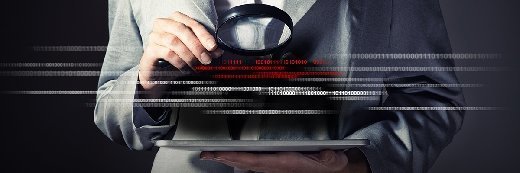
adam121 - Fotolia
Cognitive hacking: Understanding the threat of bad data
Bad data can create more than just 'fake news.' Expert Char Sample explains how cognitive hacking and weaponized information can undermine enterprise security.
One of the biggest security stories from the 2016 presidential election was not a breach of voter databases, the suspected hacking of the voting machines or the vote counting. Instead, the biggest security story was the use of weaponized information in support of cognitive hacking.
The term cognitive hacking was defined in a 2002 Dartmouth College research paper that described it as a cyberattack designed to change human users' perceptions and corresponding behaviors. Setting aside the political discussion, the real reason for this interest is that security software is vulnerable to the same problem. That is, the data entered into security products, whether by a human or a machine, is trusted to be a faithful representation of reality.
The goal of weaponized information in support of cognitive hacking is the manipulation of user perception, and the tool is the data. One type of data is information that is fully false. Other types of weaponized information may contain data that is partially true; fully true, but taken out of context; or true, but the timing of the data release is done to distract, disrupt or cause distrust. In all of the aforementioned cases, the content of the message, the data, is the weapon.
This is something retired Air Force Col. Richard Szafranski identified as a possibility in his 1995 paper titled "A Theory of Information Warfare," and it has been witnessed sporadically, such as with Stuxnet and other malware associated with the most sophisticated nation-state attacks.
In the physical world, events can be verified through the combined use of the five senses. Seeing, hearing, smelling, touching and tasting verify perceptions gathered by the other senses. In the virtual world, sight and sound are the most commonly used senses, and machines are trusted to create and relay this information. Users generally trust the machine, and bad data relayed from a bad machine is initially trustworthy until proven otherwise.
Bad data in the physical world can be verified by an organization like Reuters, academia and other institutions capable of finding and reporting the entire truth. The digital environment does not contain these sorts of institutions. An implicit assumption made when a user is validated is that the user will enter faithful or honest data. When the computer or other machine enters data, this too is assumed to be good, faithful data.
Consider both of these assumptions: The user who willingly chooses to enter bad data may have access revoked, but that user could just as easily have bad data when entering the identification data; as a result, revoking access would simply result in the user assuming a new identity. On a larger scale, this behavior is witnessed in domain name system fast flux behaviors. Similarly, a trusted, but compromised machine or device can also send misleading data, as was demonstrated by Stuxnet and Duqu. In both cases of user and machine, by the time the problem had been traced back to the source, the damage was done.
Current solutions
The existing solutions to this problem are insufficient. Take the example of fake news: The solutions for dealing with it involve researching the information or the reputation of the information source and curating it accordingly. The first issue with researching the information is the time factor. In the case of fake news, users are expected to enact a series of steps, such as checking with other reputable news sources or fact checking organizations. This can become rather time-consuming when a story contains multiple links and data sources.
Similarly, we see the same problem with security alerts. Operators are already overloaded sorting through security data, and adding to the workload will introduce new problems. Determining the cause of a security alert is still, in spite of security information and event management technology, a time-consuming task. An operator must settle into the environment and learn the unique characteristics of that environment. In this case, training data is used to prep the operator rather than the machine.
The second issue is more troubling, since it deals with researching the accuracy of the data. Think again of our fake news example. By planting fake news on several sites, it can become validated just by being mentioned on a mainstream news site. Similarly, when bad data is repeated by other sensors or monitors, even through basic propagation, the bad data can be viewed as good data. This is an instantiation of the Byzantine Generals' Problem.
One solution to the fake news problem involves a type of signature approach with reputation analysis. In response to fake news, various services can be installed as browser plug-ins, and when links to known fake news sites appear, the questionable websites can be identified on the displayed webpage. Of course, a new and unknown site, much like zero-day attacks, can easily slip through this filter.
The same processing occurs with security products. The problem with signature software has long been documented, and reputation analysis, while more inclusive than signatures, uses the same flawed post hoc model. Therefore, reputation analysis products are also vulnerable to the emergence of new, unrecognized entities creating bad data, exemplified by fast flux behaviors staying ahead of the filters.
Input validation tools are helpful, but due to the diverse number of clients that must be supported, only very basic input validation can be handled. Thus, these tools are good for handling the obvious attacks, such as buffer overflows, but not the more sophisticated, subtle attacks that involve entering inappropriate strings. Furthermore, these tools are often unable to discern the veracity of the data being entered; instead, they implement standard good software rules. While they certainly need improvement, these approaches represent a significant step in a long journey to overcome cognitive hacking and weaponized information.
Heuristic input validation provides a better solution to the problem than basic input validation, but like any other heuristic approach, the learning environment must be clean. If the learning environment is not clean, these tools will interpret bad data as normal. This problem is present in both unstructured and structured learning environments because it reflects the deeper problem of improperly baselined systems.
The problem with all of these proposed solutions is the fundamental assumption that the old garbage in, garbage out model is a sufficient deterrent to the spread of bad data. This model assumes the party that enters the data desires a normal outcome. When the party that enters the data intentionally enters bad data, the model breaks down. We still see this today when new bugs are discovered and a new set of rules are enacted. These rules can be signature-based, behavioral-based or based on any number of criteria, but they are all a response to an unanticipated input.
The Dartmouth College paper, titled "Cognitive Hacking: A Battle for the Mind," identified different methods for dealing with the problem of intentionally generated bad data. Historically, reactive measures have been the most common method for dealing with cybersecurity problems.
The emergence of Byzantine models were effective for some time, but in the case of a sophisticated attack that relies on false information, which is the same issue we are seeing with fake news, polluting of other sites used for verification will present problems in the security of data. Thus, understanding the context in which data is created and sent is of great importance.
Editor's note: Stay tuned for the next article in this series on cognitive hacking and data fidelity.
About the author:
Dr. Char Sample, a cybersecurity researcher and fellow at ICF International, has 20 years of experience in internet and information security. She previously served as a research scientist and security solutions engineer with CERT at Carnegie-Mellon University. In addition to her role at ICF International, Sample is a visiting researcher and international fellow for cybersecurity at the University of Warwick in the U.K.