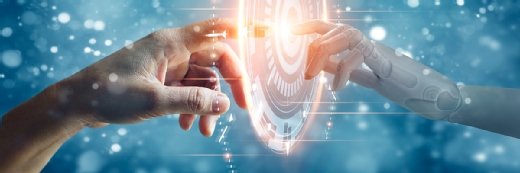
Getty Images
New ONC Challenge Promotes Big Data Analytics Development
The Synthetic Health Data Challenge will support researchers and developers in testing the effectiveness of big data analytics tools and algorithms.
The Office of the National Coordinator for Health Information Technology (ONC) has launched the Synthetic Health Data Challenge, an effort to encourage the development of big data analytics tools using realistic – but not real – health record data.
Synthetic health data contains a complete medical history from birth to death. This data can be used without cost or restriction and is meant to support the specific interests of researchers and developers testing the effectiveness of tools, data analytics algorithms, and disease modeling approaches.
The challenge is part of ONC’s Synthetic Health Data Generation to Accelerate Patient-Centered Outcomes Research (PCOR) project. Through the Synthetic Health Data Challenge, participants will create and test innovative and novel solutions to further refine the capabilities of Synthea, an open-source synthetic patient generator that models the medical histories of synthetic patients.
Synthea can use publicly available health statistics and other research resources to support a variety of academia, research, industry, and government initiatives. Because the software uses publicly available statistical data to generate synthetic data sets, the barriers to resource availability and privacy concerns are lower than they are for other synthetic data generation technologies.
The Synthetic Health Data Challenge encourages researchers and developers to validate the realism of synthetic health records generated by Synthea, develop or improve the disease-progression and treatment modules used to create synthetic records, and spur novel uses of synthetic health data.
"Synthetic data like those created by Synthea can augment the infrastructure for patient-centered outcomes research by providing a source of low risk, readily available, synthetic data that can complement the use of real clinical data," said Teresa Zayas-Cabán, ONC chief scientist.
"By enhancing Synthea with new clinical data modules or demonstrating novel uses of Synthea-generated synthetic data, Challenge participants will support PCOR research and development efforts by enhancing PCOR researchers' ability to conduct rigorous analyses and generate relevant findings."
Participants can submit their challenge Phase I proposals in one of two categories: Enhancements to Synthea or novel uses of Synthea generated synthetic data. The best proposals will move on to Challenge Phase II, prototype or solutions development.
Phase II will feature awards totaling up to $100,000, with up to two first-place winning solutions receiving $25,000 each; up to two second-place solutions receiving $15,000 each; and up to two third-place solutions receiving $10,000 each.
The newly-announced challenge is just one in a portfolio of projects led by ONC to enable PCOR through technology. The agency’s Synthetic Health Data Generation to Accelerate PCOR project aims to enhance the ability of Synthea to produce high-quality synthetic data for opioid, pediatric, and complex care use cases.
The project will reach its goal by developing opioid, pediatric, and complex care data generation modules for Synthea to increase the number and diversity of synthetic patient health records to meet PCOR needs. Additionally, the project will seek to engage the broader community of researchers and developers to validate the realism and demonstrate potential uses of the generated synthetic health records through a challenge.
Recently, leaders in the healthcare industry have turned to synthetic data to accelerate research and insights into patient health. A team from Washington University partnered with government research centers and other healthcare systems to overcome data privacy challenges using synthetic data.
“It's safer for our patients. And the time to insights is greatly reduced because we don't have to go through all the laborious regulatory processes that are normally associated with analyzing potentially identifiable patient data,” Philip Payne, PhD, associate dean for health information and data science and chief data scientist at Washington University, told HealthITAnalytics.com.
“We take that process that previously took weeks, months, or maybe a year, and we turn it into minutes or hours. That's incredibly important when we try to use these advanced data analytics methods to arrive at important conclusions about the data that we have and to produce timely results.”