The push is on to build the autonomous enterprise
The concept of an autonomous enterprise is becoming more real as enterprises combine technologies to automate all kinds of human work. Welcome to the 'Autonomous Revolution.'
Enterprises are in the early phases of a revolution whose mission is to make more kinds of business systems, equipment and processes perform with less human intervention. This autonomous revolution is rapidly moving from one-off experiments to a collective effort to build digital fabrics that can keep up with the rapid pace of change in supply chains, geopolitics and the environment. The promise? An increase in efficiency, scalability and profitability on a level previously unknown.
At the same time, the advent of more powerful AI systems, called autonomous AI or agentic AI, is raising alarm bells from AI researchers and government regulators. AI pioneers, including Geoffrey Hinton and Yoshua Bengio, have argued for increased oversight of autonomous systems built using generative AI large language models baked into ChatGPT and Bard.
These new systems can improve the deployment and use of autonomous systems within the enterprise. But they could also quickly spin out of control or empower devastating cybercrime attacks and disinformation campaigns.
Warnings about autonomous AI, notwithstanding, the push to build various types of autonomous systems is already shaping enterprise functions. Autonomous IT systems are patching security vulnerabilities, autonomous chatbots are managing customer experiences and a new generation of robots is automating the warehouse. The use of AI to improve IT infrastructure has become a quest to develop an autonomous infrastructure that is infused with sophisticated decision engines and spans the enterprise.
"I've always felt that the vision should be to open up an automation platform and say, 'Automate my enterprise!'" said Craig Stewart, an independent SaaS consultant specializing in IaaS tools.
In Stewart's vision, the platform would be able to understand the applications and data in use, the automation required and the next best action to take. Granted, there's a way to go for this to happen, he said, but reality is getting closer.
"As the journey progresses, the user is getting more accurate predictions and getting them more rapidly on the automations required," he said.
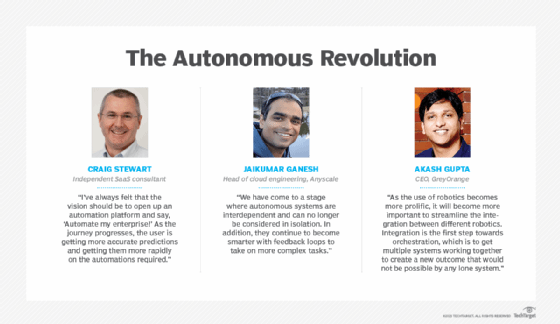
Cloud, data, IoT and AI connecting autonomous systems
Progress is being driven by a convergence of cloud, data and IoT infrastructures. As these technologies become more connected, they are stitching pockets of autonomous systems into a more capable and coherent whole. The result is that physical spaces, business processes and IT are being transformed across industries and across enterprise departments:
- Physical spaces. Autonomous systems can be found in physical spaces, such as warehouses where robots unpack boxes, stage goods for human packers and help clean the premises. These systems are also now well-embedded in the trucks, ships, cars and other vehicles that move goods. Ports are starting to deploy autonomous cranes that unpack goods more efficiently. Autonomous systems are also improving the safety and efficiency of mining operations.
- Business processes. Autonomous capabilities are moving into business processes via rapidly evolving technologies such as robotic process automation (RPA), which mimics the way humans click and scroll through business apps. More intelligent variants, called intelligent process automation, apply AI to enhance the automation of routine business process tasks and expand the types of process tasks that can be automated. The intelligence provided by AI now allows these software bots to respond to unexpected changes in the apps, environments and workflows -- a capability that distinguishes autonomous systems from traditional automation. Furthermore, these intelligent automations are gradually being scaled and orchestrated autonomously by a new generation of process intelligence and management tools.
- IT operations. On the IT side of the house, AIOps -- the use of big data, machine learning (ML) and other AI techniques to gain visibility into IT systems -- is slowly shifting to autonomous operations. Autonomous AIOps continues to get better at fixing problems. Indeed, it is widely viewed as the only way organizations will be able to support the exponential growth in data and equipment. Meanwhile, Oracle has introduced the concept of the autonomous database to characterize new capabilities for automatically provisioning, optimizing and fixing databases. Security teams are adopting autonomous tools that similarly automatically detect and respond to new breaches. Autonomous software testing is being used to improve test creation, impact analysis, test data management and UI analytics, all while supporting a faster pace of deployment.
"We have come to a stage where autonomous systems are interdependent and can no longer be considered in isolation," said Jaikumar Ganesh, head of cloud engineering at Anyscale, creators of Ray, an open source framework in distributed machine learning. "In addition, they continue to become smarter with feedback loops to take on more complex tasks."
Ganesh previously worked at Uber, where he led a program that uses historical and real-time location data along with a machine learning model to continuously improve ETA prediction. The model is regularly updated as more data is gathered. Uber's automated system for ETA prediction in turn has an impact on the service's supply and demand marketplace, which is another automated system. To understand the consequences of these overlapping systems working in tandem, Uber needs to think about them as a whole and study their interactions.
"Thinking of them as a network, understanding the interdependencies and bottlenecks, is crucial to making them work well," Ganesh said.
Similarly, in a physical warehouse, robots on one part of the assembly line need to be in sync and in coordination with robots on another part of the assembly line. The entire system needs to be thought of as a collection of mini automated systems, Ganesh explained, adding that physical infrastructure will need to be rethought to handle these overlapping autonomous systems. Indeed, new roadway designs for autonomous cars and warehouses designed explicitly for robots are already in the works.
Autonomous vs. automated, augmented and AI systems: How are they related?
Think of autonomous systems, automation and augmentation as standing for different aspects of automation.
Autonomous systems differ from automation because they can make decisions in response to new information or changes in the environment. AI algorithms are central to building autonomous systems but not sufficient. For example, an improved AI algorithm for detecting a cat in a picture doesn't improve the ability of an autonomous car to avoid hitting a cat in the road.
There are also levels of automation: An automatic transmission unburdens us of one aspect of driving -- having to shift gears -- while at the other end is a car that can drive without a driver.
Augmentation enhances the handoff between AI, ML, analytics and humans. Augmented analytics, for instance, helps explain how a new trade restriction could affect the business. An autonomous system would automatically place orders and plan new shipment routes in response to new trade restrictions.
As autonomous systems evolve, the need to balance safety and cost rises
Autonomous systems certainly are not new. Computer scientists have been exploring the core ideas of autonomous systems in different contexts for decades.
In 2001, IBM and academic researchers published the MAPE (Monitor-Analyze-Plan-Execute) architectural reference model for autonomic computing. It characterized autonomous systems as a "computer system capable of sensing environments, interpreting policy, accessing knowledge (data, information, knowledge), making decisions, and initiating dynamically assembled routines of choreographed activity to both complete a process and update the set of environmental variables that enables the autonomic system to self-manage its own operation and the processes it oversees."
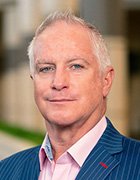
A few years later, autonomous computing entered the public realm when, in 2004, the Defense Advanced Research Projects Agency held its first grand challenge: a driverless car race across the Mojave Desert.
"Autonomy, autonomous and autonomic are big concepts that have been the penultimate goal of systems for at least the last 20 years," said Jon Theuerkauf, former executive at intelligent automation vendors Nintex and Blue Prism.
During the intervening years, researchers, engineers and IT executives have explored ways to strike the right balance between greater autonomy and safety. "It's [satisfying] the demands of complexity and risk prevention at an economical price that are the most challenging for autonomous robotics," Theuerkauf said.
Let's explore how vendors and companies are meeting these challenges as the use of autonomous systems in physical spaces, business processes and IT continues to evolve. First up: robotics.
Robots on the move
Fixed robots have been around for decades in industries such as auto manufacturing. But these simple brutes were locked behind enclosures to protect workers and other equipment from accidents.
A new generation of more intelligent, more autonomous and more collaborative robots are emerging from their cages into warehouses worldwide. Interact Analysis predicted that the mobile robot population could grow from 9,000 in 2020 to 53,000 by 2025. In addition, the firm expects a total of 2.1 million robots of all types by 2025, with about 860,000 introduced in 2025 alone.
Early implementations of mobile robots have focused on improving existing warehouse automation efforts with function-specific robots for moving boxes, unloading trucks, fetching products from shelves and packing boxes. Some firms like AutoStore and Ocado Engineering have begun to apply engineered automation, which turns whole facilities into coherent autonomous ensembles.
Outside the warehouse, robots are starting to take jobs on farms, construction sites, mines and surveillance firms. Service robots autonomously cut lawns and clean hallways.
An AI generalist in the making?
Early AI models were carefully trained and optimized for certain specific tasks. And some did amazingly well at playing chess, Go and Jeopardy. But in general, they failed miserably at doing anything outside of a carefully controlled context.
A new generation of AI models promises to autonomously navigate context across multiple domains. New multimodal AI models such as OpenAI's Dall-E could generate images from text descriptions, and CLIP could associate visual concepts with language.
Future multimodal systems might transfer context across multiple autonomous systems involved in ERP systems, warehouse robotics, autonomous commerce and IT system provisioning. Each of these systems could take advantage of autonomous guidance, orchestration and scalability to respond to a business requirement or customer order in a complementary way.
Mobile robotics sparks demand for orchestration platforms
Leading robotics vendors, meanwhile, are building autonomous orchestration frameworks for coordinating the actions of individual robots with each other and the humans they work with. The orchestration enables a large swarm of robots to go from moving like individual birds to a coordinated flock flying in unison. The multiple systems work together to create a new outcome that would not be possible by any lone system.
"As the use of robotics becomes more prolific, it will become more important to streamline the integration between different robotics," said Akash Gupta, co-founder and CEO at GreyOrange, a warehouse robotics vendor.
"Integration is the first step towards orchestration, which is to get multiple systems working together to create a new outcome that would not be possible by any lone system," he explained, predicting a rise in demand for platforms that reduce the time required to integrate robotics systems individually.
The push for easier integration and orchestration of autonomous systems is also happening in the skies. Increasingly capable drones are monitoring construction progress, keeping tabs on farms and delivering packages. Early operations require humans to track these flying robots at every step of their flight. But new FAA rules for Unmanned Aircraft Systems Beyond Visual Line of Sight could help create frameworks for autonomous orchestration at scale. In addition to paving the way for broader adoption of drones, these early frameworks could down the road help enterprises figure out how coordinate their other autonomous enterprise systems.
Hyperautomation provides pathway to business process scalability
Issues related to orchestration and scaling also loom large in efforts to build autonomous business processes. Business process management (BPM) is an age-old discipline that takes a structured approach to improving the processes organizations use to get work done, serve their customers and generate business value.
In recent years, RPA has captured the attention of BPM experts because of its ability to mimic the way humans interact with software apps automatically. The early RPA software robots, called bots, were initially only capable of automating the simplest tasks. Even small changes in the process caused these relatively brittle bots to break. RPA vendors such as UiPath, Automation Anywhere and Blue Prism have worked on improving bot resilience. They've also applied AI techniques such as optical character recognition, natural language processing and machine vision to increase the ability of RPA bots to make decisions.
But scaling these bots to take on the automation of complex business processes proved a hard nut to crack. Indeed, IT consultancy Forrester Research found in 2019 that most firms had fewer than 10 bots in production. While each bot was becoming more capable and autonomous, it was clear that a better approach was required to scale not only bots but also all types of enterprise automation in general.
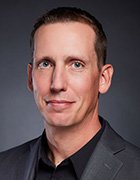
Research firm Gartner coined the term hyperautomation to characterize an approach that combines new automation capabilities with tools for scaling and orchestrating automation across the enterprise. In practice, hyperautomation is not limited to scaling RPA but can be applied to low-code/no-code platforms and enterprise applications for ERP, CRM and supply chain management.
"Companies often execute multiple hyperautomation initiatives as a part of their overall transformation, modernization and innovation strategy," said Ed Macosky, chief product and technology officer at software company Boomi. "This usually involves AI/ML technologies in various form factors for process discovery, process mining, intelligent document processing, decision management and more."
It's important to appreciate the following three scopes of autonomous functionality that support hyperautomation or, on the dark side, adversarial attacks.
- Guidance. Autonomous guidance in hyperautomation is the ability of an automated system to handle granular feedback and controls. This ability is the difference between an automation script that breaks when a service is unexpectedly updated and one that can adapt and respond accordingly. This scope characterizes autonomous vehicles, robots and self-patching IT systems.
- Scalability. Autonomous scalability is the ability to independently identify business processes that are candidates for automation using process mining and process capture tools. Vendors have started to combine these tools with analytics to enable process intelligence. These tools can automatically prioritize opportunities for automation and process reengineering. RPA and low-code tools, in turn, are beginning to use the process maps generated by these tools as starting points for new automation. As these capabilities mature, autonomous scalability will work with continuous integration to automate the process of identifying opportunities, coding the automations, testing them and then deploying them into production at scale.
- Orchestration and collaboration. Autonomous orchestration and collaboration is the ability to independently manage the interactions between individual automations and coordinate an ensemble of automations. It combines decision intelligence with automated configuration, coordination and management of the underlying computer systems. Early examples of this are new enterprise platforms to optimize control across a fleet of different types of warehouse robots or to manage autonomous swarms of drones or robots. Conversely, hackers might train autonomous bots to collaborate on identifying and exploiting vulnerabilities in enterprise systems.
New approaches and improvements to autonomous guidance, scalability and orchestration of business processes will eventually transform other aspects of IT, such as operation, security and testing.
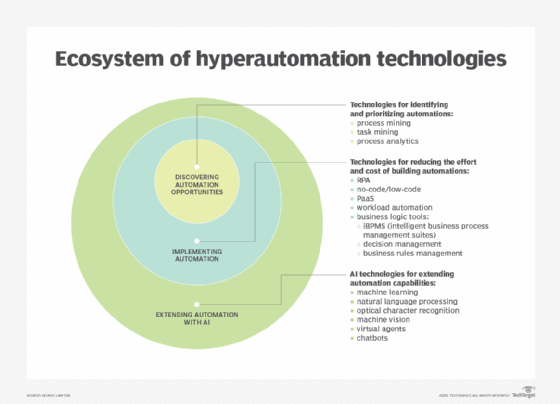
Automated IT systems get smarter with AIOps
IT service management teams have been early leaders in any form of automation. Various IT service management (ITSM) and incident response systems have evolved to help small teams manage ever-larger infrastructure and business requirements quickly and efficiently, most recently with the addition of AI techniques.
Gartner coined the term AIOps in 2016 to characterize the addition of machine learning and big data to assist IT operations. The main idea was that better analytics could help triage false alarms more efficiently, identify problems sooner and guide teams to the root cause of complex issues.
Since then, others have started to explore how better intelligence could not only identify problems but automatically fix them. Oracle launched its Autonomous Data Warehouse and Autonomous Transaction Processing in 2018. In this implementation, the autonomous capabilities help tune the database and optimize indexes on the fly. In addition, the software automatically applies patches in response to the discovery of new security vulnerabilities. Oracle later extended autonomous capabilities to its PaaS, integration and application development workloads.
More recently, ITSM vendors such as BigPanda and application performance monitoring vendors like Dynatrace have begun exploring how AIOps can be applied to autonomous operations more broadly. A recent survey conducted by Dell and Intel on autonomous operations adoption found that 90% of ITSM teams were struggling as a result of the time spent on repetitive manual tasks involving steps that could be automated and function autonomously.
Rise of the autonomous data center
Autonomous systems need to be able to adjust to new feedback automatically yet safely roll back if problems are detected. For instance, Uber uses machine learning algorithms to predict traffic on New Year's Eve, and e-commerce companies like Amazon do the same to predict Black Friday shopping trends. This allows these companies to set up automated systems that scale servers up or down depending on the traffic loads, thus preventing or mitigating expensive outages and downtimes.
"The ability to handle these and automatically detect and roll back changes has become crucial for an enterprise," Anyscale's Ganesh said.
Today, if a change made in the configuration causes a website to load more slowly in a specific geographic region, an automated system detects this and rolls back to the last known good state. Previously, this would involve waking up a human who then needed to understand where the system is failing, figure out the offending change and then roll it back. This often took hours and led to frustrated users and loss in revenue.
Syntax, a cloud consultancy, recently worked on a related use case: The autonomous optimization of disk I/O throughput for mission-critical applications. The new system analyzes customer disk I/O usage and automatically scales throughput up and down throughout the month, allowing customers to have the best possible disk performance while minimizing their total cost.
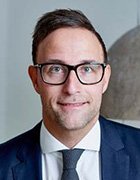
"Imagine you can free up your data center and hardware specialists by not only moving to the cloud but also by adding an autonomous management layer that optimizes your computing resources automatically at the best cost-benefit factor," said Jens Beck, partner of data management and innovation at Syntax.
Autonomous service desk
The move toward an autonomous data center could enable an autonomous service desk that removes high-volume, simple and repetitive tasks from the day-to-day duties -- such as ticket routing, password resets and user extensions. This frees up entry-level service desk employees to take on service desk tasks, which helps teams scale without necessarily adding headcount, Beck said.
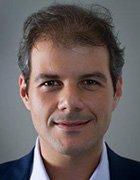
Marcelo Tamassia, global CTO at Syntax, said the use of autonomous capabilities to increase service agility and resource efficiency has the potential to significantly improve IT service delivery.
"Historically, traditional automation has been created mainly to optimize existing processes. However, the proliferation and availability of APIs paired with the rise of AI and ML services have created a unique opportunity to develop autonomous solutions to traditional use cases," Tamassia said.
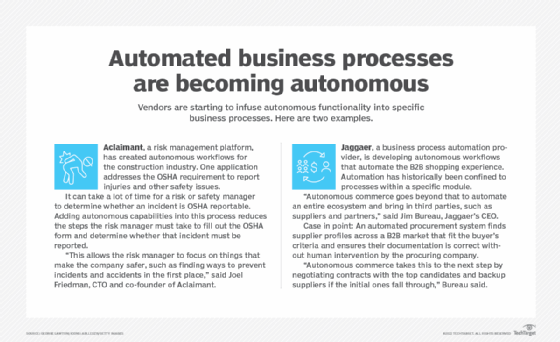
Scaling security to defend against new threats
Security teams also need to consider how autonomous systems can help them address increasingly sophisticated -- and autonomous -- threats. For example, the onslaught of DDoS attacks demonstrates the sheer power of autonomous botnets built on simple devices like video recorders and cameras.
Meanwhile, other hackers are using sophisticated bots designed to mimic humans for bad ends, including scalping tickets, buying up Sony PlayStation consoles and spreading misinformation across social media.
Down the road, these threats could possess the intelligence to outwit even the most sophisticated defenses as the raw power of a mob is fortified by improvements in guidance, scale and orchestration. Autonomous security systems will need to evolve to keep up with this possibility.
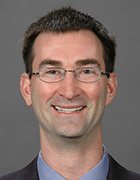
The shift to cloud computing only increases the need for autonomous security systems. As organizations continue to move operations to the cloud, the threat surface gets increasingly complex with the addition of access points for trading partners, employees, supply chains and third-party applications.
It is like building a house with a thousand locks, ripe for the picking, said Mike O'Malley, senior vice president of strategy at SenecaGlobal, an IT outsourcing and advisory firm. Autonomous security could help protect these systems at scale, he said.
There are several ways that autonomous security capabilities can improve workflows in terms of enhanced event detection and response, faster software supply chain updates and more nuanced API security capabilities. These include the following:
- Security information and event management tools, such as Palo Alto Networks' new Cortex XSIAM, are moving beyond static pattern matching capabilities to use AI and ML techniques on more granular telemetry data to identify and respond to novel attack patterns rapidly without human intervention.
- New software supply chain tools can track the discovery of novel vulnerabilities in software libraries and autonomously patch affected systems. Last year, the Biden administration mandated that a software bill of materials (SBOM) be included in all software and services rendered in the U.S. Wider adoption of this SBOM will spur the development of tools that figure out the source of a vulnerability, which in turn could help security teams prioritize upgrades or automatically implement security patches.
- A new generation of API security tools combines deeper insights gleaned from traditional app performance monitoring tools to detect and, in some cases, block suspicious behavior that might not be observed in conventional pattern matching tools.
An autonomous continuum framework for business systems?
Autonomous capabilities typically exist along a continuum. One practical framework that illustrates the continuum are the five levels of self-driving capability developed by SAE International in 2014.
At level zero, the driver is in full control. The first level of self-driving has features like automated braking and lane assistance to improve the response to accidents ahead or unintended lane changes. In the middle, more autonomous capabilities enable adaptive cruise control and the ability to move around slower moving vehicles. At level five, fully autonomous systems can assume complete control without human intervention in all conditions.
A similar kind of framework could help clarify autonomous capabilities across business systems, said Hila Lamm, co-founder and chief product officer at Innovesta Technologies, a conduct intelligence platform. "Automated processes can improve efficiency 100 times over, but human intervention is sometimes necessary for decision-making and creativity."
Businesses need to approach autonomous systems cautiously to ensure that RPA bots do not create new problems, expose critical data or run up outrageous bills. Similarly, they want to avoid issues with security patches or IT fixes bringing down critical systems during holiday sales. Thus, a measured approach will be required to go from simply supporting and augmenting humans to replacing them across more types of operations.
Planning for autonomous integration: Challenges abound
The challenge for CIOs lies in finding opportunities to extend autonomous capabilities across different types of tools and systems. Each tool used to improve the value of robots, RPA, security and IT will come with its own best practices and data formats.
Similarly, teams will need to identify how improvements across autonomous guidance, scalability and orchestration could be synergistically combined. For example, process mining tools currently used to scale RPA could be adapted to scaling security operations and IT operations. Traditional integration for exchanging data and aligning data schemas is challenging enough. Autonomous systems will also need to align control across disparate systems.
In addition, teams will need to scale their risk management capabilities to identify and plan for unforeseen risks generated by the autonomous systems. A strategy for rooting out bias in AI and increasing AI explainability could help here.
But at the end of the day, it is up to execs to make decisions that benefit the business, employees and society. This extends to the decisions made by autonomous systems. "It's important to determine if the decision the system is making is causing unintended consequences," O'Malley said.
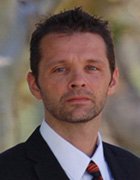
Michel Chabroux, senior director of product management at software provider Wind River, said enterprises need to consider many factors when adopting autonomous systems, including reliability, liability, regulatory issues and societal concerns about potential job losses.
Teams also need to consider the unintended consequences of hyperscale automation run amok.
The paperclip problem introduced by philosopher Nick Bostrom in 2014 illustrates the inherent danger. Bostrom theorized that a superintelligent AI programmed to make paperclips more efficiently could squander other resources and start wars to achieve its goal. A more likely scenario today is that the fulfillment of a short-sighted business goal might accidentally run up a large cloud bill, create an inhospitable environment for employees or impact sustainability goals.
As noted, AI pioneers Geoffrey Hinton and Yoshua Bengio along with 22 other academics have raised new concerns about autonomous AI systems that escape our controls. These could amplify existing AI risks as companies combine generative AI tools to create autonomous AI systems that can plan, act in the world and pursue goals.
"If we build highly advanced autonomous AI, we risk creating systems that pursue undesirable goals," the group wrote in a consensus paper. "Malicious actors could deliberately embed harmful objectives. Moreover, no one currently knows how to reliably align AI behavior with complex values. Even well-meaning developers may inadvertently build AI systems that pursue unintended goals -- especially if, in a bid to win the AI race, they neglect expensive safety testing and human oversight."
Current AI systems have limited autonomy, but this is starting to change. For example, after the release of ChatGPT, hackers quickly developed new add-ons -- including AgentGPT, Auto-GPT and BabyAGI -- that connect to the GPT-4 engine and autonomously browse the web, execute transactions, and use software tools and other AI models.
In the long run, advances in autonomous systems will need to be balanced with tools such as digital twins and the metaverse -- which provide virtual representations of real-world processes and entities -- that provide enterprises a window into how automation is playing out. Improved context will help humans more quickly detect emerging problems and provide better guardrails for autonomous systems that make decisions at scale.
George Lawton is a journalist based in London. Over the last 30 years he has written more than 3,000 stories about computers, communications, knowledge management, business, health and other areas that interest him.