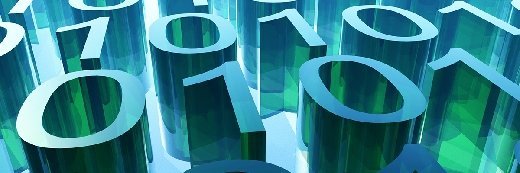
chrisharvey - Fotolia
10 tips for implementing visualization for big data projects
Organizations need to keep users and design at the forefront when launching data visualization efforts, according to experts. Find out why colors and sizing matter.
Effective visualization for big data techniques need to go beyond just painting pretty pictures for management. Experts say enterprises can improve their results by considering layout, designing iteratively, engaging users and understanding business needs.
"The key is to tailor the specific visualization to its data, context and audience -- not to blindly follow any visualization rules," advised Aaron Kalb, vice president of design and strategic initiatives at Alation, a data catalog provider. Kalb and other experts in the field had the following 10 tips for organizations embarking on data visualization projects:
1. Keep the user in mind. Use color, form, size and placement to inform the design and use of your visualization, said Dan Gastineau, visual analytics practice lead at Aspirent, an Atlanta-based management consulting firm.
Aspirent uses color to draw attention to the aspects of the analysis that it wants users to focus on. Size effectively communicates quantity, but too much use of different sizes to communicate information can become confusing. Instead, size is employed selectively in places where consultancy team members want to emphasize a point. Form determines the shapes used to present an analysis: for example, whether to use line or bar charts to present certain types of information. Placement of objects is as critical to effective communication as the objects themselves, Gastineau said.
2. Tell a coherent story. Speak to your audience and keep design simple and focused. Minute details like colors to the number of charts can help ensure that a dashboard tells a coherent story. "A dashboard, much like a book, needs design elements that keep the reader in mind and does not simply force fit all the data one has access to," said Saurabh Abhyankar, senior vice president of product management at MicroStrategy. The design of dashboards will be a factor that drives adoption.
3. Prepare to design iteratively. Work in ways to elicit frequent feedback from visual analytics users. Data exploration sparks new ideas and questions over time, and making it more pertinent over time and over adoption makes users smarter.
Solicit and incorporate feedback from your recipients to improve the experience. Building a quick proof of concept, getting feedback quickly and iterating tends to lead to a better result, faster, said Nick Mihailovski, lead product manager for Data Studio at Google Cloud. Even incorporating surveys and forms into polished reports can help ensure that the result of visualization for big data efforts indeed aids the intended recipient.
4. Personalize everything. Make sure that the dashboard reveals personalized information to the end user, and make it relevant. Ensuring that the visualizations are responsive in design to the devices they're on and offering offline access to end users will take it a long way. Engage your audience, and propagate a data culture by using well-designed and interactive visualizations to make analytics engaging and fun, Mihailovski said. It should also be intuitive for employees to access, visualize and share their reports with live, dynamic data.
5. Start with the analysis objective. Ensure that the data type and analysis objective informs what visualization type is chosen. "People often take a backwards approach by seeing a neat or obscure visualization type and then trying to fit their data to it," Mihailovski said. A simple table or bar chart may sometimes be most effective for visualization for big data projects.
6. Keep governance in mind. This might take work, but it's important that end users trust the data. Gather all the help you need from a technology, process and people standpoint to ensure that the data is vetted and accurate, Abhyankar said.
7. Empathize with the viewer. Every situation invites a different approach to visualization. For example, many data visualization gurus categorically forbid the use of pie charts , since the human eye and mind can much more readily measure differences between lengths or positions than between angles. But turning a pie chart into a bar chart can make it less obvious that the different parts add up to a single whole. It is also harder to recognize certain fractions. Consider variations like a donut chart, which is just a pie chart missing the middle for quickly showing patterns like a 75%, 20% and 5% breakdown.
8. Understand the business. Spend some time with business users to understand what they want to achieve from the visualization for big data product, and what data needs to be used to provide them with the required insights. If necessary, invest in some tools or techniques to profile and transform the data. "We are dealing with tons of data in big data -- hence, empowering the user to gain from this scale is important," said Naresh Agarwal, head of data and analytics at Brillio, a technology consultancy.
Knowing the trends in the business is important to help users apply the latest metrics and analytics to drive better business decisions. Different dashboards need to be conceived, keeping the end user in mind. Management, analysts, IT and business users will derive value from different types of visual analytics explorations.
Visualization for big data is useful only if it addresses the interests of the stakeholder for whom the analysis is performed. Clearly understanding stakeholder motivations will enable the development of visualizations that are at the right level of granularity and detail to allow stakeholders to act upon them, said Nitin Bajaj, director of business intelligence and analytics at NTT Data Services.
9. Connect visualizations. Ensure that different visualizations on the dashboard are connected and can be linked instantly to complete the story. For example, if you are analyzing a locationwise sales summary report, you should also be able to do a year-on-year analysis or compare sales across different products, said Pratik Jain, technical architect at Kyvos Insights, a business intelligence software provider.
Make sure visualizations for big data can be updated and interrogated in real time. A static display, or one that doesn't reveal where its underlying data comes from, is not helpful in analyzing the fast-moving, varied streams of big data that enterprises must process, said Zachary Jarvinen, senior analytics product marketing manager at OpenText, a supply chain software provider.
10. Simplify where possible. Most leading visualization for big data tools are feature-rich, which often results in a tendency by analysts to build visualizations that are dense and overly complex. This can make it difficult to glean actionable insights. A good analyst will simplify visualizations to address only the hypothesis at hand, Bajaj said. Then the analyst should check in with the stakeholder early and often to ensure that the final product is not loaded with bells and whistles that look cool on first blush, but do not address the needs of the stakeholder directly.