AI transparency: What is it and why do we need it?
As AI adoption has increased, the concept of AI transparency has broadened in scope and grown in importance. Learn what it means for enterprise AI teams.
What is AI transparency?
AI transparency is the broad ability to understand how AI systems work, encompassing concepts such as AI explainability, governance and accountability. This visibility into AI systems ideally is built into every facet of AI development and deployment, from understanding the machine learning model and the data it is trained on, to understanding how data is categorized and the frequency of errors and biases, to the communications among developers, users, stakeholders and regulators.
These multiple facets of AI transparency have come to the forefront as machine learning models have evolved and especially with the advent of generative AI (GenAI), a type of AI that can create new content, such as text, images and code. A big concern is that the more powerful or efficient models required for such sophisticated outputs are harder -- if not impossible -- to understand since the inner workings are buried in a so-called black box.
"Basically, humans find it hard to trust a black box -- and understandably so," said Donncha Carroll, partner and chief data scientist at business transformation advisory firm Lotis Blue Consulting. "AI has a spotty record on delivering unbiased decisions or outputs."
Misconceptions about AI transparency
AI transparency is sometimes construed narrowly as being achievable through source code disclosure, said Bharath Thota, partner in the digital and analytics practice at management consulting firm Kearney.
This article is part of
What is enterprise AI? A complete guide for businesses
But he said this limited view is built on the outdated belief that algorithms are objective and overlooks the complexities of AI systems, where transparency must encompass not just the visibility of the code, but the interpretability of model decisions, the rationale behind those decisions and the broader sociotechnical implications. Additionally, IT leaders must carefully evaluate the implications of stringent data privacy regulations, such as GDPR and the EU AI Act, and ensure that AI systems are used ethically to maintain stakeholder trust.
For example, merely revealing the source code of a machine learning model does not necessarily explain how it arrives at certain decisions, especially if the model is complex, like a deep neural network.
"Transparency should, therefore, include clear documentation of the data used, the model's behavior in different contexts and the potential biases that could affect outcomes," Thota said.
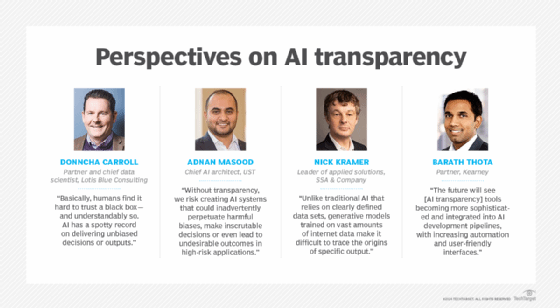
Why is AI transparency important?
Like any data-driven tool, AI algorithms depend on the quality of data used to train the AI model. The algorithms are subject to bias in the data and, therefore, have some inherent risk associated with their use. Transparency is essential to securing trust from users, regulators and those affected by algorithmic decision-making.
"AI transparency is about clearly explaining the reasoning behind the output, making the decision-making process accessible and comprehensible," said Adnan Masood, chief AI architect at digital transformation consultancy UST and a Microsoft regional director. "At the end of the day, it's about eliminating the black box mystery of AI and providing insight into the how and why of AI decision-making."
The need for AI trust, auditability, compliance and explainability are some of the reasons transparency is becoming an important discipline in the field of AI.
"Without transparency, we risk creating AI systems that could inadvertently perpetuate harmful biases, make inscrutable decisions or even lead to undesirable outcomes in high-risk applications," Masood said.
GenAI complicates transparency
The rise of generative AI models has put a spotlight on AI transparency -- and growing pressure on companies that plan to use it in their business operations. Enterprises preparing for generative AI need to improve data governance around the unstructured data that these large language models (LLMs) work with.
"Generative AI models are often much larger and more complex than traditional AI systems, making them inherently harder to interpret," said Nick Kramer, leader of applied solutions at global consulting firm SSA & Company.
Decision-making processes are less transparent in generative AI models due to the intricate interactions within the massive neural networks used to create them. These models can exhibit unexpected behaviors or capabilities not explicitly programmed, raising questions about how to ensure transparency for features that weren't anticipated by the developers. In some cases, generative AI can produce convincing but false information, further complicating the notion of transparency. It's challenging to provide transparency into why a model might generate incorrect information.
AI transparency can also be complicated by LLMs that have limited visibility into their training data, which, as noted, can introduce biases or affect algorithmic decision-making.
"Unlike traditional AI that relies on clearly defined data sets, generative models trained on vast amounts of internet data make it difficult to trace the origins of specific outputs," Kramer said. And, on the front end, the role of prompts in shaping outputs introduces a new layer of complexity in understanding how these systems arrive at their responses.
One confounding factor for enterprises has been that, as the commercial value of GenAI rises, many vendors have increased the secrecy around their architectures and training data, potentially in conflict with their stated transparency goals. Also, the conversational nature of many generative AI applications creates a more personal experience for the user, potentially leading to overreliance or a misunderstanding of the AI's capabilities, Kramer said.
Transparency vs. explainability vs. interpretability vs. data governance
AI transparency is built across many supporting processes. The aim is to ensure that all stakeholders can clearly understand the workings of an AI system, including how it makes decisions and processes data.
"Having this clarity is what builds trust in AI, particularly in high-risk applications," Masood said. The following are some important aspects of transparent AI:
- Explainability refers to the ability to describe how the model's algorithm reached its decisions in a way that is comprehensible to nonexperts.
- Interpretability focuses on the model's inner workings, with the goal of understanding how its specific inputs led to the model's outputs.
- Data governance provides insight into the quality and suitability of data used for training and inference in algorithmic decision-making.
While explainability and interpretability are crucial in achieving AI transparency, they don't wholly encompass it. AI transparency also involves being open about data handling, the model's limitations, potential biases and the context of its usage.
Data transparency key to AI transparency
Data transparency is foundational to AI transparency, as it directly affects the trustworthiness, fairness and accountability of AI systems. Ensuring transparency in data sources means clearly documenting where data originates, how it has been collected and any preprocessing steps it has undergone, a crucial element of identifying and mitigating potential biases.
"As AI technologies advance, there will likely be significant developments in tools that enhance data lineage tracking, as well as algorithmic transparency," Thota said.
These tools will enable organizations to trace data flows from source to outcome, ensuring that every step of the AI decision-making process is auditable and explainable. This will be increasingly important for meeting regulatory requirements and for maintaining public trust in AI systems, particularly as they are used in more sensitive and impactful applications.
Techniques for achieving AI transparency
Different types of tools and techniques can support various aspects of AI transparency, Thota explained. Here are some of the tools he noted that have contributed to the advancement of AI transparency:
- Explainability tools, like local interpretable model-agnostic explanations and SHapley Additive exPlanations, help explain model predictions.
- Fairness toolkits, like IBM AI Fairness 360 and Google's Fairness Indicators, assess and mitigate biases in AI systems.
- Auditing frameworks, like the Institute of Internal Auditors' AI Auditing Framework, enable automated compliance checks, ensuring that AI systems meet transparency and ethical standards.
- Data provenance tools track the origin, history and transformations of data, which is critical for ensuring the reliability and integrity of AI outputs.
- Algorithmic explainability makes the decision-making process of AI models interpretable and understandable for nontechnical stakeholders.
- Documentation of AI systems, coupled with adherence to ethical guidelines, ensures AI-driven decisions are transparent and accountable.
"The future will see these tools [and techniques] becoming more sophisticated and integrated into AI development pipelines, with increasing automation and user-friendly interfaces that democratize AI transparency efforts across industries," Thota said.
Regulation requirements for AI transparency
The future of AI transparency lies in creating comprehensive standards and regulations that enforce transparency practices, ensuring that AI systems are explainable, accountable and ethically sound. Thota said that IT decision-makers need to consider several key regulatory frameworks that mandate clear guidelines for AI transparency, fairness and accountability, including the following:
- EU AI Act.
- GDPR.
- President Biden's executive order on AI.
"The integration of AI transparency into corporate governance and regulatory compliance will be crucial in shaping a trustworthy AI ecosystem, ensuring that AI systems align with ethical norms and legal requirements," Thota said.
Tradeoffs and weaknesses of AI transparency
IT decision-makers need to consider how to weigh the tradeoff between accuracy and transparency in AI systems. Some of the cutting-edge machine learning and AI models can improve accuracy, but their superior performance can come at the cost of reduced transparency.
These black box models show better accuracy in general, but in the instances when they do go wrong, it can be difficult to identify where and how the mistakes were made.
AI transparency, it's important to note, can also cause problems, including the following:
- Hacking. AI models that show how and why a decision was made could empower bad actors. For example, attackers could use this information to take advantage of weaknesses and hack into systems.
- Difficulty. Transparent algorithms can be harder to design, in particular for complex models with millions of parameters. In cases where transparency in AI is a must, it might be necessary to use less sophisticated algorithms.
- Governance. Increased AI transparency can sometimes increase the risk of compromising users' and stakeholders' personal information.
- Reliability. Efforts to increase AI transparency can sometimes generate different results each time they are performed. This can reduce trust and hinder transparency efforts.
Transparency is not a one-and-done project. As AI models continuously learn and adapt to new data, they must be monitored and evaluated to maintain transparency and ensure that AI systems remain trustworthy and aligned with intended outcomes.
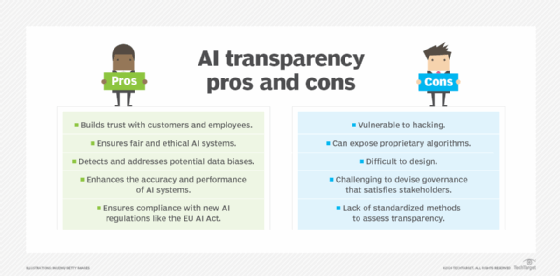
How to reach a balance between accuracy and AI transparency
As with any other computer program, AI needs optimization. To do that, AI developers look at the specific needs of a problem and then tune the general model to best fit those needs. When implementing AI, an organization must pay attention to the following four factors:
- Legal pressures. Regulatory explainability requirements may specify various transparency requirements that increase the need for simpler and more explainable algorithms.
- Severity of impact on people. AI used for life-critical decisions usually increases the need for greater AI transparency. For less critical decisions, such as fraud identification algorithms, opaque algorithms sometimes yield more accurate results, and in cases like these, a bad decision by an opaque algorithm might inconvenience users but is likely not to have a significant adverse impact on them.
- Exposure. It's also important to consider the reach of AI transparency. For example, increased transparency in cybersecurity might improve processes for users, but it could also embolden hackers to adapt attacks to bypass detection.
- Data set. AIs trained on existing data can sometimes accentuate existing systemic racism, sexism and other kinds of biases. It's important to assess these issues and balance inputs to reduce bias in a way that can improve a model's accuracy for all users and stakeholders.
Best practices for implementing AI transparency
Implementing AI transparency, including finding a balance between competing organizational aims, requires ongoing collaboration and learning between leaders and employees. It calls for a clear understanding of the requirements of a system from a business, user and technical point of view.
The tendency in AI development is to focus on features, utility and novelty, rather than on safety, reliability, robustness and potential harm, Masood said. He recommended prioritizing transparency from the inception of the AI project. It's helpful to create datasheets for data sets and model cards for models, implement rigorous auditing mechanisms and continuously study the potential harm of models.
Key use cases for AI transparency
AI transparency has many facets, so teams must identify and examine each of the potential issues standing in the way of transparency. Thota recommended that IT decision-makers consider the broad spectrum of transparency aspects, including data provenance, algorithmic explainability and effective stakeholder communication.
Here are some of the various aspects of transparency to consider:
- Process transparency audits decisions across development and implementation.
- System transparency provides visibility into AI use, such as informing users when engaged with an AI chatbot.
- Data transparency provides visibility into data used to train AI systems.
- Consent transparency informs users how their data might be used across AI systems.
- Model transparency reveals how AI systems function, possibly by explaining decision-making processes or by making the algorithms open source.
The future of AI transparency
AI transparency is a work in progress as the industry discovers new problems and better processes to mitigate them.
Lotis Blue's Carroll predicted that insurance premiums in domains where AI risk is material could also shape the adoption of AI transparency efforts. These will be based on an organization's overall systemic risk and evidence that best practices have been applied in model deployment.
Masood believes regulatory frameworks likely will play a vital role in the adoption of AI transparency. For example, the EU AI Act highlights transparency as a critical aspect. This is indicative of the shift toward more transparency in AI systems to build trust, facilitate accountability and ensure responsible deployment.
"For professionals like myself, and the overall industry in general, the journey toward full AI transparency is a challenging one, with its share of obstacles and complications," Masood said.
"However, through the collective efforts of practitioners, researchers, policymakers and society at large, I'm optimistic that we can overcome these challenges and build AI systems that are not just powerful, but also responsible, accountable and, most importantly, trustworthy," he said.
Kramer expects the market for AI transparency tools to evolve across transparency, evaluation and governance methods. For example, since newer generative AI models are designed to be different every time, there will be increased emphasis on evaluating the quality, safety and biases in the outputs rather than trying to achieve full transparency of the inner workings. The opacity of these models will drive interest in probabilistic transparency into the model's behavior.
Indeed, Thota predicted a rise of dynamic transparency frameworks that can adapt to emerging technologies and evolving regulatory landscapes. These will account for how contextual factors, such as the training data, model architecture and human oversight, shape AI outcomes. This shift will emphasize the importance of fairness, accountability and explainability, moving beyond the notion of transparency as just a technical requirement to include ethical and societal dimensions as well.
"As AI systems become more complex, transparency will evolve to include advanced tools for model interpretability, real-time auditing and continuous monitoring," Thota said. These developments will be driven by technological advancements and increasing regulatory pressures, solidifying transparency as a central pillar in the responsible deployment of AI.
Editor's note: This article was originally published in June 2023 and updated to explain how the concept of AI transparency has evolved with the increased use of AI systems in business.
George Lawton is a journalist based in London. Over the last 30 years, he has written more than 3,000 stories about computers, communications, knowledge management, business, health and other areas that interest him.