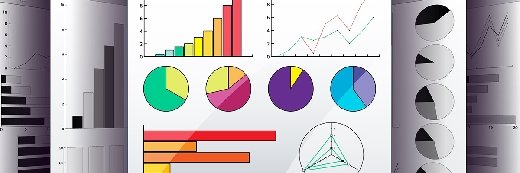
Fotolia
Seven good data visualization practices for visual integrity
Data visualizations need visual integrity to ensure that the data they present can be interpreted correctly. Follow these design steps to help make visualizations trustworthy.
It's difficult to read raw data in spreadsheets, reports and the like. As a result, good data visualization plays an increasingly important role in conveying the information embedded in corporate data sets to business decision-makers.
In many organizations, there's more to the data visualization process than designing charts and other infographics. Both data analysts and business workers use self-service BI and data visualization tools to create presentations that blend visualizations with text to tell a data story. That's meant to be more interesting -- and enlightening -- for execs than viewing rows of raw data or a series of charts.
But as citizen data science goes mainstream and the number of people using analytics and visualization tools grows, it's imperative that they follow proper design procedures to maintain visual integrity in the infographics and data stories they're creating.
In this context, visual integrity -- or graphical integrity, as it's also called -- means ensuring that what's presented accurately represents what's in the data being visualized, and that no design choices distort or obfuscate the inherent facts and analytical findings. Take a look at these recommended data visualization practices to help foster visual integrity as part of BI and analytics initiatives.
Include info on data provenance. Not properly citing the sources of the data used in constructing a data visualization leaves out potentially important information about how the data was collected, which can affect the credibility of the message being conveyed.
Clearly define the data variables. A visualization designer may presume that the intended audience will understand the meanings of the data variables incorporated into it. In many cases, though, the visual presentation only makes sense if accompanying text explains what it involves. All data variables should be unambiguously defined to prevent possible misinterpretation.
Make sure the data being used is complete. Sometimes, a data analyst decides to exclude information from a visualization or to organize it in a way that influences the viewer's interpretation in possibly misleading ways. One example is the omission of outlier values that would force a rescaling of the visualized data. Another involves manipulating the axis thresholds in a chart by setting the ranges of the axes to affect how viewers compare different data values. A third example is leaving out dependent data variables that are correlated to the ones included in the chart. Not even properly labeling the axes is a violation of data completeness that can undermine an otherwise good data visualization.
And make sure that it's consistent. Present the data in a way that it can be correctly interpreted by viewers. A common example of how to go wrong is overlaying multiple axes on a single chart and including lines pegged to the different axes -- for instance, having two different y-axes, one labeled on the left of the chart and one on the right. Doing so implies comparison; however, if there's no relation between the two axes, there should be no inferred relation between their lines. Other examples include feeding false assumptions about the use of colors, shapes, textures and line types and thicknesses.
Be consistent on scale, too. The size of the elements in a good data visualization should correspond to what's indicated by the data. In some cases, an enthusiastic designer might try to highlight a finding by scaling the results to make it look more prominent. Doing so is deceptive, especially as the ratio between the size of the graphical element and the actual data value gets bigger. (This is what Edward Tufte called the "lie factor" in his book The Visual Display of Quantitative Information.)
Keep visualizations free from visual "noise." A well-designed data visualization shouldn't include icons or other graphics that don't correctly reflect the data being presented. In other cases, designers add editorial commentary to the images that can influence how data is interpreted.
Don't filter out data so it can't be viewed. Sometimes the design of a visualization limits the ways data can be viewed -- for example, by filtering constraints for the data dimensions that business execs can drill down into in an interactive dashboard. Visualizations shouldn't prevent viewers from freely looking at all the included data values independent of a predefined set of filtering criteria.
You might be surprised to see how many visualizations violate some of these fundamental guidelines. Data analysts and other users must be careful to design their data visualizations with true visual integrity to ensure that the information being presented is viewed as credible -- both literally and figuratively.