsible and ethical use of AI.
Given that lengthy list, and the complexity and significance of each item, organizations must have an AI governance practice in place, experts said.
"You need expertise to understand regulatory concerns and risks and downstream impacts with AI," Ross said, adding that good governance should have, among other items, a mechanism to identify the risks most concerning to the organization and an audit capability to ensure AI outputs are accurate and meet the standards set by the organization.
Templates for developing an AI strategy
Resources for AI templates and guidance include the following.
Info-Tech Research Group's Build your AI Strategic Roadmap offers a template "to create an IT-led, business-aligned AI strategy for your organization," with sections on governing AI effectively, building an AI-powered enterprise, and developing AI execution capabilities.
The Generative AI Strategy Template from AI consulting company Prolego supports a multiyear organizational transformation and the shift to being AI-empowered. This template, specifically designed for developing a GenAI strategy, revolves around five dimensions: capabilities, infrastructure, talent, governance and tools.
Bernard Marr, a futurist and thought leader in the fields of business and technology, offers AI guidance on his in his website offering: How to Develop Your Artificial Intelligence (AI) Strategy – With Handy Template.
Cascade, which makes a strategy execution platform, also has a downloadable AI strategy template. This template has three focus areas, with each area having its own objectives, projects and KPIs.
Another free downloadable AI strategy template comes from Adeva, a global talent network specializing in tech staffing; this template, How to Create an Effective AI Strategy for Your Business, is designed to help organizations outline their AI strategy into a coherent plan that aligns to business objectives.
WSI World, a global network of digital marketing agencies, also offers a free downloadable template: Create Your Own AI Principles. This template guides organizations with a set of principles to help manage AI-related risks as well as ethical and responsible practices while also aiming for maximum AI benefits.
Mary K. Pratt is an award-winning freelance journalist with a focus on covering enterprise IT and cybersecurity management.