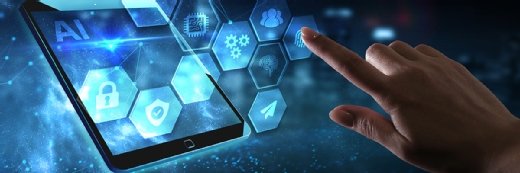
putilov_denis - stock.adobe.com
How to measure the ROI of enterprise AI initiatives
Interest in AI tools and systems has skyrocketed across industries. To ensure their endeavors are worthwhile, businesses are increasingly emphasizing return on investment.
Despite AI's transformative potential across various industry sectors, quantifying its financial impact remains difficult due to unique factors that differentiate AI from other IT investments.
Many sectors have identified valuable AI use cases. The IT and manufacturing industries are looking to AI tools to improve operational efficiency, while major retailers and e-commerce platforms hope to enhance customer experience using AI. Scientific researchers are using machine learning models to accelerate the development of lifesaving medicines.
Regardless of industry, it's essential for organizations considering AI initiatives to define the technology's value proposition, either in a comprehensive AI strategy or as a component of a broader business strategy. This involves crafting use cases for AI that address the organization's specific challenges and objectives.
Once the value proposition is defined, measuring the AI initiative's return on investment is crucial to demonstrate actionable results for stakeholders. Evaluating AI ROI -- a process that involves multiple steps and metrics -- ensures that AI initiatives meet their goals and deliver value to the business.
A framework for evaluating AI ROI
Evaluating the ROI of AI initiatives involves complexities that differ from traditional IT deployments. For instance, cloud computing ROI typically focuses on shifting from capital expenditures, such as server and data center costs, to operational expenditures for ongoing services.
In contrast, measuring the ROI of AI requires considering more complex, longer-term factors that extend beyond simple financial metrics to encompass a deeper analysis of strategic and operational metrics. On the cost side, this might include expenses related to data acquisition, model development, computational resources and ongoing maintenance. Benefits, meanwhile, could include factors such as efficiency gains, more informed decision-making and stronger market positioning.
Whereas cloud ROI is often measured financially, AI ROI calculations emphasize improving decision-making, increasing productivity, automating tasks and enhancing customer experiences. AI's financial impact can also be quantified in terms of increased revenue, reduced costs or competitive advantages gained through innovation. For these reasons, measuring AI ROI is best done by following the below steps.
1. Define the AI initiative's objectives and scope
First, introduce AI into the organization with a framework that clarifies how the AI initiative aligns with the organization's broader business objectives.
This framework must also capture the scope and scale of the AI implementation, detailing the specific processes targeted for AI enhancement or automation. Ensure that messaging and communications from project leaders explain how the AI framework will improve employee productivity, not replace employees.
2. Define and capture quantitative metrics
Evaluate the potential cost savings of the AI initiative by measuring reductions in operational costs via process automation and efficiency improvements, as well as revenue gained through AI.
Capture and document operational costs, including those associated with developing, deploying and maintaining AI tools and systems. Note that deploying AI to the public cloud will noticeably affect cloud spending, which might necessitate adjustments to current cloud cost management processes. To assess revenue growth due to AI, capture data about increased sales and analyze any new revenue streams that AI initiatives create.
3. Define and capture performance metrics
Emphasize gathering metrics such as downtime reduction, decision-making improvements, scalability within budget and qualitative metrics. Defining and capturing qualitative metrics helps avoid implementing AI just for the sake of it. Expect to spend some time identifying the right qualitative metrics to track.
If the organization is integrating AI into existing products and services, closely study how AI contributes to product innovation and market differentiation. Sales and marketing data can help illustrate this. It's also crucial to get direct input from users if the AI initiative only focuses on internal applications.
4. Align AI closely with operations
Assess how AI projects contribute to the organization's overall strategic goals, especially their impact on key performance indicators, and optimize the analysis and usage of back-end data. An AI project's ability to scale as the organization grows or downsizes indicates that it is a strategic fit.
Gauging employee satisfaction with AI can be tricky. Human nature and distrust of corporations can lead some employees to worry that AI will take their jobs. Developing a documented plan that outlines how AI will augment and improve existing workflows can better position AI as a tool to improve employee satisfaction.
Finally, track engagement levels with new AI systems, whether internal or external. Increased interaction shows that the AI system is well aligned with business users and customers.
5. Monitor AI risk and compliance
Monitoring the risk and compliance of corporate AI initiatives is a necessary element of measuring ROI. Assess AI systems' compliance with relevant data protection regulations, such as the GDPR and the California Consumer Privacy Act.
AI projects might also raise ethical concerns, such as bias in decision-making processes or lack of transparency in AI operations. It takes a cross-functional team to determine whether AI projects are free of ethical concerns. If possible, speak to vocal customers about their ethical concerns.
6. Practice continuous evaluation and iteration
Measuring AI ROI isn't a one-and-done process; it requires continuously measuring, evaluating and readjusting for changes. Factors such as technology advancements and changes to business operations will require recalculating the ROI of AI initiatives.
The process starts by extending performance monitoring tools that are already in place to AI applications, enabling the business to measure them against its performance objectives. Expect to take some time to improve reporting as operations teams learn more about AI application performance and reporting.
To integrate the human element of continuous evaluation, establish collaborative relationships with stakeholders who will see the most impact from AI applications. Their feedback can be invaluable to organizations iterating on AI tools, processes and frameworks.
Will Kelly is a freelance writer and content strategist who has written about cloud, DevOps, AI and enterprise mobility.