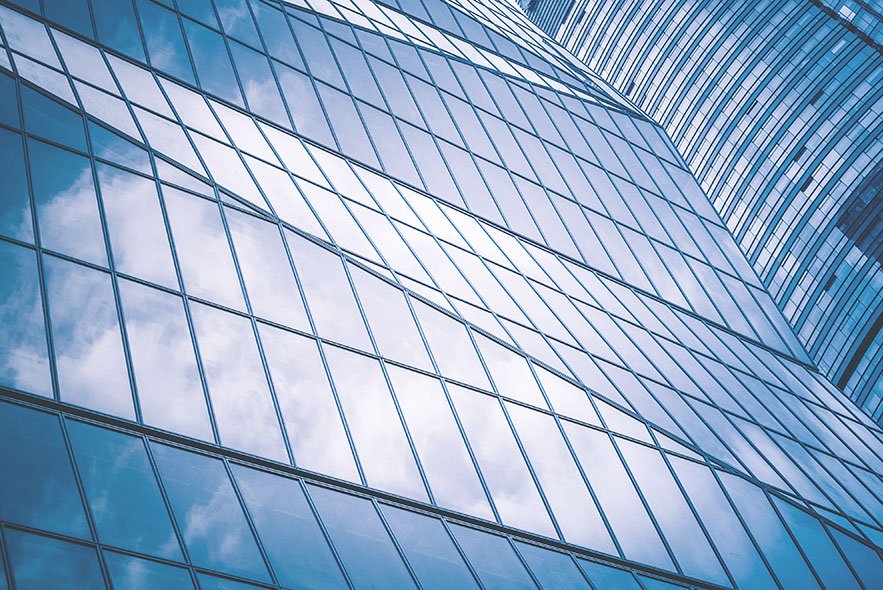
explainable AI
What is explainable AI (XAI)?
Explainable AI (XAI) is artificial intelligence (AI) that's programmed to describe its purpose, rationale and decision-making process in a way that the average person can understand. XAI helps human users understand the reasoning behind AI and machine learning (ML) algorithms to increase their trust.
Explainable artificial intelligence is often discussed in relation to deep learning and plays an important role in the FAT -- fairness, accountability and transparency -- ML model. XAI is useful for organizations that want to adopt a responsible approach to the development and implementation of AI models. XAI can help developers understand an AI model's behavior, how an AI reached a specific output, and in finding potential issues such as AI biases.
ML models are typically structured in a white box or black box format. White box models provide more visibility and understandable results to users and developers, whereas the AI decisions or predictions black box models make are extremely hard to explain, even for AI developers.
XAI provides general data focused on how an AI program makes a decision by disclosing the following:
- The program's strengths and weaknesses.
- The specific criteria the program uses to arrive at a decision.
- Why a program makes a particular decision as opposed to alternatives.
- The level of trust appropriate for various types of decisions.
- What types of errors to which the program is prone.
- How errors can be corrected.
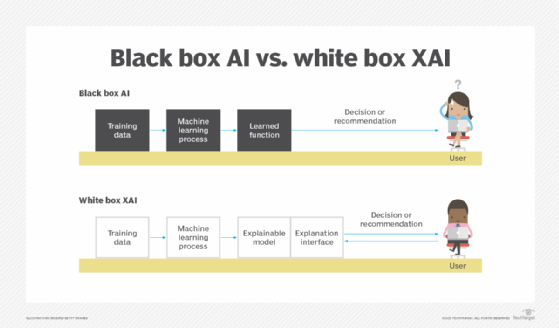
The importance of explainable AI
An important goal of XAI is to provide algorithmic accountability. AI systems used to be predominantly black boxes. Even if the inputs and outputs are known, the algorithms used to arrive at a decision are often proprietary or aren't easily understood.
This article is part of
A guide to artificial intelligence in the enterprise
With AI services being integrated into fields such as health IT or mortgage lending, it's important to ensure that the decisions made by AI systems are sound and trustable. For example, due to AI bias, an individual could be denied a mortgage. Likewise, an AI-based system can't accurately help medical professionals make objective decisions if the data set it was trained on isn't diverse enough. Without a diverse enough data set, the AI model might do an inadequate job of detecting illnesses in patients of different races, genders or geographies. Without having proper insight into how the AI is making its decisions, it can be difficult to monitor, detect and manage these types of issues.
As AI becomes increasingly prevalent, it's more important than ever to disclose how bias and the question of trust are being addressed.
How does explainable AI work?
From the outset, an organization can create an AI governance committee with the goal of guiding its development teams in the creation of their AI model. This committee's job should be to set standards for AI explainability. Doing this from the start makes explainability one of the key principles of the organization's responsible AI guidelines.
At a basic level, the data used in training is also important for creating an explainable AI model. When designing an AI model, developers should pay close attention to the training data to ensure it doesn't have any bias. If the data is biased, then developers should explore what can be done to mitigate it. In addition, any irrelevant data should be excluded from training.
Creating an explainable AI model might look different depending on the AI system. For example, some AIs might be designed to give an explanation along with each given output stating from where the information came. It's also important to design a model that uses explainable algorithms and produces explainable predictions. Designing an explainable algorithm means that the individual layers that make up the model should be clear in how they lead to an output. Likewise, producing an explainable prediction means that the features of a model that were used in a prediction or output should be clearly defined.
Examples of explainable AI
XAI can be found in the following industries:
- Healthcare. Explainable AI systems that aid in patient diagnosis can help build trust between doctor and system, as the doctor can understand where and how the AI system reaches a diagnosis.
- Financial. XAI is used to approve or deny financial claims such as loans or mortgage applications, as well as to detect financial fraud.
- Military. Military AI-based systems need to be explainable to build trust between service people and any AI-enabled equipment they rely on for safety.
- Autonomous vehicles. XAI is used in autonomous vehicles to explain driving-based decisions, especially those that revolve around safety. If a passenger can understand how and why the vehicle is making its driving decisions, they can feel safer knowing what scenarios the vehicle can or can't handle well.
XAI is especially important in areas where someone's life could be immediately affected. For example, in healthcare, AI could be used to identify patient fractures based on X-rays. But even after an initial investment in an AI tool, doctors and nurses might still not be ready to adopt the AI if they don't trust the system or know how it arrives at a patient diagnosis. An explainable system gives healthcare providers the chance to review the diagnosis and to use that information to inform their own prognosis.
Likewise, for military operations, the Defense Advanced Research Projects Agency, or DARPA, is developing XAI in its third-wave AI systems.
Benefits of explainable AI
XAI provides overall more accountability and transparency in AI systems. Its benefits include the following:
- Makes AI more trustworthy. Individuals might be reluctant to trust an AI-based system, as they can't tell how it reaches a particular conclusion. XAI is designed to give understandable explanations of its decisions to end users.
- Improves the overall AI system. With added transparency, developers can more easily identify and fix issues.
- Provides insight against adversarial attacks. Adversarial ML attacks attempt to fool or misguide a model into making incorrect decisions using maliciously designed data inputs. An adversarial attack against an XAI system would show irregular explanations for its decisions, revealing the attack.
- Offers safeguards against AI bias. The goal of XAI is to explain attributes and decision processes in ML algorithms. This helps identify unfair outcomes due to the lack of quality in training data or developer biases.
Limitations of explainable AI
XAI also has the following limitations:
- Oversimplification. Compared to other transparency methods, an XAI system might oversimplify and misrepresent a complicated system, leading to a debate about designing AI systems with more interpretable models, or models that can more accurately associate causes to effects.
- Lower model performance. XAI systems typically have lower performance when compared to black box models.
- Difficulty in training. Creating an AI system that also explains its reasoning is more complicated to achieve when compared to black box models.
- Lack of privacy. If an XAI system works with confidential data, that data could be exposed because of XAI's transparent nature.
- Decrease in understanding and trust. Although XAI should lead to an increased trust in AI, some users might still not trust the system, even with an understandable explanation behind its decisions.
The history of explainable AI
The creation of explainable AI systems is now more important than ever because of the effects that it can have on real people. The explainability of AI has been an important aspect of creating an AI system since at least the 1970s. In 1972, the symbolic reasoning system MYCIN was developed for explaining the reasoning for diagnostic-related purposes, such as treating blood infections.
Throughout the 1980s and into the 1990s, truth maintenance systems (TMSes) were developed to extend AI reasoning abilities. As an example, these were used in rule- and logic-based inference systems. A TMS tracks AI reasonings and conclusions by tracing the reasoning an AI makes through rule operations and logical inferences. This process generates an explanation for each AI reasoning.
From the 2010s onward, explainable AI systems have been used more publicly. Some AI systems began exhibiting racial and other sorts of biases, leading to an increased focus on developing ways to detect bias in AI.
Explainable AI vs. interpretable AI
Explainability and interpretability are two concepts in AI that are sometimes used interchangeably. However, there's a slight difference in meaning.
While explainability refers to the ability to explain the AI decision-making process in a way that's understandable to the user, interpretability refers to the predictability of a model's outputs based on its inputs. Interpretability is typically used to understand an AI model's inner workings. Interpretability is important if an organization needs a model with high levels of transparency and must understand exactly how the model generates its outcomes. However, this can cause a model to perform slower. If performance is a more valued factor, then an organization can instead focus on explainability.
Explainable AI vs. responsible AI
Explainable AI and responsible AI are both important concepts when designing a transparent and trustable AI system.
Responsible AI is an approach to developing and deploying AI from an ethical and legal point of view. Its goal is to employ AI in a safe, trustworthy and ethical way. AI interpretability and explainability are both important aspects of developing a responsible AI.
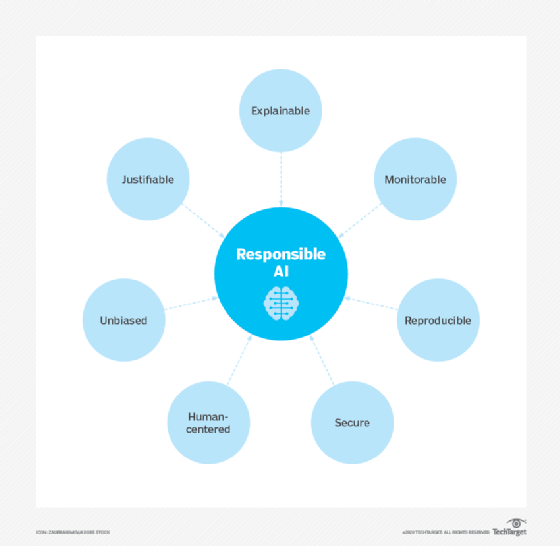
Principles that make up responsible AI include the following:
- Accountability and transparency.
- Explainability and interpretability.
- Fairness.
- Privacy.
- Security and resiliency.
- Validity and reliability.
- Safety.
Transparency and explainability continue to be important concepts in AI. Learn more about why transparency in AI matters for organizations.