What is synthetic data? Examples, use cases and benefits
Synthetic data is information that's artificially manufactured rather than generated by real-world events. It's created algorithmically and is used as a stand-in for test data sets of production or operational data to validate mathematical models and train machine learning (ML) and deep learning models.
Gathering high-quality data from the real world is difficult, expensive and time-consuming. However, synthetic data technology lets users quickly, easily and digitally generate data in whatever amount they desire, customized to their needs.
Synthetic data dates to the advent of computing in the 1970s. Most initial systems and algorithms depended on data to function. However, restricted processing capacity, challenges in collecting vast volumes of data and privacy concerns led to the creation of synthetic data.
In the wake of the ImageNet competition of 2012 -- commonly referred to as the Big Bang of artificial intelligence (AI) -- Geoff Hinton led a group of researchers in successfully training an artificial neural network to win an image classification challenge with a startlingly large margin. Researchers began looking for artificial data once it was revealed that neural networks could recognize items faster than humans.
This article is part of
What is GenAI? Generative AI explained
Why is synthetic data important?
The use of synthetic data is gaining acceptance because it can provide several benefits over real-world data. Gartner predicts that, by 2030, synthetic data will eclipse real data used for developing AI models.
The largest application of synthetic data is in the training of neural networks and ML models, as developers need carefully labeled data sets that range from a few thousand to tens of millions of items. Synthetic data can be artificially generated to mimic real data sets, letting companies create a large amount of diverse training data without spending a lot of money and time. According to Paul Walborsky, a co-founder of AI.Reverie, one of the first dedicated synthetic data services, now owned by Meta, a single image that would cost $6 from a labeling service could be artificially generated for 6 cents.
Synthetic data is also used to protect user privacy and comply with privacy laws, particularly when dealing with sensitive health and personal data. Additionally, it can ensure customers have access to diverse data that accurately depicts the real world, reducing bias in data sets.
How is synthetic data generated?
The process of generating synthetic data differs depending on the tools and algorithms used and the specific use cases. The following are three common techniques used for creating synthetic data:
- Drawing numbers from a distribution. Randomly selecting numbers from a distribution is a common method for creating synthetic data. Although this method doesn't capture the insights of real-world data, it can produce a data distribution that closely resembles real-world data.
- Agent-based modeling. This simulation technique involves creating unique agents that communicate with one another. These methods are especially helpful when examining how different agents -- such as mobile phones, people and even computer programs -- interact with one another in a complex system. Using prebuilt core components, Python packages such as Mesa make it easier to quickly develop agent-based models and view them in a browser-based interface.
- Generative models. These algorithms generate synthetic data that replicates the statistical properties or features of real-world data. Generative models learn the statistical patterns and relationships in training data and then use this knowledge to generate new synthetic data that's similar to the original data. Examples of generative AI models include generative adversarial networks and variational autoencoders.
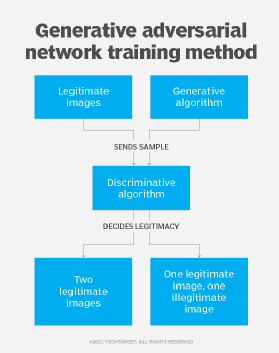
What are the advantages of synthetic data?
Synthetic data offers the following advantages:
- Customizable data. An organization can customize synthetic data to its needs, tailoring the data to conditions that can't be obtained with authentic data. They can also generate data sets for software testing and data quality assurance (QA) purposes for DevOps teams.
- Cost-effective data. Synthetic data is an inexpensive alternative to real-world data. For example, real vehicle crash data can cost an automaker more to collect than simulated data.
- Data labeling. Even when synthetic data is available, it isn't always labeled. For supervised learning tasks, manually labeling a multitude of instances can be time-consuming and error-prone. Synthetically labeled data can speed up the model development process. Additionally, it guarantees labeling accuracy.
- Faster production. Because synthetic data isn't gathered from actual events, it's possible to create a data set faster with the right software and technology. As a result, a significant amount of artificial data is created in a shorter amount of time.
- Complete annotation. Perfect annotation eliminates the need for manual data collection. Each object in a scene automatically creates a variety of annotations. This is one of the main reasons synthetic data is so inexpensive when compared to real data.
- Data privacy. While synthetic data can resemble real data, it shouldn't contain any information that could be used to identify the real data. This characteristic makes synthetic data anonymous and suitable for dissemination and can be a major part of data optimization for the healthcare and pharmaceutical industries.
- Full user control. Synthetic data simulation enables complete control over every aspect. The person handling the data set controls event frequency, item distribution and many other factors. ML practitioners also have control over the data set when using synthetic data. Some examples include controlling the degree of class separations, sample size and noise level in the data set.
Synthetic data also comes with drawbacks, including inconsistencies when trying to replicate the complexity found in the original data set. Another challenge is the inability to replace authentic data outright, as accurate, authentic data is still required to produce useful synthetic examples of the information.
What are the use cases for synthetic data?
Synthetic data should appropriately reflect the original data that it strives to improve. Typical use cases for synthetic data include the following:
- Testing. Synthetic test data is easier to create than rules-based test data and offers flexibility, scalability and realism. It's crucial for data-driven testing and software development.
- AI and ML model training. Synthetic data is increasingly used to train AI models. It often outperforms real-world data and is essential for developing superior AI models. Synthetic training data enhances model performance, eliminating bias and adding fresh domain knowledge and explainability. Besides being completely privacy-compliant, it also enhances the original data thanks to the nature of the AI-powered synthetization process. For example, in artificial training data, uncommon patterns and occurrences can be upsampled, where new data is added to data sets to address imbalances.
- Privacy regulations. Synthetic data helps data analysts adhere to data privacy laws, such as the Health Insurance Portability and Accountability Act, General Data Protection Regulation and California Consumer Privacy Act. It's also the best option when using sensitive data sets for testing or training. Synthetic data provides insight without jeopardizing privacy compliance.
- Healthcare and other private data. Health and privacy data are particularly appropriate for a synthetic approach because privacy rules place significant restrictions on these fields. By using synthetic data, researchers can extract the information they need without invading people's privacy. Because synthetic data doesn't represent the data of actual patients, it's unlikely that it will result in the identification of an actual patient or their personal data record. Synthetic data also has an advantage over data masking techniques, which pose greater privacy-related risks.
What are examples of synthetic data?
Synthetic data is used across many different industries for various use cases. The following are some examples of synthetic data applications:
- Media data. In this use case, computer graphics and image processing algorithms are used to generate synthetic images, audio and video. For example, Amazon uses synthetic data to train Amazon Alexa's language system.
- Text data. This can include chatbots, machine translation algorithms and sentimental analysis based on artificially generated text data. ChatGPT is an example of a tool that uses text data.
- Tabular data. This consists of synthetically generated data tables used for data analysis, model training and other applications.
- Unstructured data. Unstructured data can include images, video and audio data that are mostly used in fields such as machine vision, speech recognition and autonomous vehicle technology. For example, Google's Waymo uses synthetic data to train its self-driving cars.
- Financial services data. The financial sector relies heavily on synthetic data for fraud prevention, risk management and credit risk assessments. For example, American Express uses synthetic financial data to improve fraud detection.
- Manufacturing data. The manufacturing industry uses synthetic data for quality control testing and predictive maintenance. For instance, the German insurance company Provinzial tests synthetic data for predictive analytics.
For more on generative AI, read the following articles:
Pros and cons of AI-generated content
AI content generators to explore
Top generative AI benefits for business
Assessing different types of generative AI applications
Generative AI challenges that businesses should consider
Generative AI ethics: biggest concerns
Generative AI landscape: Potential future trends
Real-world industry examples of synthetic data
Financial services and healthcare are two industries that use synthetic data techniques to manufacture data with attributes similar to actual sensitive or regulated data. This lets data professionals use and share data more freely.
For example, healthcare data professionals use synthetic data to enable public use of record-level data while still maintaining patient confidentiality.
In the financial sector, synthetic data sets -- such as debit and credit card payments -- look and act as typical transaction data to help expose fraudulent activity. Data scientists can use synthetic data to test or evaluate fraud detection systems, as well as develop new fraud detection methods. Synthetic financial data sets are found on Kaggle, a crowdsourced platform that hosts predictive modeling and analytics competitions.
DevOps teams use synthetic data for software testing and QA. They can plug artificially generated data into a process without taking authentic data out of production. However, some experts recommend DevOps teams choose data masking techniques over synthetic data techniques because production data sets contain complex relationships that make it hard to manufacture an accurate representation quickly and cheaply.
Real vs. mock data
Real and mock data differ from synthetic data in the way they are used and generated.
Real data
Real data is the best option for training models to make effective predictions. However, real data isn't always available to a business in need of training data. Also, training a model with real data that contains sensitive information might violate data privacy regulations. Synthetic data that closely mimics real data acts as a substitute when real data is lacking and prevents issues with sensitive data.
Mock data
Mock data sets are created using simple placeholder values and aren't meant to possess any properties or insights that real data sets do. They don't require complicated tools, such as neural networks, to generate them.
An experienced data scientist can easily create mock data for testing and training prototype models to see if real model versions will work correctly. Complex data and relationships between data points aren't necessary, and mock data sets with placeholder values are sufficient.
Synthetic data and machine learning
Synthetic data is gaining traction in the machine learning domain. ML algorithms are trained using an immense amount of data, and collecting the necessary amount of labeled training data can be cost-prohibitive.
Synthetically generated data helps companies and researchers build data repositories needed to train and even pretrain ML models. This technique is referred to as transfer learning.
Data science research efforts to advance synthetic data use in ML are underway. For example, members of the Data to AI Lab at the Massachusetts Institute of Technology documented the successes it had with its Synthetic Data Vault. It can construct machine learning models to automatically generate and extract their own synthetic data.
Companies are also experimenting with synthetic data techniques. For example, Google Deepmind used synthetic data to train an AI system called AlphaGeometry to solve complex geometry problems. Computer vision, image recognition and robotics are other applications that are benefiting from the use of synthetic data.
Synthetic data tools and technologies
As AI model use expands across various industries, the market for synthetic data generation tools is growing as well. Various tools are available.
Enterprises also can use various methods and tools to create their own synthetic data. These methods include the following:
- Large language models. Organizations can train LLMs, such as GPT models, using their own data sets to create synthetic data.
- Generative adversarial networks. The GAN approach involves two neural networks: a generative network and a discriminative network. The generative network is trained to generate synthetic yet realistic data, and the discriminative network attempts to distinguish real from synthetic data. The generative network continuously improves at generating realistic data when attempting to fool the discriminative network.
- Variational autoencoders. VAEs take real data sets, encode them into a compressed format and then decode them into synthetic data sets that mimic the characteristics of the real data sets.
- Statistical distribution. If data scientists understand the statistical distribution of a real data set, they can manually create a similar synthetic data set without using other tools.
Machine learning can use synthetic data to remove bias, democratize data, enhance privacy and reduce costs. Learn how synthetic data can solve problems of ML bias and privacy.