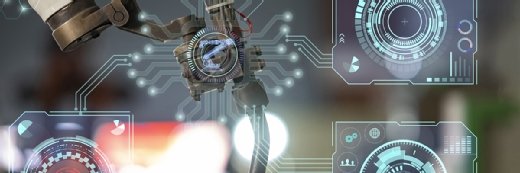
Getty Images/
Patient Deterioration Predictor Outperforms Vital Sign Measurements
Researchers at the University of Michigan have developed an artificial intelligence model that can accurately detect and predict patient deterioration.
A recently developed artificial intelligence (AI) model out of the University of Michigan (U-M) can predict hemodynamic instability, a key indicator of patient deterioration, more accurately than traditional vital sign measurements.
Hemodynamic instability is defined in the press release as a combination of elevated heart rate and low blood pressure, and hemodynamic assessments are often used to measure patient deterioration.
However, patient monitoring typically relies on intermittent static vital sign measurements, which limits early detection of potential deterioration, according to the press release. Traditional vital signs also have limitations, including limited accuracy in non-invasive monitoring.
To combat these limitations, researchers sought to develop an AI model that could accurately predict hemodynamic instability in real-time. The researchers gathered data from over 5,000 adult patients at U-M Health, which they used to train and develop the Analytic for Hemodynamic Instability (AHI).
AHI is a software as a medical device designed to detect and predict changes in hemodynamic status in real-time using data from a single electrocardiogram (ECG) lead. The researchers compared its performance with that of the gold standard for measuring patient deterioration: vital sign measurement of continuous heart rate and blood pressure measured by invasive arterial monitoring.
AHI outperformed the vital sign measurement, achieving a 97 percent sensitivity and a 79 percent specificity. These findings, the study authors stated, suggest that the AHI may be able to provide continuous dynamic monitoring capabilities in patients who traditionally have intermittent static vital sign measurements.
“AHI performs extremely well, and it functions in a way that we think may have transformative clinical utility,” said Ben Bassin, MD, associate professor of emergency medicine at U-M Medical School and senior author of the study. “Most vital signs measurements are static, subject to human error, and require validation and interpretation. AHI is the opposite of that. It’s dynamic, produces a binary output of ‘stable’ or ‘unstable,’ and it may enable early martialing of resources to patients who may not have been on a clinician’s radar.”
The model was approved by the FDA in 2021, the press release noted. The next phase of AHI research will focus on how U-M Medicine uses the model, and the researchers stated that future studies are needed to determine if AHI provides clinical and resource allocation benefits in patients undergoing infrequent blood pressure monitoring.
“The vision of AHI was born out of our continued inability to identify unstable patients and to predict when patients would become unstable, especially in settings where they cannot be intensively monitored,” said co-author Kevin Ward, MD, executive director of the Weil Institute and professor of emergency medicine and biomedical engineering at U-M Medicine, in the press release.
“AHI is ideally suited to be utilized with wearable monitors such as ECG patches, that could make any hospital bed, waiting room or other setting into a sophisticated monitoring environment,” Ward added. “The implication of such a technology is that it has the potential to save lives not only in the hospital, but also at home, in the ambulance and on the battlefield.”