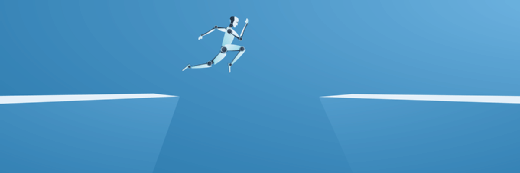
Deep learning pioneer Fei-Fei Li on the fundamentals of ethical AI
AI luminary Fei-Fei Li was among a group of distinguished AI researchers asked to share their thoughts on how to develop ethical AI. The right data and careful observation help.
Business and IT leaders grappling with how to build an enterprise AI program that is effective and ethical might want take a page from the AI pioneers who invented the technology that sparked the current AI boom: It's not about the tool set.
Rather than starting with the goal of building better tools, they began by identifying the kinds of data sets they believed would drive the evolution of better algorithms and techniques. The data sets needed for businesses to create an ethical AI program are no doubt more nuanced and more nebulous than those that drive better AI tools -- in large part because it's unclear what constitutes ethical AI. Still, the approaches used by the world's leading experts to improve AI probably trump simplistic boardroom notions about good vs. bad AI.
At the EmTech Digital conference in San Francisco, AI experts from Stanford and Microsoft elaborated on their methods for developing AI that's designed to have a positive rather than a negative impact on the people, processes and organizations it touches. At a high level, ethical AI involves deep investigation into how AI could fit into existing processes, somewhat akin to the design feeling approach pursued by Google. And it requires input from many perspectives -- not just from computer scientists -- in order to identify potential barriers to AI adoption and to find the areas where AI can make the biggest positive impact.
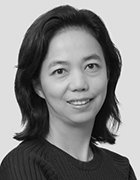
Fei-Fei Li, professor of computer science at Stanford and co-director of the Stanford Human-Centered AI Institute and former chief scientist of AI and machine learning at Google. Meanwhile, former AI executives from Microsoft discussed their explorations of how AI could improve the quality of life in India.
How data sets spawned the AI boom
Li, whose work in AI is credited with developing the algorithms behind the current deep learning revolution, recognized that improving machine vision would require a better data set for researchers to test out algorithms on. So, she led the development of ImageNet, a repository of thousands of images that were labeled by humans, and held an annual contest to improve AI tools for recognizing objects in these images.
Li said that human visual intelligence is one of the key differentiators between humans and other animals. She believes that the rise of vision in animals kicked off the Cambrian explosion millions of years ago, a period when the world's major animal groups appeared. Hawks can see farther than humans, and cats can see at night, Li said. But human brains have evolved to integrate visual input with the ability to communicate and manipulate things with more sophistication. "We have the most incredible visual system that we know of," she said.
With human vision, there is a feedback loop connecting vision, language and manipulation. "You cannot have language without seeing," Li said. She recognized that the richness of vision goes beyond pixels. So, she started work on developing AI to connect vision to language. This started with writing simple annotations for pictures, like "people in a meeting room," for an image of a conference. Now, the research is looking beyond objects to understand activities as well.
Shadowing people, bringing in diverse viewpoints
One of the areas Li is currently excited about is healthcare, which involves lots of human activity from patients, clinicians, caretakers and administrators. The research includes using smart sensors to collect and analyze what people in this setting are doing and combining that data with other data to optimize human activity. "We suddenly find we have the potential to provide technology to help doctors and nurses enhance their job in taking care of patients," she said.
A good part of Li's research involved shadowing doctors and patients across different stages in the healthcare journey in order to understand how people feel across the healthcare system. "The fundamental thing I recognize and find important is that it is all about humans," she said. "At the end of the day, [AI] has to be human-centered."
Li said that one of her principle aims is to find ways to reduce the scientific, philosophical and cultural biases that get programmed into AI. This line of inquiry helped spark the formation of the Stanford Institute for Human-Centered Artificial Intelligence, which has attracted over 200 different colleagues from across Stanford in areas as diverse as law, sociology, UX design and data science to work with AI researchers.
"At Stanford, I recognized we needed to usher in a new era of AI, where it is no longer a computer science discipline; it is a multidisciplinary field with scientists, sociologists, legal scholars and neuroscientists to help us to reimagine what AI is," she said.
Having experts from diverse disciplines, however, is not enough to develop ethical AI programs. Diversity is also needed. To that end, Li helped launch the AI4All program to train more women to be AI experts. The program is also now being extended beyond Stanford to campuses all over the country.
An important element of developing ethical AI programs is to more fully understand the technology's impact on human society. "We see AI as a technology that will augment, amplify and enhance humanity and not replace it," Li said. So, part of her team's work involves showcasing applications in fields like healthcare and manufacturing that will demonstrate a positive role for AI.
Li said people involved in AI must recognize that there are no independent machine values. The value behind algorithms is really about the users, practitioners, developers and business managers involved in their development and use. Every AI algorithm -- whether used for self-driving cars, healthcare or recommendation engines -- can have both positive and negative effects on people. "With every one of [these AI applications], we need to get the people who will be impacted to decide what is the value" of AI augmentation, Li said.
Developing a model for good AI
Over in India, the Wadhwani Institute for AI is exploring different ways that AI can be applied to social good. Its CEO, Padmanabhan Anandan, came from Microsoft, where he was founder and director of the Microsoft India Research Lab and led Microsoft's computer vision research. In an interview with SearchCIO, he explained that implementing AI requires going beyond just deploying better algorithms. It requires doing extensive exploration of existing cultures, institutions and processes to find where AI can be adopted in a way that makes a positive difference for everyone.
In the case of the Wadhwani Institute for AI, the first implementations all involved using machine vision to improve different processes around maternal care, agriculture and tuberculosis management. For maternal care, the research team created an app that enables fieldworkers to virtually "weigh" newborns without having to pick them up. This helps prioritize assistance for low birth weight babies. Other approaches using scales did not work well because cultural prohibitions prevent many Indians from letting nonfamily members touch their babies for a month. And when the parents weighed their own kids, a suspiciously large majority of babies met the exact 2.5 kilogram cutoff for not qualifying as low birth weight.
Another machine vision app counts the number of bugs caught on cardboard glue traps with a smartphone app. This promises to reduce the amount of time to identify new infestations and help government officials prioritize remediation strategies when they can still make a difference. Last year, over 1,000 cotton farmers committed suicide after a widespread pestilence killed many crops.
A lot of Wadhwani's work involves talking with social sector organizations and governments, which typically have a poor understanding of AI. "By building these partnerships, we are hoping to learn how to work in those domains and build a network of partners that are receptive to AI," Anandan said. "The point solutions are a way of think[ing] about how we can have an impact on the larger system."
In the same way, executives might consider early AI projects as a learning process for the organization to identify how AI might have an impact, both inside the organization and in the world at large.