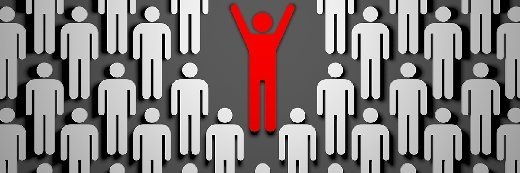
AKS - Fotolia
A tale of two retailers profiting from big data
A young and an old retailer shed light on how they're profiting from big data. (Hint: The secret is in their approach to project management.)
Etsy Inc., an online marketplace for vintage and handmade products, could be described as a grand experiment, at least when it comes to big data and analytics. "We're a continuous delivery shop," Nellwyn Thomas, director of analytics at Etsy, said. "Instead of pushing code once every week, two weeks or three months, we push code multiple times a day."
Some of that code is for A/B testing, which exposes one group of customers to site tweaks and new features while leaving others alone. Data is collected over a period of time, and the results provide a window into customer behavior and inform the team how to improve the site, Thomas said. In fact, A/B testing has become so essential to running the business, Etsy built its own A/B analyzer tool to aggregate and visualize the data automatically.
That kind of commitment to experimentation -- a style more associated with research and development (R&D) than with conventional IT project management -- is crucial for businesses looking to profit from big data, according to experts. Robert Morison, lead faculty member with the International Institute for Analytics in Portland, Ore., said typical IT projects tend to be goal- and milestone-driven. "With analytics in general, predictive analytics more so and big data in particular, the process is iterative. You experiment and evaluate what you're learning and decide what to do differently next or whether to proceed at all," he said.
Experts like Morison aren't just talking science and medical research here, or of Etsy in particular. An R&D mentality is a major criterion for big data success across all industries, they say -- from manufacturing to telecommunications to retail. In the past, data experiments were prohibitively expensive, according to Mike Gualtieri, analyst at Forrester Research Inc. But today, with technologies like cloud and big data platforms like Hadoop, cost is less of an issue.
"Part of the phenomenon about big data is that it's much less expensive to run experiments to analyze big data," Gualtieri said. "And, because of that, you can run more experiments and you can use more data. That's a key use case."
A/B testing at Etsy
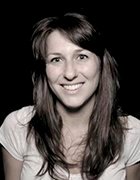
That R&D mentality is alive and well at Etsy. When Thomas and her team noticed that a "popular landing page" snagged customer attention but failed to keep it, they decided to investigate the problem. On closer examination, they noticed the page had minimal "calls to action." "You can leave, buy, search or click on two additional images in the [landing page's] 'shop,'" said Thomas, a featured speaker at the recent Strata + Hadoop World in New York City. "We wondered, 'What if we just showed more items?'"
Utilizing A/B testing, some site visitors were shown a strip across the top of the page that algorithmically displayed additional images within a seller's shop. The results of the experiment were "astounding," Thomas said, including "a 10% reduction in bounces, an increase in pages viewed and an increase in conversion rates." The value of the experiment, however, was not in the metrics for that particular page. "The most important part was that we validated the idea and determined this was a direction to go in -- that this was a meaningful way to change the buyer experience," she said.
But success within an R&D framework isn't always so cut and dried. Businesses also have to be open to ambiguity, to failure and even to the unknown. "Not all projects are [perfectly] designed from the get-go, and [researchers] don't always know where they're going," said Doug Laney, an analyst with Gartner Inc.
Forrester's Gualtieri suggests that businesses approach big data projects the way a venture capitalist invests in companies: "A venture capitalist will invest in 10 companies hoping one or two will hit, and that's how companies have to view advanced analytics and predictive modeling." A case in point is Etsy's recent experiment to bolster the reach of its email marketing channel. When Thomas and her team tried out a call-to-action feature at the top of the receipt box, asking customers to confirm their email addresses, the direct results of the test were promising: The company saw a 40% increase in users confirming their accounts.
The indirect results, on the other hand, were "totally unexpected," Thomas said, notably a 3% decrease in the average number of conversions per user. Because the call to action appeared after the purchase, "there was no reason to think this would influence your future purchasing behavior," she said.
Initially, Thomas and her team thought something was wrong with the data (like any other company, Etsy does battle with data quality), but a closer inspection didn't reveal anything significant. They tried cutting and segmenting the data to see if they could sift out some explanation to no avail.
In this "state of ambiguity," Thomas discussed keeping or discarding the new feature with stakeholders, essentially trying to nail down whether it served Etsy's buyers and sellers. "The point of the story isn't, 'Oh, you found an issue and you solved it with data.' In some cases, you can't solve the problem, and you still have to make a decision about what you're going to do," she said.
Three reasons for big data failures
Robert Morison, lead faculty member for the International Institute for Analytics, provided three reasons businesses experience big data failures. Briefly, they are as follows:
- As cited in the piece, clinging to a traditional IT project management style. Solution: Think R&D.
- Businesses are taken in by the hype and make their first big data project a big deal. Solution: Businesses should start with a smaller project that will "move the proverbial needle."
- Reasonably good analytics are done, but they are not adopted. Solution: The business has to own the problem or the ambition to improve.
LL Bean's big data experiment isn't just for Internet-only businesses
For other industries, figuring out how to use data to find a path forward is a matter of survival. L.L. Bean Inc., a 102-year-old retailer in Freeport, Maine, is a catalogue business that's transitioning with the times. And that transition is not just technological, but cultural -- an experiment in itself. Back in the mid-90s, L.L. Bean flourished using "highly structured, big production systems, all built up around what, today, we would consider one marketing channel," said Chris Wilson, senior vice president of direct channel for the retailer, at Strata. "Now, we have roughly 30 different marketing channels."
Like other retailers, L.L. Bean wants to reach customers no matter where they are and provide as personalized a service as possible, but that's easier said than done. "The real wild card here is that, short of full authentication on every visit, we're never 100% confident in how we're stitching customers together across all of those visits," he said. "So all of these data elements that we used to think of as deterministic are really probabilistic, and it adds a scalar component to all of these variables that we've been thinking of as binary."
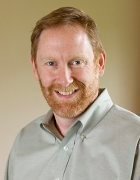
The complexity of the big data problem aside, L.L. Bean also wants to "go fast." Neither problem was going to be solved with the company's legacy enterprise data warehouse approach alone. "At a fundamental level, there is just a lot more unstructured data that doesn't play terribly well with our traditional, relational database approach," Wilson said.
So the retailer reached out to RichRelevance, a personalization service provider based on Cloudera Hadoop that lists mega-retailers like Wal-Mart and Target as its customers. Within the pilot program's first six months at L.L. Bean, "we'd already put two times more marketing data in the cloud than we had on our internal data warehouse that took us 20 years to aggregate," Wilson said.
Data collection is only a fragment of what L.L. Bean is after. What the retailer really wants to do is make data and analytics a core competency across the company: "[We want] to move away from that center of excellence approach we've followed for years and really distribute these tools across the organization," he said.
Wilson's aim in moving to a more decentralized approach is to produce a better experience for the customer by tailoring its content and providing faster, more personalized service, but that will require the company to embrace an experimental, iterative approach to doing business. "The goal isn't to create a new, static structure going forward, but to have an organization and platforms and systems that can support an adaptive, observant, responsive organization going forward," he said.
Let us know what you think of the story; email Nicole Laskowski, senior news writer, or find her on Twitter @TT_Nicole.