How big data collection works: Process, challenges, techniques
Taming large amounts of data from multiple sources and deriving the greatest value to ensure trusted business decisions hinge on a foolproof system for collecting big data.
Big data has become one of the more valuable assets held by enterprises, and virtually every large organization is making investments in big data initiatives.
That's not an overstatement. A 2021 survey by NewVantage Partners found that 99% of senior C-level executives at Fortune 1000 companies said they're pursuing a big data program. Perhaps even more significant, 96% reported that their companies have had success with their big data and artificial intelligence programs, 92% said the pace of their investments in these areas is accelerating and 81% voiced optimism about the future of big data and AI in their organizations.
What is big data collection?
Big data collection is the methodical approach to gathering and measuring massive amounts of information from a variety of sources to capture a complete and accurate picture of an enterprise's operations, derive insights and make critical business decisions. Data collection is far from new, of course, since information gathering has been an ingrained practice for millennia. Moreover, researchers for centuries have been confounded in their attempts to manage and analyze overwhelming amounts of data.
Big data collection entails structured, semi-structured and unstructured data generated by people and computers. Big data's value doesn't lie in its quantity, but rather in its role in making decisions, generating insights and supporting automation -- all critical to business success in the 21st century.
"Companies need to invest in what the data can do for their business," said Christophe Antoine, vice president of global solutions engineering at data integration platform provider Talend. But organizations that want to reap the benefits of big data must first effectively collect it -- not so easy a feat given the volume, variety and velocity of data today.
What data is collected?
Today the volume, variety and velocity of data are so much greater that it warrants the title big data. The world now generates an estimated 2.5 quintillion bytes of data every day, according to general consensus statistics. This data comes in the following three forms:
- Structured data is highly organized and exists in predefined formats like credit card numbers and GPS coordinates.
- Unstructured data exists in the form it was generated, such as social media posts.
- Semi-structured data is a mix of structured and unstructured data like email addresses and text, respectively.
Data generally can be classified as quantitative and qualitative. Quantitative data comes in numerical form such as statistics and percentages, while qualitative data carries descriptive characteristics like color, smell, appearance and quality. In addition to the primary data, organizations might use secondary data collected by another party for a different purpose.
Common methods of collecting big data
In big data collection, the range of a company's sources generating data needs to be identified. Typical sources include the following:
- Operational systems producing transactional data such as point-of-sale software.
- Endpoint devices within IoT ecosystems.
- Second- and third-party sources such as marketing firms.
- Social media posts from existing and prospective customers.
- Multiple additional sources like smartphone locational data.
- Surveys that directly ask customers for information.
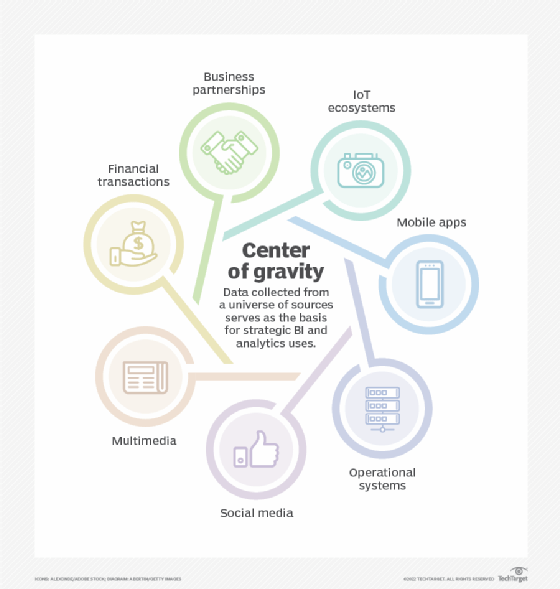
No enterprise can collect and use all the data being created. So, business leaders need to build a big data collection program that identifies the data they need for their existing and future business use cases. Some experts believe enterprises should collect as much data as they can acquire to pilot innovative use cases, while others advise organizations to be more selective to avoid running up costs, complexity and compliance issues without getting any business value in return.
Steps in the data collection process
Identifying useful data sources is just the start of the big data collection process. From there, an organization must build a pipeline that moves data from generation to enterprise locations where the data will be stored for organizational use. Most commonly, this data ingestion process involves three overarching steps -- extract, transform and load (ETL):
- Extraction -- data is taken from its originating location.
- Transformation -- data is cleansed and normalized for business use.
- Loading -- data is moved into a database, data warehouse or data lake to be accessed for use.
Data management teams face additional considerations and requirements at each of these steps, such as how to ensure the data they've identified for use is reliable and how to prepare it for use.
"Data determines the uses you can have, and desired applications determine the data you will need," said David Belanger, senior research fellow at the Stevens Institute of Technology School of Business and retired chief scientist at AT&T Labs. "Once you know the sources, there are a number of questions to be answered: Where can I get the data I need? Is the source reliable? What are its properties, for example, velocity, stream, transaction, purchased? What is its quality? Is it internally or externally sourced? etc."
Challenges in big data collection
Not surprisingly, many businesses struggle with these questions. "There are all kinds of challenges -- technical challenges, organizational and sometimes compliance challenges," said Max Martynov, CTO at digital transformation service provider Grid Dynamics. These challenges can include the following:
- Identifying and managing all the data held by an organization.
- Accessing all the required data sets and breaking down internal and external data silos.
- Achieving and maintaining good data quality.
- Selecting and properly using the right tools for the various ETL tasks.
- Having the right skills and enough skilled talent for the level of work required to meet organizational objectives.
- Properly securing all the collected data and adhering to privacy and security regulations while enabling access to meet business needs.
Such challenges within the data collection process mirror the challenges that executives cite as barriers to developing their big data initiatives overall. The NewVantage study, for example, found that 92% of respondents identified culture -- people, business processes, change management -- as the biggest challenge to becoming a data-driven organization, while just 8% identified technology limitations as the leading barrier.
Big data security and privacy issues
Experts advise business leaders to develop a strong data governance program to help address those challenges, particularly security- and privacy-related challenges. "You don't want to hurt access, but you do need to put the right governance in place to protect your data," Talend's Antoine noted.
A good governance program should establish the processes needed to dictate how the data is collected, stored and used and ensure that the organization does the following:
- Identifies regulated and sensitive data.
- Establishes controls to prevent unauthorized access to it.
- Creates controls to audit those who access it.
- Creates systems to enforce governance rules and protocols.
Such steps help secure and protect data to ensure regulatory compliance. Moreover, experts said these measures help the business to trust its data -- an important part of becoming a data-driven organization.
Best practices for collecting big data
To build a successful, secure process for big data collection, experts offered the following best practices:
- Develop a framework for collection that includes security, compliance and governance from the start.
- Build a data catalog early in the process to know what's in the organization's data platform.
- Let business use cases determine the data that's collected.
- Tune and tweak data collection and data governance as use cases emerge and the data program matures, identifying what data sets are missing from the organization's big data collection process and what collected data sets hold no value.
- Automate the process as much as possible from data ingestion to cataloging to ensure efficiency and speed as well as adherence to the protocols established by the governance program.
- Implement tools that uncover problems in the data collection process, such as data sets that don't show up as expected.