12 top resources to build an ethical AI framework
Examine the standards, tools and techniques available to help navigate the nuances and complexities in establishing a generative AI ethics framework that supports responsible AI.
As generative AI (GenAI) gains a stronger foothold in the enterprise, executives are called upon to bring greater attention to AI ethics, moral principles and methods that help shape the development and use of AI technology.
An ethical approach to AI is important, not only to making the world a better place but also to protecting a company's bottom line, since it involves understanding the financial and reputational impact of biased data, hallucinations, transparency, explainability, limitations and other factors that erode public trust in AI.
"The most impactful frameworks or approaches to addressing ethical AI issues … take all aspects of the technology -- its usage, risks and potential outcomes -- into consideration," said Tad Roselund, managing director and senior partner at Boston Consulting Group.
Many firms approach the development of ethical AI frameworks from a purely values-based position, Roselund said. It's important to take a holistic, ethical AI approach that integrates strategy with process and technical controls, cultural norms and governance. These three elements of an ethical AI framework can help institute responsible AI policies and initiatives. And it all starts by establishing a set of principles around AI usage.
This article is part of
What is GenAI? Generative AI explained
"Oftentimes, businesses and leaders are narrowly focused on one of these elements when they need to focus on all of them," Roselund reasoned. Addressing any one element might be a good starting point, but by considering all three elements -- controls, cultural norms and governance -- businesses can devise an all-encompassing ethical AI framework. This approach is especially important when it comes to GenAI and its ability to democratize the use of AI.
Enterprises must also instill AI ethics in those who develop and use AI tools and technologies. Open communication, educational resources, and enforced guidelines and processes to ensure the proper use of AI, Roselund advised, can further bolster an internal AI ethics framework that addresses GenAI.
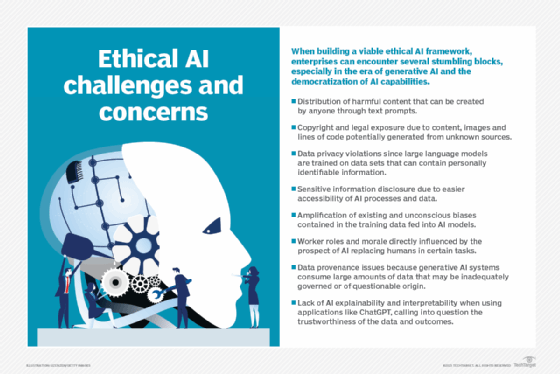
Top resources to shape an ethical AI framework
The following is an alphabetical list of standards, tools, techniques and other resources to help shape a company's internal ethical AI framework.
1. AI Now Institute
The institute focuses on the social implications of AI and policy research in responsible AI. Research areas include algorithmic accountability, antitrust concerns, biometrics, worker data rights, large-scale AI models and privacy. The April report "AI Now 2023 Landscape: Confronting Tech Power" provides a deep dive into many ethical issues that can be helpful in developing a responsible AI policy.
2. Berkman Klein Center for Internet & Society at Harvard University
The center fosters research into the big questions related to the ethics and governance of AI. It has contributed to the dialogue about information quality, influenced policymaking on algorithms in criminal justice, supported the development of AI governance frameworks, studied algorithmic accountability and collaborated with AI vendors.
3. CEN-CENELEC Joint Technical Committee 21 on Artificial Intelligence
JTC 21 is an ongoing EU initiative for various responsible AI standards. The group focuses on producing standards for the European market and informing EU legislation, policies and values. It also plans to specify technical requirements for characterizing transparency, robustness and accuracy in AI systems.
4. Institute for Technology, Ethics and Culture Handbook
The ITEC Handbook was a collaborative effort between Santa Clara University's Markkula Center for Applied Ethics and the Vatican to develop a practical, incremental roadmap for technology ethics. The handbook includes a five-stage maturity model with specific, measurable steps that enterprises can take at each level of maturity. It also promotes an operational approach for implementing ethics as an ongoing practice, akin to DevSecOps for ethics. The core idea is to bring legal, technical and business teams together during ethical AI's early stages to root out bugs at a time when they're much cheaper to fix than after responsible AI deployment.
5. IEEE Global Initiative 2.0 on Ethics of Autonomous and Intelligent Systems
The initiative focuses on ethical issues with incorporating GenAI into future autonomous and agentic systems. In particular, the group is exploring how to extend traditional safety concepts to include scientific integrity and public safety as priorities. Of note, they are exploring how the concept of safety has sometimes been misappropriated and misunderstood with regard to new GenAI risks. This working group is developing new standards, toolkits, AI safety champions and awareness campaigns to improve the ethical use of GenAI tools and infrastructure.
6. ISO/IEC 23894:2023
The standard describes how an organization can manage risks specifically related to AI. It can help standardize the technical language characterizing the underlying principles and how these principles apply to developing, provisioning or offering AI systems. It also covers policies, procedures and practices to assess, treat, monitor, review and record risk. It's highly technical and oriented toward engineers rather than business experts.
For more on generative AI, read the following articles:
AI content generators to explore
The best large language models
Attributes of open vs. closed AI explained
Generative models: VAEs, GANs, diffusion, transformers, NeRFs
7. NIST AI Risk Management Framework
AI RMF 1.0 guides government agencies and the private sector in managing new AI risks and in promoting responsible AI. According to NIST, the framework is "intended to be voluntary, rights-preserving, non-sector-specific, and use-case agnostic."
8. Nvidia NeMo Guardrails
The open source toolkit provides a flexible interface for defining the specific behavioral rails that bots need to follow. It supports the Colang modeling language. One chief data scientist said his company uses Nvidia NeMo Guardrails to prevent a support chatbot on a lawyer's website from providing answers that might be construed as legal advice.
9. Partnership on AI to Benefit People and Society
PAI has been exploring many fundamental assumptions about how to build AI systems that benefit all stakeholders. Founding members include executives from Amazon, Facebook, Google, Google DeepMind, Microsoft and IBM. Supporting members include more than a hundred partners from academia, civil society, industry and various nonprofits. One novel aspect of this group has been the creation of an AI Incident Database to assess, manage and communicate newly discovered AI risks and harms. It has also explored many of the concerns related to the opportunities and harms of AI-generated content.
10. Stanford Institute for Human-Centered Artificial Intelligence
HAI provides ongoing research and guidance into best practices for human-centered AI. One early initiative in collaboration with Stanford Medicine is Responsible AI for Safe and Equitable Health, which addresses ethical and safety issues surrounding AI in health and medicine.
11. "Towards Unified Objectives for Self-Reflective AI" paper
This paper by Matthias Samwald, Robert Praas and Konstantin Hebenstreit takes a Socratic approach to identify underlying assumptions, contradictions and errors through dialogue and questioning about truthfulness, transparency, robustness and alignment of ethical principles. One goal is to develop AI meta-systems in which two or more component AI models complement, critique and improve their mutual performance.
12. World Economic Forum's "The Presidio Recommendations on Responsible Generative AI" white paper
This June 2023 white paper includes 30 "action-oriented" recommendations to "navigate AI complexities and harness its potential ethically." It includes sections on responsible development and release of GenAI, open innovation and international collaboration, and social progress.
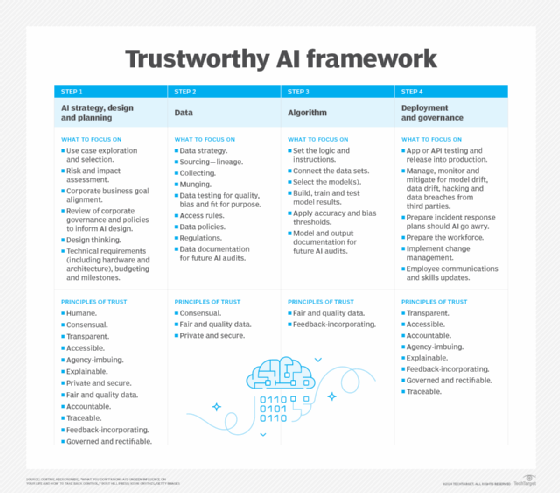
Best ethical AI practices
Ethical AI resources are a sound starting point toward tailoring and establishing a company's ethical AI framework and launching responsible AI policies and initiatives. The following best practices can help achieve these goals:
- Appoint an ethics leader. There are instances when many well-intentioned people sit around a table discussing various ethical AI issues but fail to make informed, decisive calls to action, Roselund noted. A single leader appointed by the CEO can drive decisions and actions.
- Take a cross-functional approach. Implementing AI tools and technologies companywide requires cross-functional cooperation, so the policies and procedures to ensure the responsible use of AI need to reflect that approach, Roselund advised. Ethical AI requires leadership, but its success isn't the sole responsibility of one person or department.
- Customize the ethical AI framework. A GenAI ethics framework should be tailored to a company's unique style, objectives and risks. Harmonize ethical AI programs with existing workflows and governance structures.
- Establish ethical AI measurements. For employees to buy into an ethical AI framework and responsible AI policies, companies need to be transparent about their intentions, expectations and corporate values, as well as their plans to measure success. "Employees not only need to be made aware of these new ethical emphases, but they also need to be measured in their adjustment and rewarded for adjusting to new expectations," explained Brian Green, director of technology ethics at Santa Clara University's Markkula Center for Applied Ethics.
- Be open to different opinions. It's essential to engage a diverse group of voices, including ethicists, field experts and those in surrounding communities that AI deployments might affect. "By working together, we gain a deeper understanding of ethical concerns and viewpoints and develop AI systems that are inclusive and respectful of diverse values," said Paul Pallath, vice president of the applied AI practice at technology consultancy Searce.
- Take a holistic perspective. Legalities don't always align with ethics, Pallath cautioned. Sometimes, legally acceptable actions might raise ethical concerns. Ethical decision-making needs to address both legal and moral aspects. This approach ensures AI technologies meet legal requirements and uphold ethical principles to safeguard the well-being of individuals and society.
Future of ethical AI frameworks
Researchers, enterprise leaders and regulators are still investigating ethical issues related to responsible AI. Increasingly, enterprises will need to consider ethics, not just as a liability and checkmark item but as a lens to expand opportunities for collecting better data and building trust with customers and regulators.
Legal challenges involving copyright and intellectual property protection will also have to be addressed. Issues related to GenAI and hallucinations will take longer to address, since some of those potential problems are inherent in the design of today's AI systems. In the U.S., copyright regulators have agreed that AI-generated content is copyrightable with some level of human involvement. There are still many question marks relating to training data, which sometimes includes copyrighted content, violation of terms of service and even blatant pirating of copyrighted works by large AI developers like Meta. The courts are still deciding these matters, and risk-averse companies might consider steering clear of the AI tools and services built on some of these practices -- even when they are proffered as "open AI."
Enterprises and data scientists will also need to solve issues of bias and inequality in training data and machine learning algorithms. In addition, issues relating to AI system security, including cyberattacks against large language models, will require continuous engineering and design improvements to keep pace with increasingly sophisticated criminal adversaries. The NIST AI Risk Management Framework could help mitigate some of these concerns.
In the near future, Pallath sees AI evolving toward enhancing human capabilities in collaboration with AI technologies rather than supplanting humans entirely. "Ethical considerations," he explained, "will revolve around optimizing AI's role in augmenting human creativity, productivity and decision-making, all while preserving human control and oversight."
AI ethics will continue to be a fast-growing movement for the foreseeable future, Green said. "With AI," he acknowledged, "now we have created thinkers outside of ourselves and discovered that, unless we give them some ethical thoughts, they won't make good choices."
AI ethics is never done. Ethical judgments might need to change as conditions change. "We need to maintain our awareness and skill," Green said, "so that, if AI is not benefiting society, we can make the necessary improvements."
For example, new GenAI tools could give nations a leg up in critical areas such as warfare or more effective cyberattacks, yet merely developing these tools could also empower adversaries. This could result in a zero-sum situation that benefits no one and costs money in the process. These issues are getting thornier, particularly with the growth of open source AI that could also empower nation-states and malicious adversaries. However, stifling open source AI development could also disempower more efficient and effective AI development in the long run.
Editor's note: This article was updated in March 2025 to include additional ethical AI framework resources.
George Lawton is a journalist based in London. Over the last 30 years, he has written more than 3,000 stories about computers, communications, knowledge management, business, health and other areas that interest him.