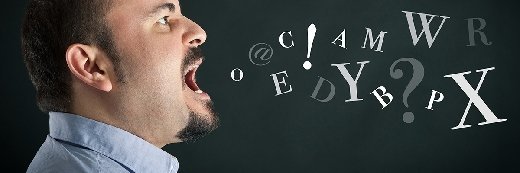
pio3 - Fotolia
NLP progress in BI and analytics slowed by language barriers
Natural language processing shows potential in simplifying data access and deriving deeper insights, but NLP's strengths can be its weaknesses in reaching the Promised Land.
Natural language processing, the latest advancement in business intelligence and analytics applications, provides an easier way to access quality data and derive deeper insights. But because of its sophistication and nuances, NLP progress has been limited as well.
There's a very good reason why BI and analytics have traditionally required some level of expertise. People don't intuitively understand how to query machines.
Querying a SQL database in the traditional way, for example, requires an understanding of the SQL language and how it works. By comparison, NLP can take a natural language query, translate it into a SQL query and communicate the result back to the user in natural language. That scenario involves three different natural language technologies, all of which fall under the general category of NLP:
- natural language understanding -- understanding written or spoken text;
- NLP -- converting text into structured data; and
- natural language generation -- converting structured data into text.
NLP progress in general BI and analytics
Tableau Software has a new capability in beta called Ask Data, which enables users to ask questions about sales, profitability, etc. and receive a natural language answer. Both Tableau and Qlik have Narrative Science extensions that automatically supplement their respective charts and graphs in dashboards with a natural language narrative. Microsoft Power BI supports natural language queries, although reports and dashboards haven't achieved feature parity as yet.
While these examples demonstrate general BI and analytics platforms taking advantage of NLP, a separate issue is using NLP to do particular types of analytics. The Lexalytics Intelligence Platform, for instance, analyzes unstructured text databases, data warehouses, data lakes, search engines, social media and web crawlers. Probably one of the more talked-about use cases for unstructured text analysis is social media sentiment analysis.
Typically, when data scientists want to understand unstructured data, they use one of two techniques: Classification uses supervised machine learning to separate text into predefined or labeled classes such as positive and negative sentiment, while clustering uses unstructured machine learning to separate text into groups without using predefined labels.
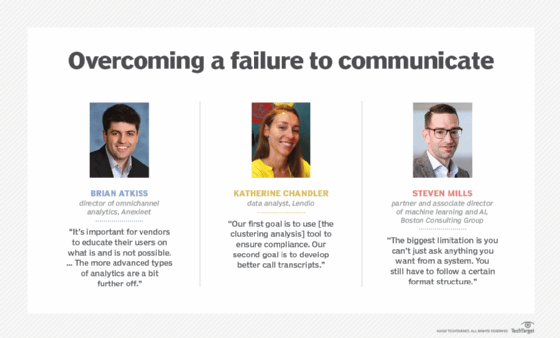
Lendio, a small business loan marketplace, is considering applying clustering analysis to customer service call transcripts to identify common issues, customer sentiment and trends so service representatives won't have to listen to the calls, according to Lendio data analyst Katherine Chandler.
"We currently have some transcripts that we give to people in our call center, but if we have access to all the calls that have ever happened, we're hoping we could develop some better transcripts," Chandler said. "Our first goal is to use this tool to ensure compliance. Our second goal is to develop better call transcripts."
Similarly, a multinational technology service provider wanted to improve customer experiences, but it could track only 5% of customer interactions using surveys and manual quality control. The company worked with customer experience analytics platform provider Summatti to improve the quality of insights.
To prepare for the platform's ongoing monitoring and analysis of text, the company analyzed six months of customer relationship management and interactive voice response data to identify positive and negative experiences. It also incorporated customer service success metrics (key performance indicators). After setup, the platform monitored and analyzed customer interactions via phone, email and chat so the company could identify and proactively address issues, monitor employee performance and improve channel retention rates.
Not quite there yet
End users tend to expect more from NLP than it can deliver because human language is a more natural form of communication than SQL queries or Boolean searches. There's also a common misconception that AI in all its offshoots, including NLP, is a general form of machine intelligence that can be simply applied to narrow problems. Narrow AI is the current state of the art, so a product built to do sentiment analysis is very effective at doing sentiment analysis but not contract review.
"It's important for vendors to educate their users on what is and is not possible," said Brian Atkiss, director of omnichannel analytics at digital experience consultancy and reseller Anexinet Corp., which uses NLP to understand customer interactions. "[If you just want to know] how many customers or how many prospects my team reached out to today, that's probably possible, but a root cause analysis, the more advanced types of analytics are a bit further off."
To help set users expectations when he's conducting demos, Steven Mills, associate director of machine learning and AI at Boston Consulting Group, makes it a point to present the shortcomings of NLP progress in a humorous way. "The biggest limitation," he said, "is you can't just ask anything you want from a system. You still have to follow a certain format structure, and there are limits to that. Where we want to get to is a question-and-answer type interface, but those types of natural language understanding and capabilities are only so good. We want systems that can interpret complex questions, but we just aren't there yet."
Hurdles to jump
One challenge to NLP progress is intent; what people say or type is not necessarily what they mean. A BI or analytics platform using NLP should be able to infer the user's intent and deliver a relevant result. To do that, the system needs to understand the relevant variations of a query. It also has to understand the context of the query.
The context of data usage is also important. Most departments use software applications that are specific to their function. While the data in those departmental systems may be accessible with BI or analytics via an API, data used outside its originally intended context needs to be handled accordingly. Because context is such a big issue with NLP, a successful NLP pilot in one department may not scale well across the enterprise or even to another department because each may require different models, data or data integrations to achieve their goals.
More BI and analytics vendors are adding NLP capabilities to their products to improve the user experience. Eventually, users will be able to carry on an interactive dialogue with these tools to get the information they need quickly without clicking through analytics dashboards or BI reports. But before rolling out an NLP-powered system broadly, it's wise to understand its capabilities and limitations so that appropriate end-user expectations can be set.