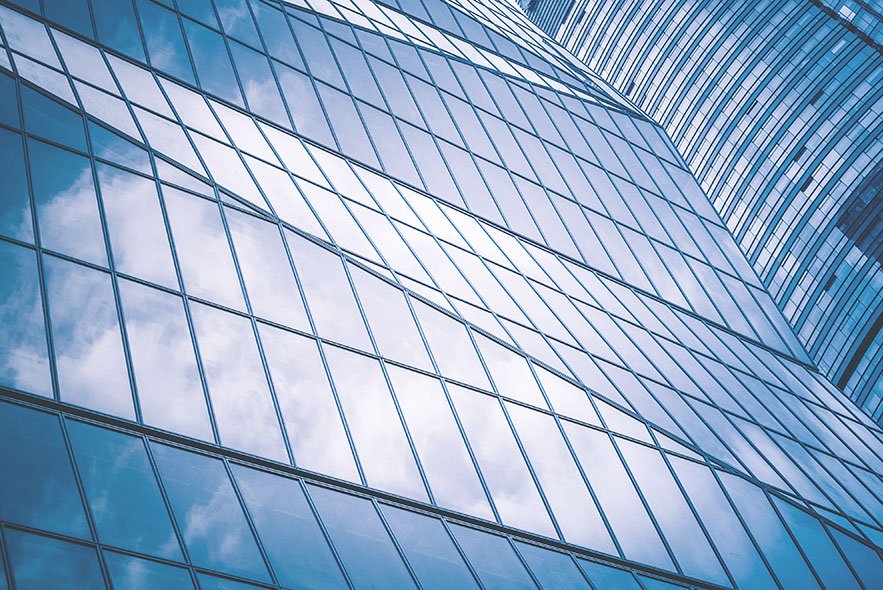
What is AI ethics?
AI ethics is a system of moral principles and techniques intended to inform the development and responsible use of artificial intelligence technology. As AI has become integral to products and services, organizations are also starting to develop AI codes of ethics.
An AI code of ethics, also called an AI value platform, is a policy statement that formally defines the role of artificial intelligence as it applies to the development and well-being of humans. The purpose of an AI code of ethics is to provide stakeholders with guidance when faced with an ethical decision regarding the use of artificial intelligence.
Isaac Asimov, a science fiction writer, foresaw the potential dangers of autonomous AI agents in 1942, long before the development of AI systems. He created the Three Laws of Robotics as a means of limiting those risks. In Asimov's code of ethics, the first law forbids robots from actively harming humans or allowing harm to come to humans by refusing to act. The second law orders robots to obey humans unless the orders aren't in accordance with the first law. The third law orders robots to protect themselves insofar as doing so is in accordance with the first two laws.
Today, the potential dangers of AI systems include AI replacing human jobs, AI hallucinations, deepfakes and AI bias. These issues could be responsible for human layoffs, misleading information, falsely mimicking others' images or voices, or prejudiced decisions that could negatively affect human lives.
This article is part of
What is enterprise AI? A complete guide for businesses
The rapid advancement of AI in the past 10 years has spurred groups of experts to develop safeguards for protecting against the risk of AI to humans. One such group is the nonprofit Future of Life Institute founded by Massachusetts Institute of Technology cosmologist Max Tegmark, Skype co-founder Jaan Tallinn and Google DeepMind research scientist Victoria Krakovna. The institute worked with AI researchers and developers, as well as scholars from many disciplines, to create the 23 guidelines now referred to as the Asilomar AI Principles.
Kelly Combs, director advisory of Emerging Technology Risk Services for KPMG US, said that, when developing an AI code of ethics, "it's imperative to include clear guidelines on how the technology will be deployed and continuously monitored." These policies should mandate measures that guard against unintended bias in machine learning (ML) algorithms, continuously detect drift in data and algorithms, and track both the provenance of data and the identity of those who train algorithms.
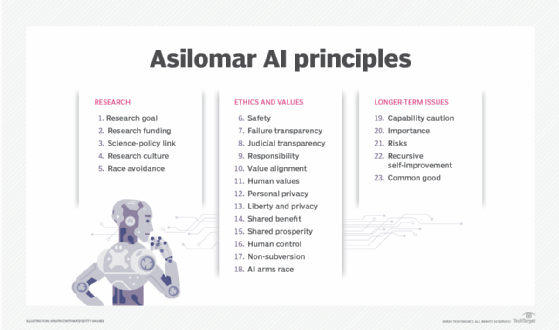
Principles of AI ethics
There isn't one set of defined principles of AI ethics that someone can follow when implementing an AI system. Some principles, for example, follow the Belmont Report's example. The Belmont Report was a 1979 paper created by the National Commission for the Protection of Human Subjects of Biomedical and Behavioral Research. It referenced the following three guiding principles for the ethical treatment of human subjects:
- Respect for persons. This recognizes the autonomy of individuals and relates to the idea of informed consent.
- Beneficence. This relates to the idea of do no harm.
- Justice. This principle focuses on fairness and equality.
Although the Belmont Report was originally developed regarding human research, it has recently been applied to AI development by academic researchers and policy institutes. The first point relates to the consent and transparency relating to individual rights when engaging with AI systems. The second point relates to ensuring that AI systems don't cause harm, like amplifying biases. And the third point relates to how access to AI is distributed equally.
Other common ideas when it comes to AI ethics principles include the following:
- Transparency and accountability.
- Human-focused development and use.
- Security.
- Sustainability and impact.
Why are AI ethics important?
AI is a technology designed by humans to replicate, augment or replace human intelligence. These tools typically rely on large volumes of various types of data to develop insights. Poorly designed projects built on data that's faulty, inadequate or biased can have unintended, potentially harmful consequences. Moreover, the rapid advancement in algorithmic systems means that, in some cases, it isn't clear how the AI reached its conclusions, so humans are essentially relying on systems they can't explain to make decisions that could affect society.
An AI ethics framework is important because it highlights the risks and benefits of AI tools and establishes guidelines for their responsible use. Coming up with a system of moral tenets and techniques for using AI responsibly requires the industry and interested parties to examine major social issues and, ultimately, the question of what makes us human.
What are the ethical challenges of AI?
Enterprises face the following ethical challenges in their use of AI technologies:
- Explainability. When AI systems go awry, teams must be able to trace through a complex chain of algorithmic systems and data processes to find out why. Organizations using AI should be able to explain the source data, resulting data, what their algorithms do and why they're doing that. "AI needs to have a strong degree of traceability to ensure that, if harms arise, they can be traced back to the cause," said Adam Wisniewski, CTO and co-founder of AI Clearing.
- Responsibility. Society is still sorting out responsibility when decisions made by AI systems have catastrophic consequences, including loss of capital, health or life. The process of addressing accountability for the consequences of AI-based decisions should involve a range of stakeholders, including lawyers, regulators, AI developers, ethics bodies and citizens. One challenge is finding the appropriate balance in cases where an AI system might be safer than the human activity it's duplicating but still causes problems, such as weighing the merits of autonomous driving systems that cause fatalities but far less than people do.
- Fairness. In data sets involving personally identifiable information, it's extremely important to ensure that there are no biases in terms of race, gender or ethnicity.
- Ethics. AI algorithms can be used for purposes other than those for which they were created. For example, people might use the technology to make deepfakes of others or to manipulate others by spreading misinformation. Wisniewski said these scenarios should be analyzed at the design stage to minimize the risks and introduce safety measures to reduce the adverse effects in such cases.
- Privacy. Because AI takes massive amounts of data to train properly, some companies are using publicly facing data, such as data from web forums or social media posts, for training. Other companies like Facebook, Google and Adobe have also added into their policies that they can take user data to train their AI models, leading to controversies in protecting user data.
- Job displacement. Instead of using AI to help make human jobs easier, some organizations might choose to use AI tools to replace human jobs altogether. This practice has been met with controversy, as it directly affects those who it replaces, and AI systems are still prone to hallucinations and other imperfections.
- Environmental. AI models require a lot of energy to train and run, leaving behind a large carbon footprint. If the electricity it uses is generated primarily from coal or fossil fuels, it causes even more pollution.
The public release and rapid adoption of generative AI applications, such as ChatGPT and Dall-E, which are trained on existing data to generate new content, amplify the ethical issues related to AI, introducing risks related to misinformation, plagiarism, copyright infringement and harmful content.
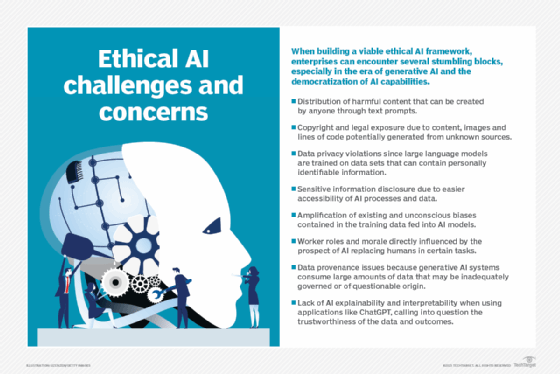
What are the benefits of ethical AI?
The rapid acceleration in AI adoption across businesses has coincided with -- and, in many cases, helped fuel -- two major trends: the rise of customer-centricity and the rise in social activism.
"Businesses are rewarded not only for providing personalized products and services, but also for upholding customer values and doing good for the society in which they operate," said Sudhir Jha, CEO at Brighterion and executive vice president at Mastercard.
AI plays a huge role in how consumers interact with and perceive a brand. Responsible use is necessary to ensure a positive impact. In addition to consumers, employees want to feel good about the businesses they work for. "Responsible AI can go a long way in retaining talent and ensuring smooth execution of a company's operations," Jha said.
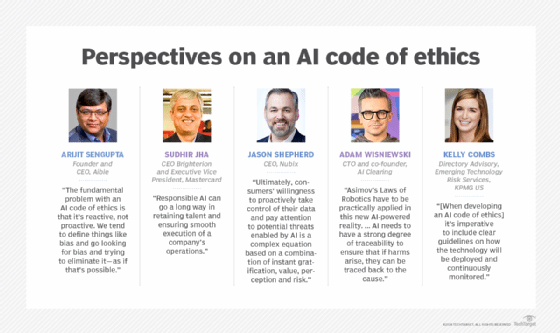
What is an AI code of ethics?
A proactive approach to ensuring ethical AI requires addressing the following three key areas, according to Jason Shepherd, CEO at Atym:
- Policy. This includes developing the appropriate framework for driving standardization and establishing regulations. Documents like the Asilomar AI Principles can be useful when starting the conversation. Government agencies in the U.S., Europe and elsewhere have launched efforts to ensure ethical AI, and a raft of standards, tools and techniques from research bodies, vendors and academic institutions are available to help organizations craft AI policy. See the "Resources for developing ethical AI" section below. Ethical AI policies need to address how to deal with legal issues when something goes wrong. Companies should consider incorporating AI policies into their own codes of conduct. But effectiveness depends on employees following the rules, which might not always be realistic when money or prestige are on the line.
- Education. Executives, data scientists, frontline employees and consumers all need to understand policies, key considerations and potential negative effects of unethical AI and fake data. One big concern is the tradeoff between ease of use around data sharing and AI automation and the potential negative repercussions of oversharing or adverse automation. "Ultimately, consumers' willingness to proactively take control of their data and pay attention to potential threats enabled by AI is a complex equation based on a combination of instant gratification, value, perception and risk," Shepherd said.
- Technology. Executives also need to architect AI systems to automatically detect fake data and unethical behavior. This requires not just looking at a company's own AI, but vetting suppliers and partners for the malicious use of AI. Examples include the deployment of deepfake videos and text to undermine a competitor or the use of AI to launch sophisticated cyberattacks. This will become more of an issue as AI tools become commoditized. To combat this potential snowball effect, organizations must invest in defensive measures rooted in open, transparent and trusted AI infrastructure. Shepherd believes this will give rise to the adoption of trust that provides a system-level approach to automating privacy assurance, ensuring data confidence and detecting unethical use of AI.
Examples of AI codes of ethics
An AI code of ethics can spell out the principles and provide the motivation that drives appropriate behavior. For example, the following summarizes Mastercard's AI code of ethics:
- An ethical AI system must be inclusive, explainable, positive in nature and use data responsibly.
- An inclusive AI system is unbiased. AI models require training with large and diverse data sets to help mitigate bias. It also requires continued attention and tuning to change any problematic attributes learned in the training process.
- An explainable AI system should be manageable to ensure companies can ethically implement it. Systems should be transparent and explainable.
- An AI system should have and maintain a positive purpose. In many cases, this could be done to aid humans in their work, for example. AI systems should be implemented in a way so they can't be used for negative purposes.
- Ethical AI systems should also abide by data privacy rights. Even though AI systems typically require a massive amount of data to train, companies shouldn't sacrifice their users' or other individuals' data privacy rights to do so.
Companies such as Mastercard, Salesforce and Lenovo have committed to signing a voluntary AI code of conduct, which means they're committed to the responsible development and management of generative AI systems. The code is based on the development of a Canadian code of ethics regarding generative AI, as well as input gathered from a collection of stakeholders.
Google also has its own guiding AI principles, which emphasize AI's accountability, potential benefits, fairness, privacy, safety, social and other human values.
Resources for developing ethical AI
The following is a sampling of the growing number of organizations, policymakers and regulatory standards focused on developing ethical AI practices:
- AI Now Institute. The institute focuses on the social implications of AI and policy research in responsible AI. Research areas include algorithmic accountability, antitrust concerns, biometrics, worker data rights, large-scale AI models and privacy. The "AI Now 2023 Landscape: Confronting Tech Power" report provides a deep dive into many ethical issues that can be helpful in developing ethical AI policies.
- Berkman Klein Center for Internet & Society at Harvard University. The center fosters research into the big questions related to the ethics and governance of AI. Research supported by the center has tackled topics that include information quality, algorithms in criminal justice, development of AI governance frameworks and algorithmic accountability.
- CEN-CENELEC Joint Technical Committee 21 Artificial Intelligence. JTC 21 is an ongoing European Union (EU) initiative for various responsible AI standards. The group produces standards for the European market and informs EU legislation, policies and values. It also specifies technical requirements for characterizing transparency, robustness and accuracy in AI systems.
- Institute for Technology, Ethics and Culture Handbook. The ITEC Handbook is a collaborative effort between Santa Clara University's Markkula Center for Applied Ethics and the Vatican to develop a practical, incremental roadmap for technology ethics. The ITEC Handbook includes a five-stage maturity model with specific, measurable steps that enterprises can take at each level of maturity. It also promotes an operational approach for implementing ethics as an ongoing practice, akin to DevSecOps for ethics. The core idea is to bring legal, technical and business teams together during ethical AI's early stages to root out the bugs at a time when they're much cheaper to fix than after responsible AI deployment.
- ISO/IEC 23894:2023 IT-AI-Guidance on risk management. The International Organization for Standardization and International Electrotechnical Commission describe how an organization can manage risks specifically related to AI. The guidance can help standardize the technical language characterizing underlying principles and how they apply to developing, provisioning or offering AI systems. It also covers policies, procedures and practices for assessing, treating, monitoring, reviewing and recording risk. It's highly technical and oriented toward engineers rather than business experts.
- NIST AI Risk Management Framework. This guide from the National Institute of Standards and Technology for government agencies and the private sector focuses on managing new AI risks and promoting responsible AI. Abhishek Gupta, founder and principal researcher at the Montreal AI Ethics Institute, pointed to the depth of the NIST framework, especially its specificity in implementing controls and policies to better govern AI systems within different organizational contexts.
- Nvidia NeMo Guardrails. This toolkit provides a flexible interface for defining specific behavioral rails that bots need to follow. It supports the Colang modeling language. One chief data scientist said his company uses the open source toolkit to prevent a support chatbot on a lawyer's website from providing answers that might be construed as legal advice.
- Stanford Institute for Human-Centered Artificial Intelligence. The mission of HAI is to provide ongoing research and guidance into best practices for human-centered AI. One early initiative in collaboration with Stanford Medicine is "Responsible AI for Safe and Equitable Health," which addresses ethical and safety issues surrounding AI in health and medicine.
- "Towards Unified Objectives for Self-Reflective AI." Authored by Matthias Samwald, Robert Praas and Konstantin Hebenstreit, this paper takes a Socratic approach to identify underlying assumptions, contradictions and errors through dialogue and questioning about truthfulness, transparency, robustness and alignment of ethical principles. One goal is to develop AI metasystems in which two or more component AI models complement, critique and improve their mutual performance.
- World Economic Forum's "The Presidio Recommendations on Responsible Generative AI." These 30 action-oriented recommendations help to navigate AI complexities and harness its potential ethically. This white paper also includes sections on responsible development and release of generative AI, open innovation, international collaboration and social progress.
These organizations provide a range of resources that serve as a solid foundation for developing ethical AI practices. Using these as a framework can help guide organizations to ensure they develop and use AI in an ethical way.
The future of ethical AI
Some argue that an AI code of ethics can quickly become outdated and that a more proactive approach is required to adapt to a rapidly evolving field. Arijit Sengupta, founder and CEO of Aible, an AI development platform, said, "The fundamental problem with an AI code of ethics is that it's reactive, not proactive. We tend to define things like bias and go looking for bias and trying to eliminate it -- as if that's possible."
A reactive approach can have trouble dealing with bias embedded in the data. For example, if women haven't historically received loans at the appropriate rate, that gets woven into the data in multiple ways. "If you remove variables related to gender, AI will just pick up other variables that serve as a proxy for gender," Sengupta said.
He believes the future of ethical AI needs to talk about defining fairness and societal norms. So, for example, at a lending bank, management and AI teams would need to decide whether they want to aim for equal consideration, such as loans processed at an equal rate for all races; proportional results, where the success rate for each race is relatively equal; or equal impact, which ensures a proportional amount of loans goes to each race. The focus needs to be on a guiding principle rather than on something to avoid, Sengupta argued.
Most people agree that it's easier and more effective to teach children what their guiding principles should be rather than to list out every possible decision they might encounter and tell them what to do and what not to do. "That's the approach we're taking with AI ethics," Sengupta said. "We are telling a child everything they can and cannot do instead of providing guiding principles and then allowing them to figure it out for themselves."
For now, humans develop rules and technologies that promote responsible AI. Shepherd said this includes programming products and offers that protect human interests and aren't biased against certain groups, such as minorities, those with special needs and the poor. The latter is especially concerning, as AI has the potential to spur massive social and economic warfare, furthering the divide between those who can afford technology -- including human augmentation -- and those who can't.
Society also urgently needs to plan for the unethical use of AI by bad actors. Today's AI systems range from fancy rules engines to ML models that automate simple tasks to generative AI systems that mimic human intelligence. "It might be decades before more sentient AIs begin to emerge that can automate their own unethical behavior at a scale that humans wouldn't be able to keep up with," Shepherd said. But, given the rapid evolution of AI, now is the time to develop the guardrails to prevent this scenario.
The future might see an increase in lawsuits, such as the one The New York Times brought against OpenAI and Microsoft in relation to the use of Times articles as AI training data. OpenAI has also found itself in more controversies that might influence the future, such as its consideration of moving toward a for-profit business model. Elon Musk has also announced an AI model that sparked controversy in both the images it can create and the environmental and social impact of the physical location in which it's being placed. The future of AI might continue to see this pushing of ethical boundaries by AI companies, as well as pushback on ethical, social and economic concerns and a call for more ethical implementations by governments and citizens.
The recent advancements of generative AI have also pushed the topic of how to implement these systems ethically. Learn more about AI ethics and generative AI.