Generative AI ethics: 11 biggest concerns and risks
As adoption and use cases grow, generative AI is upending business models and driving ethical issues such as misinformation, brand integrity and job displacement to the forefront.
Like other forms of AI, generative AI can affect ethical issues and risks pertaining to data privacy, security, energy usage, political impact and workforces. GenAI technology can also potentially introduce a series of new business risks, such as misinformation and hallucinations, plagiarism, copyright infringements and harmful content. Lack of transparency and the potential for worker displacement are additional issues that enterprises might need to address.
"Many of the risks posed by generative AI ... are enhanced and more concerning than those [associated with other types of AI]," said Tad Roselund, managing director and senior partner at consultancy BCG. Those risks require a comprehensive approach, including a clearly defined strategy, good governance and a commitment to responsible AI.
Corporate cultures that use GenAI should consider the following 11 issues:
1. Distribution of harmful content
Generative AI systems can create content automatically based on text prompts by humans. "These systems can generate enormous productivity improvements, but they can also be used for harm, either intentional or unintentional," explained Bret Greenstein, partner and generative AI leader at professional services consultancy PwC. An AI-generated email sent on behalf of the company, for example, could inadvertently contain offensive language or issue harmful guidance to employees. GenAI should be used to augment but not replace humans or processes, Greenstein advised, to ensure content meets the company's ethical expectations and supports its brand values.
2. Copyright and legal exposure
Popular generative AI tools are trained on massive image and text databases from multiple sources, including the internet. When these tools create images or generate lines of code, the data's source could be unknown, which might be problematic for a bank handling financial transactions or a pharmaceutical company relying on a formula for a complex molecule in a drug. Reputational and financial risks could also be massive if one company's product is based on another company's intellectual property. "Companies must look to validate outputs from the models," Roselund advised, "until legal precedents provide clarity around IP and copyright challenges."
This article is part of
What is GenAI? Generative AI explained
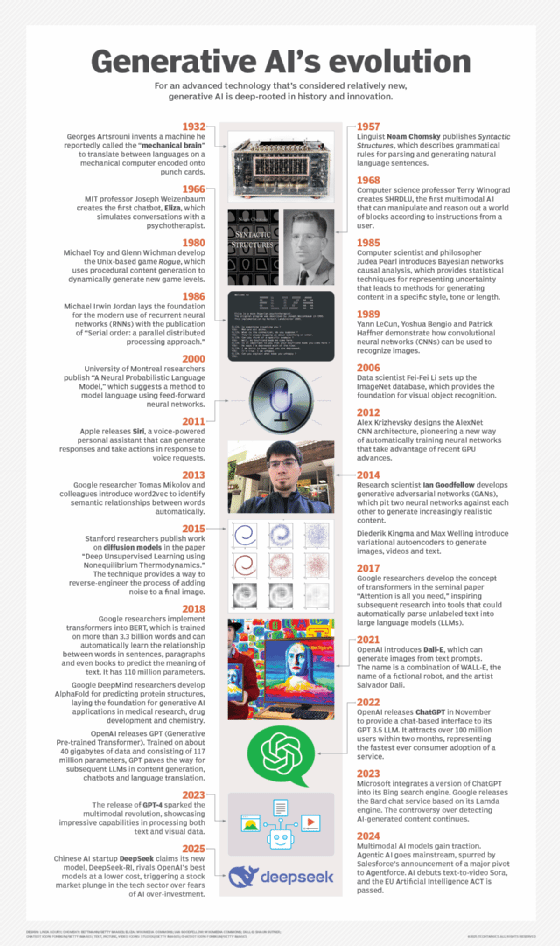
Generative AI large language models (LLMs) are trained on data sets that might include personally identifiable information (PII) about individuals. This data can sometimes be elicited with a simple text prompt.
Moreover, compared with traditional search engines, it can be more difficult for consumers to locate and request removal of the information. Companies that build or fine-tune LLMs must ensure that PII isn't embedded in the language models and that it's easy to remove PII from these models in compliance with privacy laws.
4. Sensitive information disclosure
GenAI is democratizing AI capabilities and making them more accessible. This combination of democratization and accessibility, Roselund said, could potentially lead to a medical researcher inadvertently disclosing sensitive patient information or a consumer brand unwittingly exposing its product strategy to a third party. The consequences of unintended incidents like these could irrevocably breach patient or customer trust and carry legal ramifications. Roselund recommended that companies institute clear guidelines, governance and effective communication from the top down, emphasizing shared responsibility for safeguarding sensitive information, protected data and IP.
5. Amplification of existing bias
Generative AI can potentially amplify existing bias. For example, there can be bias in the data used for training LLMs, which can be outside the control of companies that use these language models for specific applications. It's critically important for companies working on AI to have diverse leaders and subject matter experts to help identify bias in data and models, Greenstein said.
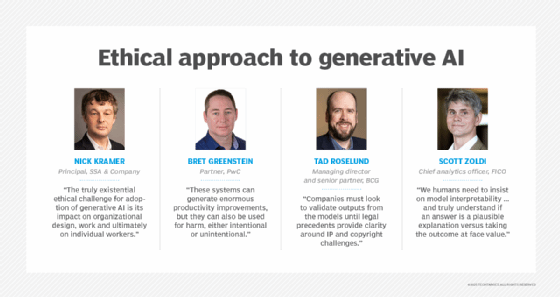
6. Workforce roles and morale
AI is being trained to do more of the daily tasks that knowledge workers do, including writing, coding, content creation, summarization and analysis, Greenstein said. Although worker displacement and replacement have been ongoing since the first AI and automation tools were deployed, the pace has accelerated as a result of the innovations in generative AI technologies. "The future of work itself is changing," Greenstein added, "and the most ethical companies are investing in this [change]."
Ethical responses have included investments in preparing certain parts of the workforce for the new roles created by generative AI applications. Businesses, for example, will need to help employees develop generative AI skills such as prompt engineering. "The truly existential ethical challenge for adoption of generative AI is its impact on organizational design, work and ultimately on individual workers," said Nick Kramer, vice president of applied solutions at consultancy SSA & Company. "This will not only minimize the negative impacts, but it will also prepare the companies for growth."
7. Data provenance
GenAI systems consume tremendous volumes of data that could be inadequately governed, of questionable origin, used without consent or biased. Additional levels of inaccuracy could be amplified by social influencers or the AI systems themselves.
"The accuracy of a generative AI system depends on the corpus of data it uses and its provenance," explained Scott Zoldi, chief analytics officer at credit scoring services company FICO. "ChatGPT-4 is mining the internet for data, and a lot of it is truly garbage, presenting a basic accuracy problem on answers to questions to which we don't know the answer." FICO, according to Zoldi, has been using generative AI for more than a decade to simulate edge cases in training fraud detection algorithms. The generated data is always labeled as synthetic data, so Zoldi's team knows where the data is allowed to be used. "We treat it as walled-off data for the purposes of test and simulation only," he said. "Synthetic data produced by generative AI does not inform the model going forward in the future. We contain this generative asset and do not allow it 'out in the wild.'"
8. Lack of explainability and interpretability
Many generative AI systems group facts together probabilistically, going back to the way AI has learned to associate data elements with one another, Zoldi explained. But these details aren't always revealed when using applications like ChatGPT. Consequently, data trustworthiness is called into question.
When interrogating GenAI, analysts expect to arrive at a causal explanation for outcomes. But machine learning models and generative AI search for correlations, not causality. "That's where we humans need to insist on model interpretability -- the reason why the model gave the answer it did," Zoldi said. "And truly understand if an answer is a plausible explanation versus taking the outcome at face value."
Until that level of trustworthiness can be achieved, GenAI systems should not be relied upon to provide answers that could significantly affect lives and livelihoods.
9. AI hallucinations
Generative AI techniques all use various combinations of algorithms, including autoregressive models, autoencoders and other machine learning algorithms, to distill patterns and generate content. As good as these models are at identifying new patterns, they sometimes struggle with teasing out important distinctions relevant to human use cases.
This can include creating authoritative-sounding but inaccurate prose or producing pictures with realistic-looking imagery but misshapen representations of humans that contain extra fingers or eyes. With language models, these errors can show up as chatbots inaccurately representing corporate policies, such as in the case of an Air Canada chatbot that misrepresented corporate policies regarding bereavement benefits. Lawyers using these tools have also been fined for filing briefs that cited nonexistent court cases.
Newer techniques like retrieval augmented generation and agentic AI frameworks can help reduce these issues. However, it's important to keep humans in the loop to verify the accuracy of generative AI information to avoid customer backlash, sanctions or other problems.
10. Carbon footprint
Many AI vendors argue that bigger AI models can deliver better results. This is partly true, but it can often involve considerably more data center resources, either for training new AI models or running AI inference processes in production. The issue is hardly clear-cut. As some argue, improving an AI model that has the potential to reduce the carbon footprint of an employee traveling to work or the efficiency of a product could be a good thing. Conversely, developing that model could also exacerbate global warming or other environmental problems
11. Political impact
The political impact of GenAI technologies is a fraught topic. On the one hand, better GenAI tools have the potential to make the world a better place. At the same time, they could also enable various political actors -- voters, politicians, authoritarians -- to make communities worse. One example of generative AI's negative impact on politics can be found in social media platforms that algorithmically promote or create divisive comments as a strategy for increasing engagement (and profits) for their owners over comments that find common ground but might not have the same click-through and sharing numbers.
These issues will continue to be thorny for years to come as societies sort out which GenAI use cases serve the public good and whether that should be the end goal.
Editor's note: This article was updated in 2025 to include additional ethical issues and concerns stemming from the use of generative AI.
George Lawton is a journalist based in London. Over the last 30 years, he has written more than 3,000 stories about computers, communications, knowledge management, business, health and other areas that interest him.