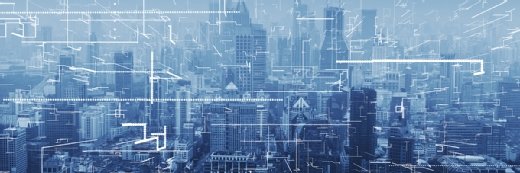
Getty Images/iStockphoto
Analytics software unlocks the benefits of IoT data collection
IoT data is useless if it can't provide operational insights. Analytics offerings should be device-flexible and have visualization features to make data useful to stakeholders.
In recent years, IoT technologies have been hailed as an important enabling infrastructure for Industry 4.0, and there are already more than 7 billion connected IoT devices today, with this number expected to triple by 2025.
With all the data that these devices currently -- and will -- generate, there is now a real need for processing this data in a way to gather meaningful insights at scale. Examples include sensor data recorded as points in time-series and rich multimodal data -- such as vision and speech -- that is uploaded to the cloud or even processed using edge computing as technology becomes cheaper and faster.
With these growing data processing requirements, there is now as pressing a need for IoT analytics solutions as for the devices themselves.
A look at data sources
There are several stages of IoT data collection, depending on the use case; the types, origins and properties of the data can significantly differ.
For example, when considering IoT in smart offices, the goal usually is to improve productivity, security and workplace safety. Energy monitoring and savings devices, such as a commercial smart thermostat, tend to play a leading role in such deployments, and the data they collect is often localized to specific offices, conference rooms or floors. A comprehensive analytics software may not be required in such settings, and indeed, the quality, cost and fidelity of the devices themselves become more important concerns.
In contrast, industrial sectors, including factory floors, are likely to have device clusters in a location, and the data is also multigranular and heterogeneous.
Other examples include retail, where data can be collected from weight sensors on shelves, which help inventory control and provide real-time alerts, as well as healthcare, where even wheelchairs are equipped with IoT sensors.
Across all sectors, there are creative uses for devices -- and the data they collect -- that are likely unknown at this point.
IoT analytics software is necessary
To make full use of data being generated in this environment, as well as enable truly cost-effective optimizations and efficiencies, companies need to invest in an IoT analytics software that has the following features:
- Is business-ready. The software must come with all the necessary features to ensure that the organization can facilitate an entire IoT data analytics operation. Ideally, the offering is available as SaaS and provides privacy, security and customer support guarantees that are essential to the functioning and sale of enterprise software.
- Is device-flexible. The IoT analytics offering must be able to ingest data from a variety of devices and not be overly constrained or specialized given how rapidly IoT is expanding and changing. There is a natural tradeoff between how specialized a software is to an industry or enterprise and how well it can scale to other industries. The leaders in this space need to be able to navigate these tradeoffs effectively to maintain their incumbency. Startups in the IoT analytics space should explore this sector's underserved niches but must eventually address scaling concerns.
- Incorporates machine learning. While much of basic analytics, and even visualization, can be accomplished without machine learning, its integration into the analytics pipeline is essential for organizations to achieve greater efficiency and optimization. Analytics software that makes machine learning seamless has a competitive advantage.
- Uses advanced models, such as explainable AI. Machine learning and AI are not the same thing, although they are often conflated with each other. Explainable AI that may draw on machine learning -- but is distinct from it -- will be important for IT teams that do not wish for a black box of machine learning models that tells them what to do, but instead provides reasonable explanations that underly any recommendations.
The incorporation of explainable AI will enable startups and smaller companies to break into the IoT analytics market and charge a premium for their services. Potentially, companies offering IoT-specialized explainable AI could partner with more traditional IoT analytics vendors that have advantages of scale in software testing, delivery and marketing. - Offers dashboarding, visualization and report generation. Visualization has always been an important, and frequently underestimated, component of analytics pipelines. A good platform must support visualization and products that are a direct result of visualization. More advanced software options enable operators to quickly and easily generate reports based on the visualization and data and help business leaders make decisions within short cycles.
Looking ahead at IoT data collection and analytics
An exciting industry trend is the push toward greater standardization of connected devices that comprise industrial IoT, home smart devices and internet-connected appliances. Such standardization is inevitable in the next two to three years. Due to this trend, there is an expectation analytics software will become less expensive and more interoperable in the next few years.
Executives may decide to wait before they invest in a software that is costly in the near future only to discover it is superseded by a superior option. Instead, the next year could be better spent on planning, budgeting, strategy and training. For a company that heavily relies on IoT, waiting may not be an option, but executives should be wary to buy into longer-term contracts for existing software offerings.