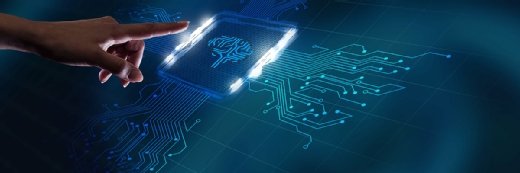
putilov_denis - stock.adobe.com
Empower intelligence at the edge with AI deployment
AI deployment at the edge has the potential to unleash powerful real-time analysis and processing. Use cases include industrial automation, remote monitoring and healthcare.
Edge deployments of AI refer to the practice of deploying AI models and algorithms on edge devices, or local servers, rather than relying on cloud-based processing. This approach brings AI capabilities to where data is generated, which results in faster and more efficient processing, real-time analysis and reduced dependence on internet connectivity.
The concept of edge computing forms the foundation for AI deployments at the edge. It involves distributing resources and data storage to the network's edge where data originates. Embedded devices, such as smartphones, IoT devices, cameras and drones, serve as platforms for deploying AI models.
Edge deployments facilitate real-time decision-making by enabling real-time analysis of data streams without relying on cloud connectivity or external servers. This localized processing addresses concerns about data privacy and security because information doesn't need to be transmitted to the cloud. Analyzing data on the edge device itself reduces access risks or potential data breaches.
Edge AI deployment prioritizes the transmission of insights or summarized results to minimize network congestion and reduce latency. It uses hybrid architectures to combine the use of edge deployments and cloud-based processing, which creates a distributed system.
Edge deployments enable customization and adaptation of AI models to meet the requirements of edge devices, applications or users. Models can be adjusted to optimize their performance and efficiency according to the limitations of the edge hardware. Additionally, edge deployments enable distributed learning, which involves AI training models, across multiple edge devices without centralizing data. This approach ensures privacy and preserves model training, while taking advantage of data sets.
Advantages of edge AI deployment
AI deployments at the edge offer benefits that make them appealing choices for a range of applications across industries like healthcare, manufacturing, transportation, surveillance and smart cities.
Let's explore the advantages of AI edge deployments.
Real-time decision-making
By processing data on edge devices, AI algorithms can provide real-time decision-making. This capability is important in use cases with vehicles, industrial automation and critical infrastructure monitoring, where immediate insights are crucial for safe and efficient operations.
Data stream analysis
Edge deployments enable efficient analysis of data streams. By processing data on edge devices, AI models can deliver insights and predictions. This proves advantageous in applications that require prompt actions, such as fraud detection, anomaly detection, predictive maintenance and monitoring systems.
Privacy and security
Edge AI deployments bolster data privacy and security measures. Instead of transmitting data to the cloud for processing, AI algorithms operate locally on the edge devices. This minimizes risks associated with data exposure during transmission and addresses concerns related to data privacy regulations. Critical data remains within the confines of the network, thereby improving security.
Less data transmission to cloud
Edge deployments minimize the need to send large volumes of data to the cloud. By processing and filtering data, AI edge deployments send only the relevant insights or summarized results. This optimization helps make optimum use of network resources, lowers transmission costs and eases network congestion.
Less reliance on internet connectivity
Edge AI enables AI applications to work offline or in environments with intermittent internet connectivity. AI models are directly deployed on edge devices, which enables them to perform processing without relying on a connection to the cloud. This ensures that AI capabilities remain accessible and operational even when a reliable network connection isn't present.
Flexibility and customization
Edge deployments offer the flexibility to customize and adapt AI models according to specific edge devices, applications or user requirements. AI models can be tailored to fit the limitations and capabilities of edge hardware. This adaptability results in improved performance, reduced resource usage and optimized energy efficiency.
5 real-world applications of edge AI deployment
Here are some practical applications where deploying AI at the edge brings benefits.
1. Autonomous vehicles
Deploying AI at the edge is crucial for autonomous vehicles, as it enables real-time processing and decision-making for safe navigation. Using AI algorithms on devices within the vehicle helps enable real-time perception, object recognition and collision avoidance. This reduces latency and real-time responsiveness.
2. Industrial automation
The deployment of AI at the edge is widely used in factory automation to enable real-time analysis and control. Equipping edge devices with AI models helps optimize manufacturing processes, detect anomalies, predict equipment failures and enable maintenance. This leads to increased efficiency, reduced downtime and cost savings.
3. Remote monitoring
The deployment of AI at the edge enables monitoring of infrastructure and remote locations. For example, in oil and gas pipelines, edge devices equipped with AI can perform real-time analysis of sensor data to detect leaks, abnormal conditions or security threats. Similarly, in environmental monitoring scenarios, edge devices can analyze sensor data to track air quality levels, weather patterns and natural disaster events.
4. Healthcare
Deploying AI at the edge holds value in healthcare settings, such as remote patient monitoring applications, real-time diagnostics and personalized healthcare. Edge devices, such as medical sensors, have the capability to analyze information directly on the device itself. This enables identification of any health irregularities and timely sharing of insights with healthcare professionals. As a result, it facilitates healthcare interventions and reduces the reliance on constant cloud connectivity.
5. Surveillance systems
Deploying AI on edge devices is also valuable for surveillance systems, as it enhances real-time threat detection and response. Equipping edge devices with AI models enables video feeds to be analyzed locally to identify activities and trigger alerts or actions. This eliminates the need for video streaming to the cloud. This could improve the overall efficiency and effectiveness of surveillance systems.
Efficient data management in edge AI deployments
Data management plays a crucial role in edge deployments, as it ensures processing efficiency, reduction in bandwidth usage, and maintenance of data security and privacy. Let's look at the importance of data management in edge deployments and how edge devices handle tasks like data storage, synchronization and security.
Pre-processing data
Edge devices often receive noisy data from sensors or IoT devices. Techniques like noise removal, data cleaning and normalization help improve the quality of data analysis. These methods not only optimize bandwidth usage, but enhance the efficiency of subsequent analysis.
Filtering data
Edge devices can perform initial data filtering to extract information or detect events of interest. By doing this, they ensure that only valuable or significant data is transmitted to the cloud or local servers. This helps reduce network traffic and minimize latency.
Summarizing data
Summarization techniques are employed at the edge to condense data sets into compact representations. These summarized representations provide a format that can be transmitted to the cloud for analysis or stored locally with bandwidth requirements.
Storing data
Edge devices need to manage storage for temporary or offline operation because their storage capacities might be limited compared to cloud servers. Therefore, effective management of data storage becomes crucial in edge scenarios.
Synchronizing data
It's critical to synchronize data in situations where edge devices have limited network connectivity or operate offline. Edge devices sync their data with either the cloud or local servers whenever they establish a connection.
Securing data
Comprehensive security measures are essential for edge deployments to safeguard information. Edge devices employ encryption techniques, access controls and secure protocols to ensure the security of data during both transmission and storage.
Keeping data private
Data privacy in edge deployment scenarios is important, especially when handling sensitive or personal information. Edge devices must comply with privacy regulations and implement methods, such as data anonymization and differential privacy, to protect individuals' identities and maintain data confidentiality.
Unlock the potential of edge AI deployments
Overall, deploying AI at the edge holds promise in fostering innovation, improving efficiency and enabling real-time decision-making across industries. With research and technological advancements in this field, expect to see a revolution in how AI is applied. This shift can empower organizations to take advantage of their data, while implementing privacy, security and seamless integration into existing infrastructure.
About the author
Saqib Jang is founder and principal of Margalla Communications, a market analysis and consulting firm, with expertise in cloud infrastructure and services. He is a marketing and business development executive with over 20 years' experience in setting product and marketing strategy and delivering infrastructure services for cloud and enterprise markets.