8 AI and machine learning trends to watch in 2025
AI agents, multimodal models, an emphasis on real-world results -- learn about the top AI and machine learning trends and what they mean for businesses in 2025.
Generative AI is at a crossroads. It's now more than two years since ChatGPT's launch, and the initial optimism about AI's potential is decidedly tempered by an awareness of its limitations and costs.
The 2025 AI landscape reflects that complexity. While excitement still abounds -- particularly for emerging areas, like agentic AI and multimodal models -- it's also poised to be a year of growing pains.
Companies are increasingly looking for proven results from generative AI, rather than early-stage prototypes. That's no easy feat for a technology that's often expensive, error-prone and vulnerable to misuse. And regulators will need to balance innovation and safety, while keeping up with a fast-moving tech environment.
Here are eight of the top AI trends to prepare for in 2025.
1. Hype gives way to more pragmatic approaches
Since 2022, there's been an explosion of interest and innovation in generative AI, but actual adoption remains inconsistent. Companies often struggle to move generative AI projects, whether internal productivity tools or customer-facing applications, from pilot to production.
This article is part of
What is enterprise AI? A complete guide for businesses
Although many businesses have explored generative AI through proofs of concept, fewer have fully integrated it into their operations. In a September 2024 research report, Informa TechTarget's Enterprise Strategy Group found that, although over 90% of organizations had increased their generative AI use over the previous year, only 8% considered their initiatives mature.
"The most surprising thing for me [in 2024] is actually the lack of adoption that we're seeing," said Jen Stave, launch director for the Digital Data Design Institute at Harvard University. "When you look across businesses, companies are investing in AI. They're building their own custom tools. They're buying off-the-shelf enterprise versions of the large language models (LLMs). But there really hasn't been this groundswell of adoption within companies."
One reason for this is AI's uneven impact across roles and job functions. Organizations are discovering what Stave termed the "jagged technological frontier," where AI enhances productivity for some tasks or employees, while diminishing it for others. A junior analyst, for example, might significantly increase their output by using a tool that only bogs down a more experienced counterpart.
"Managers don't know where that line is, and employees don't know where that line is," Stave said. "So, there's a lot of uncertainty and experimentation."
Despite the sky-high levels of generative AI hype, the reality of slow adoption is hardly a surprise to anyone with experience in enterprise tech. In 2025, expect businesses to push harder for measurable outcomes from generative AI: reduced costs, demonstrable ROI and efficiency gains.
2. Generative AI moves beyond chatbots
When most laypeople hear the term generative AI, they think of tools like ChatGPT and Claude powered by LLMs. Early explorations from businesses, too, have tended to involve incorporating LLMs into products and services via chat interfaces. But, as the technology matures, AI developers, end users and business customers alike are looking beyond chatbots.
"People need to think more creatively about how to use these base tools and not just try to plop a chat window into everything," said Eric Sydell, founder and CEO of Vero AI, an AI and analytics platform.
This transition aligns with a broader trend: building software atop LLMs rather than deploying chatbots as standalone tools. Moving from chatbot interfaces to applications that use LLMs on the back end to summarize or parse unstructured data can help mitigate some of the issues that make generative AI difficult to scale.
"[A chatbot] can help an individual be more effective ... but it's very one on one," Sydell said. "So, how do you scale that in an enterprise-grade way?"
Heading into 2025, some areas of AI development are starting to move away from text-based interfaces entirely. Increasingly, the future of AI looks to center around multimodal models, like OpenAI's text-to-video Sora and ElevenLabs' AI voice generator, which can handle nontext data types, such as audio, video and images.
"AI has become synonymous with large language models, but that's just one type of AI," Stave said. "It's this multimodal approach to AI [where] we're going to start seeing some major technological advancements."
Robotics is another avenue for developing AI that goes beyond textual conversations -- in this case, to interact with the physical world. Stave anticipates that foundation models for robotics could be even more transformative than the arrival of generative AI.
"Think about all of the different ways we interact with the physical world," she said. "I mean, the applications are just infinite."
3. AI agents are the next frontier
The second half of 2024 has seen growing interest in agentic AI models capable of independent action. Tools like Salesforce's Agentforce are designed to autonomously handle tasks for business users, managing workflows and taking care of routine actions, like scheduling and data analysis.
Agentic AI is in its early stages. Human direction and oversight remain critical, and the scope of actions that can be taken is usually narrowly defined. But, even with those limitations, AI agents are attractive for a wide range of sectors.
Autonomous functionality isn't totally new, of course; by now, it's a well-established cornerstone of enterprise software. The difference with AI agents lies in their adaptability: Unlike simple automation software, agents can adapt to new information in real time, respond to unexpected obstacles and make independent decisions.
Yet, that same independence also entails new risks. Grace Yee, senior director of ethical innovation at Adobe, warned of "the harm that can come ... as agents can start, in some cases, acting upon your behalf to help with scheduling or do other tasks." Generative AI tools are notoriously prone to hallucinations, or generating false information -- what happens if an autonomous agent makes similar mistakes with immediate, real-world consequences?
Sydell cited similar concerns, noting that some use cases will raise more ethical issues than others. "When you start to get into high-risk applications -- things that have the potential to harm or help individuals -- the standards have to be way higher," he said.
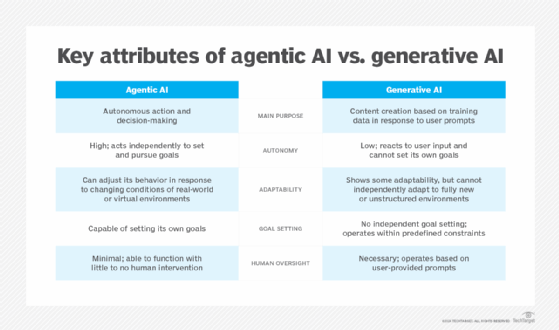
4. Generative AI models become commodities
The generative AI landscape is evolving rapidly, with foundation models seemingly now a dime a dozen. As 2025 begins, the competitive edge is moving away from which company has the
best model to which businesses excel at fine-tuning pretrained models or developing specialized tools to layer on top of them.
In a recent newsletter, analyst Benedict Evans compared the boom in generative AI models to the PC industry of the late 1980s and 1990s. In that era, performance comparisons focused on incremental improvements in specs like CPU speed or memory, similar to how today's generative AI models are evaluated on niche technical benchmarks.
Over time, however, these distinctions faded as the market reached a good-enough baseline, with differentiation shifting to factors such as cost, UX and ease of integration. Foundation models seem to be on a similar trajectory: As performance converges, advanced models are becoming more or less interchangeable for many use cases.
In a commoditized model landscape, the focus is no longer number of parameters or slightly better performance on a certain benchmark, but instead usability, trust and interoperability with legacy systems. In that environment, AI companies with established ecosystems, user-friendly tools and competitive pricing are likely to take the lead.
5. AI applications and data sets become more domain-specific
Leading AI labs, like OpenAI and Anthropic, claim to be pursuing the ambitious goal of creating artificial general intelligence (AGI), commonly defined as AI that can perform any task a human can. But AGI -- or even the comparatively limited capabilities of today's foundation models -- is far from necessary for most business applications.
For enterprises, interest in narrow, highly customized models started almost as soon as the generative AI hype cycle began. A narrowly tailored business application simply doesn't require the degree of versatility necessary for a consumer-facing chatbot.
"There's a lot of focus on the general-purpose AI models," Yee said. "But I think what is more important is really thinking through: How are we using that technology ... and is that use case a high-risk use case?"
In short, businesses should consider more than what technology is being deployed and instead think more deeply about who will ultimately be using it and how. "Who's the audience?" Yee said. "What's the intended use case? What's the domain it's being used in?"
Although, historically, larger data sets have driven model performance improvements, researchers and practitioners are debating whether this trend can hold. Some have suggested that, for certain tasks and populations, model performance plateaus -- or even worsens -- as algorithms are fed more data.
"The motivation for scraping ever-larger data sets may be based on fundamentally flawed assumptions about model performance," authors Fernando Diaz and Michael Madaio wrote in their paper "Scaling Laws Do Not Scale." "That is, models may not, in fact, continue to improve as the data sets get larger -- at least not for all people or communities impacted by those models."
6. AI literacy becomes essential
Generative AI's ubiquity has made AI literacy an in-demand skill for everyone from executives to developers to everyday employees. That means knowing how to use these tools, assess their outputs and -- perhaps most importantly -- navigate their limitations.
Notably, although AI and machine learning talent remains in demand, developing AI literacy doesn't need to mean learning to code or train models. "You don't necessarily have to be an AI engineer to understand these tools and how to use them and whether to use them," Sydell said. "Experimenting, exploring, using the tools is massively helpful."
Amid the persistent generative AI hype, it can be easy to forget that the technology is still relatively new. Many people either haven't used it at all or don't use it regularly: A recent research paper found that, as of August 2024, less than half of Americans aged 18 to 64 use generative AI, and just over a quarter use it at work.
That's a faster pace of adoption compared with the PC or the internet, as the paper's authors pointed out, but it's still not a majority. There's also a gap between businesses' official stances on generative AI and how real workers are using it in their day-to-day tasks.
"If you look at how many companies say they're using it, it's actually a pretty low share who are formally incorporating it into their operations," David Deming, professor at Harvard University and one of the paper's authors, told The Harvard Gazette. "People are using it informally for a lot of different purposes, to help write emails, using it to look up things, using it to obtain documentation on how to do something."
Stave sees a role for both companies and educational institutions in closing the AI skills gap. "When you look at companies, they understand the on-the-job training that workers need," she said. "They always have because that's where the work takes place."
Universities, in contrast, are increasingly offering skill-based, rather than role-based, education that's available on an ongoing basis and applicable across multiple jobs. "The business landscape is changing so fast. You can't just quit and go back and get a master's and learn everything new," Stave said. "We have to figure out how to modularize the learning and get it out to people in real time."
7. Businesses adjust to an evolving regulatory environment
As 2024 progressed, companies were faced with a fragmented and rapidly changing regulatory landscape. Whereas the EU set new compliance standards with the passage of the AI Act in 2024, the U.S. remains comparatively unregulated -- a trend likely to continue in 2025 under the Trump administration.
"One thing that I think is pretty inadequate right now is legislation [and] regulation around these tools," Sydell said. "It seems like that's not going to happen anytime soon at this point." Stave likewise said she's "not expecting significant regulation from the new administration."
That light-touch approach could promote AI development and innovation, but the lack of accountability also raises concerns about safety and fairness. Yee sees a need for regulation that protects the integrity of online speech, such as giving users access to provenance information about internet content, as well as anti-impersonation laws to protect creators.
To minimize harm without stifling innovation, Yee said she'd like to see regulation that can be responsive to the risk level of a specific AI application. Under a tiered risk framework, she said, "low-risk AI applications can go to market faster, [while] high-risk AI applications go through a more diligent process."
Stave also pointed out that minimal oversight in the U.S. doesn't necessarily mean that companies will operate in a fully unregulated environment. In the absence of a cohesive global standard, large incumbents operating in multiple regions typically end up adhering to the most stringent regulations by default. In this way, the EU's AI Act could end up functioning similarly to GDPR, setting de facto standards for companies building or deploying AI worldwide.
8. AI-related security concerns escalate
The widespread availability of generative AI, often at low or no cost, gives threat actors unprecedented access to tools for facilitating cyberattacks. That risk is poised to increase in 2025 as multimodal models become more sophisticated and readily accessible.
In a recent public warning, the FBI described several ways cybercriminals are using generative AI for phishing scams and financial fraud. For example, an attacker targeting victims via a deceptive social media profile might write convincing bio text and direct messages with an LLM, while using AI-generated fake photos to lend credibility to the false identity.
AI video and audio pose a growing threat, too. Historically, models have been limited by telltale signs of inauthenticity, like robotic-sounding voices or lagging, glitchy video. While today's versions aren't perfect, they're significantly better, especially if an anxious or time-pressured victim isn't looking or listening too closely.
Audio generators can enable hackers to impersonate a victim's trusted contacts, such as a spouse or colleague. Video generation has so far been less common, as it's more expensive and offers more opportunities for error. But, in a highly publicized incident earlier this year, scammers successfully impersonated a company's CFO and other staff members on a video call using deepfakes, leading a finance worker to send $25 million to fraudulent accounts.
Other security risks are tied to vulnerabilities within models themselves, rather than social engineering. Adversarial machine learning and data poisoning, where inputs and training data are intentionally designed to mislead or corrupt models, can damage AI systems themselves. To account for these risks, businesses will need to treat AI security as a core part of their overall cybersecurity strategies.
Lev Craig covers AI and machine learning as site editor for TechTarget's Enterprise AI site. Craig graduated from Harvard University with a bachelor's degree in English and has previously written about enterprise IT, software development and cybersecurity.