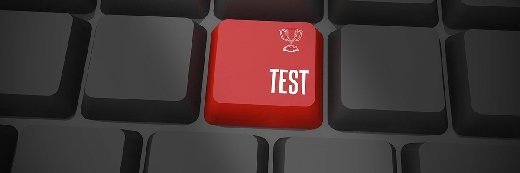
WavebreakmediaMicro - Fotolia
Among AI use cases that matter to CIOs, ITSM ranks high
AI use cases for your ITSM program abound, but early adopters caution that vendor offerings are immature and will require hands-on testing for best results.
Advanced AI for consumer application gets the lion's share of publicity. But CIOs in most industries are likely to get higher returns on AI programs that solve boring problems in IT service management and, as a result, increase IT visibility, improve staff utilization, save money and deliver new business value.
Jeromy Grimmett, CTO at CloudBrix, a managed service provider, said the first task for IT leaders is to sift through the hype for meaningful AI use cases for ITSM.
"The promise and hopes for what AI can do are real, but the reality is drastically different. It is important for CIOs to focus on solutions that will deliver value to our internal and external customers," Grimmett said. To that end, CloudBrix has started experimenting with new AI technology from Loom Systems to get better insight from its ITSM performance monitoring and incident analysis tools.
Indeed, experimentation is the operative word for CIOs when integrating AI into ITSM processes, according to Grimmett and others. Potential AI use cases in ITSM run the gamut -- from improving performance monitoring to using chatbots to field and decode employee requests. In cybersecurity, the use of anomaly-detection algorithms could help find and resolve threats much better than rules-based metrics.
But while AI use cases for ITSM abound, early adopters like Grimmett cautioned that the offerings are relatively immature and will likely involve a good deal of hands-on work as IT teams figure out what works for their ITSM programs. Another bit of advice? Integrating AI in ITSM is more like onboarding an employee than deploying traditional technology.
"You have to provide a scope of work and assign responsibility, just like you would for a person," Grimmett said.
Augment humans, don't replace them
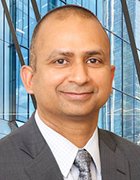
Vinodh Swaminathan, principal, innovation and enterprise solutions at KPMG, sees three key AI use cases for ITSM: systems management, user experience and threat management.
With systems management, he said the goal is to use machine learning capabilities to better predict and drive systems utilization and provisioning. The user experience can be improved using tools like chatbots that help answer questions and automatically solve problems. He's also seen promise around using AI as an add-on to existing security tooling for better detection of cyberthreats.
In these early days, it's probably more useful for CIOs to think of the "A" in AI as augmented or automated intelligence, said Mike Gualtieri at Forrester. The focus should be more on improving existing decisions made by humans with better recommendations. He said, "In the field of ITSM, AI is about predicting failures before they happen. And if it does happen, it is about predicting the best action to take to resolve it."
Vendors using AI to predict failures include BMC Software Inc., Datadog, Dynatrace, ServiceNow and Loom Systems. Gualtieri said the maturity of these vendors in implementing AI is still low as they shift from rules-based approaches to more robust anomaly-detection algorithms. But he expects offerings to mature quickly as vendors and their customers build better models, gain practice in implementation and learn from larger sets of data.
Replacing rules with machine intelligence
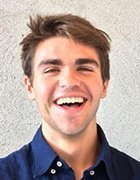
CIOs should consider replacing rules-based engines with AI anomaly detection gradually, as exemplified by the strategy of Calvin French-Owen, CTO of Segment, an API integration service. He said he sees benefits in shifting from a rules-based to an AI approach. Segment has begun gradually implementing new AI anomaly-detection algorithms from Datadog to complement its rules-based alerting systems.
"The idea here is that we don't have to spend a ton of time endlessly tweaking the thresholds on specifics rules, and, instead, let Datadog do the hard work," explained French-Owen. "It's simplified the contract on our end by letting our developers specify: 'Alert when disk will run out in the next 30 minutes,' rather than saying when disk is at 70% full versus 80% versus 90%."
Segment has also been focusing on using AI for correlating metrics. The API integration service tracks millions of metrics per second from tens of thousands of containers. It can be tricky for humans to find patterns in these. For example, French-Owen's team was recently hunting delays in the company's data pipeline. AI helped them determine the root cause was due to a component hitting its I/O limits that wasn't even triggering alerts. He said, "Had we been able to quickly correlate those instances maxing out their network connections, we could've saved hours spent debugging and jumping from microservice to microservice."
Build a better chatbot interface agent
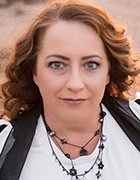
Aspect Software Inc., a call center management service, has been experimenting with chatbots to complement and, in some cases, replace the work of servicing internal IT support queries, said Stefanie Causey, senior director of information services solutions delivery at Aspect. As part of this project, Causey's team is building up a comprehensive knowledge base on top of BMC Remedy. This helps answer simple support questions so that her staff can focus on more challenging issues.
One of the biggest challenges lay in integrating the chatbot to various ITSM-related data sources spread throughout the enterprise. Causey's team adopted the Jitterbit integration as a service platform to make it easier to connect the chatbot to these data sources. This has complemented a longer-term strategy of replacing a legacy Oracle E-Business Suite system with best-of-breed cloud services for back-end infrastructure, Causey said. "We would not have been able to do the AI stuff as easily with Oracle. But now it is much easier with everything in the cloud."
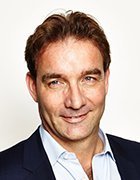
Thomas Reuner, senior VP at HfS Research Ltd., said that chatbot and conversational interfaces are still in the early days for ITSM. Going forward, vendors like IPsoft and Arago are planting the seeds for automatically remediating complex IT service requests with sophisticated agent technology. Reuner said, "Most organizations look at those technologies as augmenting, rather than replacing, human agents."
Onboarding AI rather than deploying it
For CIOs weighing AI use cases for ITSM, CloudBrix's Grimmett and others advised they think about the introduction of AI as more akin to onboarding an employee than deploying a new service. "It is not really a software app -- it is an intelligence -- and it has to be treated that way," Grimmett said. "You have to provide a scope of work and assign responsibility, just like you would for a person."
But when it is up and running, AI can find insights buried in thousands of data points that are not readily visible for humans. For example, CloudBrix was running into problems diagnosing Simple Network Management Protocol (SNMP) traffic bottlenecks. Their AI implementation found a mismatch in time stamps that human engineers had not been able to identify.
One caution: An AI system can flood the IT department with too many insights of little importance if not well implemented. It's important to know where to direct the new AI's attention to get the most rewards. Jeff Kaplan, managing director of ThinkStrategies, an IT consultancy, explained, "Prioritizing which data points are most critical is essential to properly configure the solution to address the organization's greatest needs."
Earning trust
Over time, these better insights begin to earn the trust of CIOs and their teams. Traditional rules-based thresholds are simple to parse. Segment's French-Owen said, "AI alerts are much more of a black box, and I think developers will tend to mistrust them until they are proven to work."
A good strategy is to try out these alerts and watch where they work and fail in order to de-risk the AI alerts. Over time, this should embolden CIOs to use these alerts to drive automatic remediation measures.
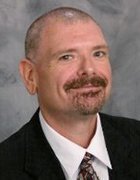
AI may gain trust faster in industries that are feeling challenged by the traditional threshold metrics that drive alerts. For example, Mark Kaplan, director of IT for Barbri Inc., a legal prep course service, has been experimenting with Dynatrace's AI capabilities for identifying problems in its seasonal traffic spikes. These normal shifts in traffic make it difficult to set up alerts because it's hard to predict what the thresholds should be. Kaplan said, "What we like most about Dynatrace is that its software actually learns our environment; not just the physical aspects of the environment, but how the environment itself operates."
Over time, as the system learned Barbri's environment, it yielded better insights into traffic changes and app performance compared to previous years. The first year required a lot of manual intervention to create the baseline, but the system required less hands-on work as it learned..
"Once we had the baseline for that traffic set, Dynatrace figured things out intuitively going forward," Kaplan said, adding that it's also important to inform the AI system when there is a change to the business that might affect the number of users or their behavior.
Architect for data flows
Indeed, AI systems need to analyze a vast amount of data to deliver meaningful results. It can take time for CIOs to prioritize the steps required to configure these data flows in a way that maintains governance, risk management and compliance requirements. For example, CloudBrix spent about six months configuring their network to send ITSM data to its Loom AI engine.
Part of this process involved adding a new encryption overlay for securely transmitting SNMP messages to the AI engine. Then they had to add some custom code to funnel information from multiple protocols into the engine in a common format. Grimmett said, "Out of the box it works with standardized syslog messages. We had to implement some specialized secret sauce to funnel that information across multiple protocols to get it into the AI in a readable form, so we could interpret it."
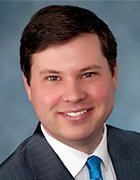
Over time, the AI use cases in ITSM could expand beyond back-end data centers to include customer experience infrastructure as well. For example, Tom Ivory, head of strategic innovation for Capgemini, said the firm has begun implementing AI-infused ITSM-like capabilities for a couple of entertainment parks and cruises. In this case, the AI component gives them visibility into the performance of applications running across physical gates, customer service kiosks and specialized customer authentication terminals.
As CIO's gain confidence in AI recommendations, these tools are also likely to play a greater role in automatically remediating problems.
"Eventually AI should not only surface anomalies, but automatically heal your infrastructure," Segment's French-Owen said. Companies pour millions of dollars per year into building out their own autoscaling, self-healing infrastructure. "It's my hope that alerting, metrics and infrastructure management all become table stakes for various cloud providers and [software-as-a-service] tools."
It is important for CIOs and CTOs to focus on the business problems they are trying to solve rather than on implementing AI technology for its own sake. And many business problems are better solved with other approaches, reminded CloudBrix's Grimmett. "The best answer may be to hire a database administrator to clean up your data rather than implement an AI tool."