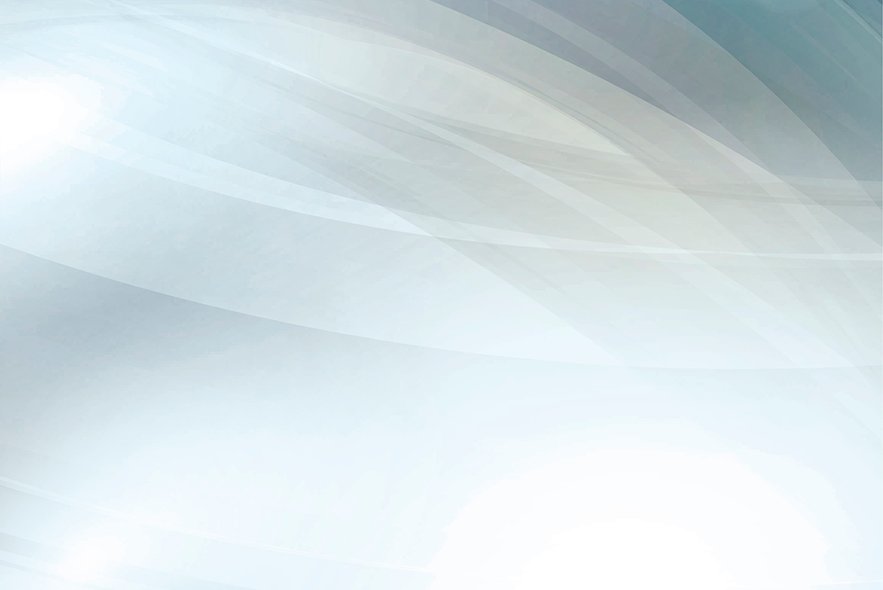
What is data labeling?
Data labeling is the process of identifying and tagging data samples commonly used in the context of training machine learning (ML) models. The process can be manual, but it's usually performed or assisted by software. Data labeling helps machine learning models make accurate predictions. It's also useful in processes such as computer vision, natural language processing (NLP) and speech recognition.
The process starts with raw data, such as images or text data, that's collected, and then one or more identifying labels are applied to each segment of data to specify the data's context in the ML model. The labels used to identify data features must be informative, specific and independent to produce a quality model.
What is data labeling used for?
Data labeling is an important part of data preprocessing for ML, particularly for supervised learning. In supervised learning, a machine learning program is trained on a labeled data set. Models are trained until they can detect the underlying relationship between the input data and the output labels. In this setting, data labeling helps the model process and understand the input data.
For example, a model trained to identify images of animals is provided with multiple images of various types of animals from which it learns the common features of each. This enables the model to correctly identify the animals in unlabeled data.
This article is part of
What is machine learning? Guide, definition and examples
Data labeling is also used when constructing machine learning algorithms for autonomous vehicles, such as self-driving cars. They need to be able to tell the difference between objects that might get in their way. This information lets these vehicles process the external world and drive safely. Data labeling enables the car's artificial intelligence (AI) to tell the differences between a person and another car or between the street and the sky. Key features of those objects or data points get labeled and similarities between them are identified.
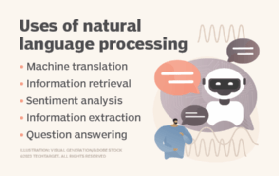
Like supervised ML, computer vision also uses data labeling to help identify raw data. Computer vision deals with how computers interpret digital images and videos.
NLP, which enables a program to understand human language, uses data labeling to extract and organize data from text. Specific text elements are identified and labeled in an NLP model for understanding.
How does data labeling work?
ML and deep learning systems require massive amounts of data to establish a foundation for reliable learning patterns. The data they use to inform active learning must be labeled or annotated based on data features that help the model organize the data into patterns that produce a desired answer.
The data labeling process starts with data collection and then moves on to data tagging and quality assurance (QA). It ends when the model starts training. There are four key steps in the process:
- Data collection. In this step, raw data that's useful to train a model is collected, cleaned and processed.
- Data tagging. In either a manual or software-aided effort, the data is then labeled with one or more tags, which give the ML model context about the data.
- QA. The quality of the machine learning model depends on the quality and the preciseness of the tags. Properly labeled data becomes what's referred to as the ground truth.
- Training. The machine learning model is then trained using the labeled data.
A properly labeled data set provides a ground truth against which the ML model checks its predictions for accuracy and continues refining its algorithm. A high-quality algorithm is high in accuracy, referring to the proximity of certain labels in the data set to the ground truth.
Errors in data labeling impair the quality of the training data set and the performance of any predictive models it's used for. To mitigate this and ensure high performance, many organizations take what's called a human-in-the-loop approach. This is where people are involved in training and testing the data.
Data labeling vs. data classification vs. data annotation
Data labeling, classification and annotation are three methods used to prepare data that's fed to ML models. They're also practical for handling large volumes of raw data in need of organizing. However, these three methods are carried out in different ways and for different purposes.
Data labeling
With this approach, a data expert predefines labels and applies them to each data point within a data set. The process provides a model with the necessary context in its training data to learn from that data and produce outputs, such as insights or predictions.
Data classification
Data classification refers to categorizing data, either categorically or using a binary approach. Successful data classification depends on good data labeling practices. For example, a binary classification task can sort emails, determining if they are spam or not spam, by examining labeled data. Data classification is mainly used in supervised learning when teaching the ML model to make predictions.
Data annotation
Data annotation provides specific information about data inputs, giving ML models a deeper understanding of those inputs. For instance, data annotation can be applied in autonomous vehicle systems where a model must examine many different objects in its surroundings. Annotating different objects with more context and detail enhances the system's understanding of its surroundings.
What are common types of data labeling?
The different types of data labeling are defined by the medium of the data being labeled:
- Image and video labeling. An example of this type of data labeling is a computer vision model, where tags are added to individual images or video frames. This type of image classification is used in healthcare diagnostics, object recognition and automated cars.
- Text labeling. NLP uses this type, adding tags to words for interpretation of human languages. NLP is used in chatbots and sentiment analysis.
- Audio labeling. This type of data labeling is used in speech recognition, where audio segments are broken down and labeled. Audio labeling is useful for voice assistants and speech-to-text transcriptions.
Benefits of data labeling
Benefits that come from data labeling include the following:
- Accurate predictions. If data scientists input properly labeled data, a trained machine learning model can use that data as a ground truth to make accurate predictions when presented with new data.
- Data usability. Developers reduce the number of input variables in order to optimize models in ways that produce better analysis and predications. It's important that input data be labeled in a way that specifies the features and data variables that are most relevant or important for the model to learn. This helps the model focus on the most useful and relevant data and makes it better equipped to perform its assigned tasks.
- Enhanced innovation and profitability. Once a data labeling approach is in place, workers can spend less time on tedious data labeling tasks and instead focus on finding new practical or revenue-generating uses for labeled data.
Challenges of data labeling
A few challenges are evident for businesses tasked with large-scale data labeling:
- Costs. Data labeling can be expensive, especially when done manually. Even with an automated approach, there are upfront costs involved in buying and setting up the necessary tech infrastructure.
- Time and effort. Manual data labeling inevitably takes longer than an automated approach. However, even with automation, employees with the needed expertise divert time from their normal duties to set up the infrastructure.
- Human error. Mistakes happen. For example, data might be mislabeled because of coding or manual entry errors. This could lead to inaccurate data processing, modeling or ML bias.
Best practices for data labeling
Best practices to follow in data labeling include the following:
- Collect diverse data. When collecting data to be labeled, data sets should be as diverse as possible to prevent bias.
- Ensure data is representative. Collected data must be clear and specific if model developers want their models to be accurate.
- Provide feedback. Regular feedback helps ensure the quality of the data labels.
- Create a label consensus. This should measure the agreement rate between both human and machine labelers.
- Audit labels. Audits are done to verify label accuracy.
Methods of data labeling
An enterprise can use several methods to structure and label its data. The options range from using in-house staff to crowdsourcing and data labeling services:
- Crowdsourcing. A third-party crowdsourcing platform gives an enterprise access to many workers at once.
- Outsourcing. An enterprise can hire temporary freelance workers to process and label data.
- Managed teams. An enterprise can enlist a managed team to process data. Managed teams are trained, evaluated and managed by a third-party organization.
- In-house staff. An enterprise can use its existing employees to process data.
- Synthetic labeling. New project data is generated using already existing data sets. This process increases data quality and time efficiency but requires more computing power.
- Programmatic labeling. Scripts are used to automate the data labeling process.
There is no singular optimal method for labeling data. Enterprises should use the method or combination of methods that best suits their needs. Some questions must be considered when choosing a high-quality data labeling method, such as the following:
- Is the business a large enterprise or a small to medium-sized organization?
- What's the size of the data set that requires labeling?
- What's the skill level of employees on staff?
- Are there financial restraints?
- What's the purpose of the ML model being supplemented with labeled data?
A good data labeling team should ideally have domain knowledge of the industry an enterprise serves. Human labelers who have outside context guiding them are more accurate than ones who don't, and they also have diverse perspectives. They must also be flexible and nimble because data labeling and ML are iterative processes, always changing and evolving as more information is taken in.
The importance of data labeling
Spending on AI projects typically goes toward preparing, cleaning and labeling data. Manual data labeling is the most time-consuming and expensive method, but it might be warranted for important applications.
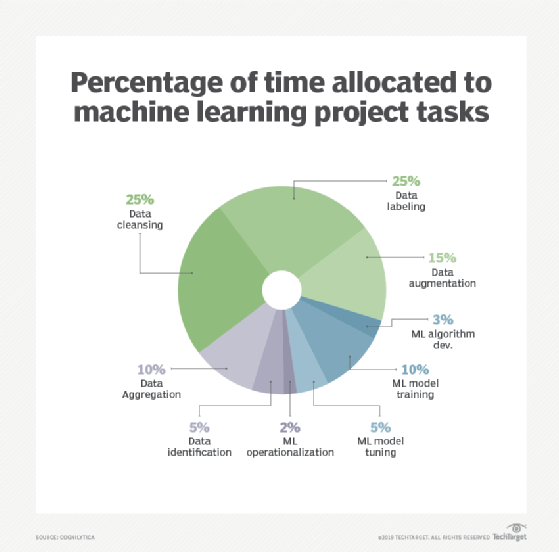
Critics of AI speculate that automation will put low-skill jobs, such as call center work and truck and Uber driving, at risk. It will be easier for machines to perform these types of rote tasks.
However, some experts believe data labeling itself will be a new low-skill job opportunity for people displaced by automation. There will be an ever-growing surplus of data and machines that need to be trained to process that data. Skilled data labelers will be needed to properly label data and train the advanced AI and ML models.
Examples of companies using data labeling
Companies that have incorporated data labeling into their business models include the following:
- Alibaba uses data labeling for its e-commerce platform. Data about customers' purchases is labeled and annotated so it can be fed to an AI model that offers product recommendations to those customers based on that data.
- Amazon uses a similar approach to generate product recommendations to consumers with its AI-powered recommendation engine.
- Facebook. Facebook can take facial images of its users and label them for the purpose of training algorithms to offer tagging suggestions for those photos.
- Microsoft. Data labeling was essential in developing Microsoft Azure services, particularly Azure Machine Learning, when using training data to train the model.
- Autonomous vehicle manufacturers. Tesla developed autonomous capabilities for its vehicles by labeling and annotating objects in the surrounding world. Waymo has done similar model training with its autonomous vehicles, also relying heavily on labeling objects in the physical world.
- Voice assistant developers. Google Assistant, Apple's Siri and Amazon's Alexa were all developed using model training that labeled speech data and audio samples.
Future of data labeling
As advanced technologies, such as AI and ML, become more pervasive across industries for real-world uses, AI-powered data labeling tools are becoming more common. As big data collection becomes more common as well, ML algorithms are fed large training data volumes to learn new information. The algorithms are also expected to become better at categorization or classification. The goal is to efficiently label data volumes so people don't have to do these painstaking tasks.
Other trends include an expansion of crowdsourcing, where workers are pooled to collectively label data. Large data sets benefit from having people with various backgrounds and areas of expertise add detailed information to data points.
Finally, data privacy concerns have grown as AI and ML models are being fed large data sets, often including people's personal data. Therefore, businesses have to be cautious when labeling data to not violate local, state and federal data protection laws and ensure the privacy of data subjects.
Data labeling is a subset of data preprocessing. Learn about various data science tools, including ones used for data preprocessing.