The future of generative AI: 10 trends to follow in 2025
Dig into trends and products that will contribute to GenAI's development and the technology's impact on how your organization will operate in the coming year.
Multiple reports show that enterprise leaders expect their generative AI investments to deliver measurable value this year, a clear indication that the days of experimenting with GenAI for learning purposes alone are over.
For example, 75% of the 1,803 C-level executives polled by the Boston Consulting Group (BCG) for its "AI Radar" global survey list AI/GenAI among their top three strategic priorities for 2025.
And organizations are backing up that sentiment with money. GenAI budgets will grow 60% from 2025 to 2027, expanding from an average 4.7% of total IT budgets to approximately 7.6% by 2027, according to BCG's July 2024 "7th IT Spending Pulse Survey" of 330 IT buyers conducted with global consulting firm GLG.
For some organizations, that translates into significant dollars.
Professional services firm KPMG found that 68% of respondents to its "AI Quarterly Pulse Survey," released in January 2025, plan to invest between $50 million and $250 million in GenAI over the next year, up from the 45% planning such investments a year ago.
This article is part of
What is GenAI? Generative AI explained
Here are 10 GenAI trends for 2025 -- and the GenAI tools that will help power them.
1. Executives seek ROIs from GenAI investments
Organizations have been experimenting with GenAI ever since ChatGPT arrived on the scene in late 2022, but those experiments have yielded mixed results in terms of delivering returns.
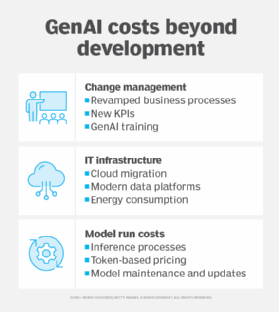
Now, the pressure is on to demonstrate solid ROIs. "We're into 'Show me the money, show me the value, show me the return on my generative AI investments,'" said Brian Jackson, principal research director at Info-Tech Research Group. "GenAI is becoming more like any other service or infrastructure that's been bought, where it's expected to produce value."
Organizations are well on their way. "The ROI of Gen AI," a report conducted in fall 2024 by the National Research Group and commissioned by Google Cloud, found that 74% of respondent enterprises using GenAI are seeing ROIs, with another 35% or so anticipating ROIs within the next 12 months.
The 2,500 executive-level business leaders surveyed for the report listed increased employee productivity as well as improved user experience, user engagement and user satisfaction as the most common measurable benefits they've seen.
2. GenAI initiatives will increasingly add a bottom-up approach
Executives have made GenAI a top priority and are doing what they can to drive GenAI initiatives in their organizations. However, just as much value will come from everyday workers' ability to use the technology to help them do their jobs faster and better, Jackson said.
"There is a mistake that can be made in terms of pursuing ROI with GenAI with too much of a top-down approach," he explained. "That's one way we're going to unlock the value. But another way we'll get value from AI is by putting it in the hands of employees. The workers who are close to the work are often the ones coming up with the ways that GenAI can be useful."
3. GenAI will make everyone a programmer
Workers will use GenAI to help them deploy the technology, Jackson said. They'll increasingly be able to instruct GenAI tools in everyday language to create GenAI programs and other software code to automate and streamline tasks.
"Generative AI is turning everyone into a programmer," he said. "That's huge. We shouldn't miss that value here."
That doesn't happen by chance. Jackson said executives -- particularly CIOs -- need to educate their organization's workforce on how to use GenAI and "go out to the lines of business and demonstrate to them how this could be useful to them, how they should imagine the use cases, and show them this is what we should be doing with generative AI."
4. Organizations and executives will build trust in GenAI systems
As GenAI becomes pervasive, building trust in these systems is crucial, said Manjeet Rege, professor and chair of the software engineering and data science department at the University of St. Thomas.
Rege said he sees organizations adopting the following strategies to foster trust:
- Transparency to provide clear explanations of GenAI decision-making processes and data sources.
- Explainability to develop techniques to interpret and understand GenAI-driven outcomes.
- Accountability to establish clear lines of responsibility for GenAI-related decisions and actions.
Rege said enterprise leaders are also implementing continuous monitoring to regularly review and update GenAI systems so they remain fair, accurate and unbiased -- a move that further builds trust.
5. Organizations will deploy more tailored, customized and vertical GenAI apps
Organizations have been moving away from the plug-and-play approach they had when ChatGPT debuted and instead deploying GenAI "in more customized fashion," said Sagar Samtani, an associate professor at Indiana University's Kelley School of Business and director of its Data Science and Artificial Intelligence Lab.
"So, what's happened, and what we'll see more [organizations] do in 2025, is more vertical applications of training models," Samtani explained.
Others also cited organizations using GenAI engines for organization-, industry- and workflow-specific applications, with large language models (LLMs) trained on data gleaned from those respective areas delivering those highly customized and targeted use cases.
For example, think of GenAI that has been trained on a financial firm's data to help its investors choose investments, said Vivek Mishra, senior member at the professional association IEEE. Or, he said, it might be a company training an LLM on its own unique processes to create an agent to coach employees on workflows.
6. Agentic AI will become commonplace
The push to deploy GenAI agents, also known as agentic AI, will be a significant focus for many this year, according to Vlad Lukic, global leader of BCG's tech and digital advantage practice. Agentic AI is a type of AI in which systems work independently and without direct human intervention.
And BCG research backs that trend: 67% of respondents to its "AI Radar" global survey said they were "considering autonomous agents as part of their AI transformation."
Other observers also predicted a rise in the use of agentic AI.
A press release for KPMG's "AI Quarterly Pulse Survey" declared, "2025 is the year of AI agents and scaling AI across the enterprise." The report noted that this year "brings the opportunity to scale and advance AI capabilities across the enterprise, and a majority of organizations are looking to agentic AI, or tools that can work independently to perform tasks and adapt in real time, to help do so."
The KPMG survey found that while 51% of organizations said they are exploring the use of AI agents, only 12% had deployed them.
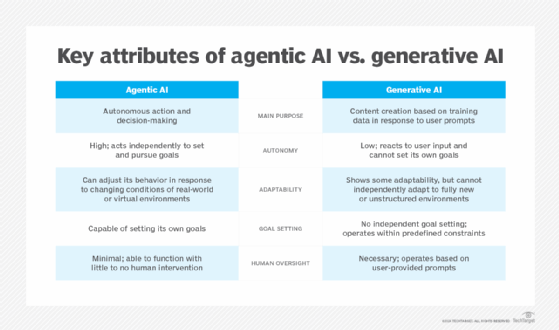
7. Increased use of multimodal models
Most organizations have used GenAI to interpret and create a single data type: text. However, the technology has also been used to interpret and create images, video and audio.
But the use of multimodal GenAI -- AI that can accept and synergize multiple types of data inputs, such as text, images, video and audio -- will increase, according to Sreekanth Menon, senior vice president of global AI practice and innovation at Genpact, a global professional services firm.
"This creates significant opportunities to expand the use of GenAI," he said.
Researchers predict an increased use of multimodal GenAI in the upcoming few years. Gartner, for example, estimated that 40% of GenAI offerings will be multimodal by 2027, up from 1% in 2023.
These multimodal models can combine information from any and all of these modalities to deliver a more complete understanding of the data and to create a more immersive output with which to interact. Experts have noted that multimodal AI is another step toward artificial general intelligence.
8. Continued concerns over responsible AI principles
Although GenAI adoption has soared in the past two years, many people have concerns about its accuracy and applications.
To counter such concerns and ensure GenAI is used ethically and in legally permissible ways, organizations are increasingly focused on responsible AI -- an approach to developing and deploying AI in ways that are fair, accurate, transparent, accountable and explainable.
"Responsible AI is becoming more and more important because it boosts stakeholder confidence," Menon said.
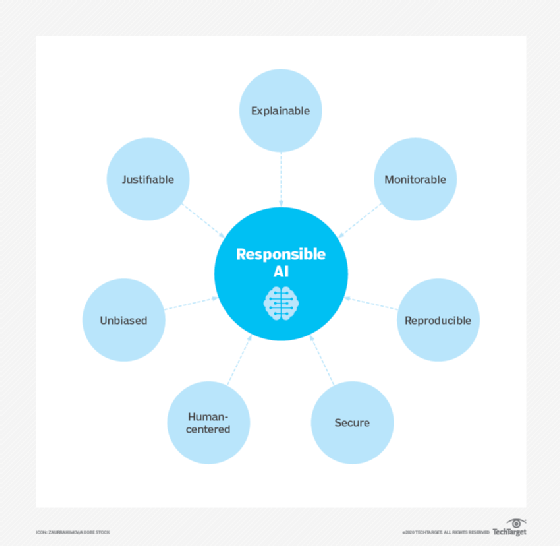
A January 2025 report titled "Implementing Responsible AI in the Generative AI Age," from tech company HCLTech and MIT Technology Review Insights, backs up that assertion, with 87% of respondents stating the importance of responsible AI principles.
However, 85% cited implementation issues, such as complexity, a shortage of staff expertise, problems managing operational risks, regulatory compliance issues and inadequate resource allocation.
9. AI regulatory environment remains fractured
Governments around the world are writing, implementing and -- in some cases -- revising numerous laws and regulations regarding AI in general and GenAI specifically.
"This is going to be on everybody's mind this year: How do we comply with AI regulations?" said Mike Mason, chief AI officer at global technology consultancy Thoughtworks.
Mason said regulations vary from country to country and region to region, making it nearly impossible for any organization to develop a single GenAI approach that works across the globe.
As a result, he predicted organizations will implement multiple approaches for their global AI work, developing one GenAI program to meet EU regulations, for example, and another GenAI program for the U.S. and its regulatory environment.
10. GenAI will impact an organization's cybersecurity posture
When it comes to cybersecurity, GenAI plays multiple roles.
To start, security teams can use the technology to help them detect and respond to incidents more effectively and efficiently.
However, enterprise use of GenAI also creates risk for the organization, as bad actors can attack the models themselves in various ways. This has security chiefs and their executive colleagues working to shore up their defenses, Samtani said.
"We're going to see more attention [being paid] to hardening the surface before we see a big attack -- such as a poisoning attack -- occur," he added.
Additionally, bad actors are using GenAI to craft attacks and find novel ways of attacking by creating "known unknowns" that security teams must try to anticipate, Lukic said.
GenAI tools to know in 2025
When OpenAI launched ChatGPT in 2022, the chatbot was practically synonymous with generative AI. That misconception might have been well deserved at the time, but today there are numerous GenAI tools and related technologies on the market.
Here are some GenAI and GenAI-related tools and categories that AI researchers and technology advisers said are worth knowing in 2025:
- AI chip makers. The most notable and recognized AI chip maker is Nvidia, which designs and manufactures software and hardware, including chips and GPUs.
- Claude. A GenAI chatbot and family of LLMs from Anthropic, an AI safety and research company working to build reliable, interpretable and steerable AI systems.
- Data platform companies. Examples include Databricks, which provides a platform to process, manage and govern the large amounts of data organizations need for GenAI; Hugging Face, a machine learning and data science platform and community that helps users build, deploy and train machine learning models; and Snowflake, maker of a cloud platform to access and analyze data.
- Enterprise software vendors. This category of vendors now incorporates GenAI tools and products into their products for use by their customers.
- Hyperscalers. These types of cloud service providers, such as AWS, Google Cloud and Microsoft Azure, offer the massive amounts of compute power needed to run GenAI applications and LLMs.
- OpenAI. The research and development company that developed ChatGPT.
- Open source GenAI. An alternative to proprietary GenAI models, open source GenAI enables developers to create GenAI programs and collaborate on advancing the models within the rules governing each open source resource. Examples include Mistral AI, OpenAI's GPT-2 and Stability AI's Stable Diffusion.
Editor's note: This article was updated in February 2025.
Mary K. Pratt is an award-winning freelance journalist with a focus on covering enterprise IT and cybersecurity management.