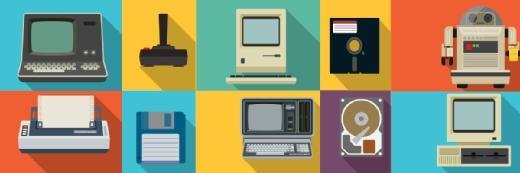
Fotolia
Is single-point sensing the next-gen IoT sensor network?
Single-point sensing systems help developers use advanced algorithms to replace the number of sensors in a network. But it's not a fail-proof method -- yet.
Many silicon vendors, device makers and enterprises have been pursuing a strategy that assumes businesses and consumers will want to place devices everywhere for monitoring. While this strategy has some merit, as the cost of monitoring devices decreases, it still comes with associated management, power and security overhead for each device installed.
An alternative single-point sensing approach around the concept of infrastructure-mediated sensing lies in deploying an IoT sensor network of relatively fewer sensors with higher telemetry resolution, coupled with back-end cloud analytics. This approach promises to reduce cost, management and user challenges, and it's already seeing some traction for replacing a network of power monitoring devices with a single device situated at a main power box.
Another example of an IoT sensor network that uses single-point sensing is microphones that detect windows breaking. A single microphone, for example, would reduce the need to rely on dedicated sensors on each window in terms of security.
Early work on infrastructure-mediated sensing
In the early 2000s, led by Washington Research Foundation Entrepreneurship Endowed Professor Shwetak Patel, researchers at the University of Washington began experimenting with strategically placed sensors to monitor the electricity usage of each appliance in a house based on their electrical signal. Not only could they see if a light went on, but they found a lightbulb in the living room had a slightly different signal than one in the kitchen due to the topology of the wiring. Patel envisioned this new type of ubiquitous compute-sensing infrastructure could improve elder care, electrical consumption monitoring, home automation and entertainment.
Researchers coined the term infrastructure-mediated sensing because it uses a single sensor on a type of system to monitor events throughout the house. Similar approaches were used to monitor water usage and gas appliance usage. Researchers even found a single air pressure sensor in an air duct could track human movements throughout the house.
Through single-point sensing, a single sensor in a strategic location can measure pressure signals in air vents and waterlines, as well as electrical signals on power lines. Machine learning technologies are used to correlate physical events, such as turning on a light, flushing a toilet or opening a door, with different signals. One of the primary goals of single-point sensing systems is to reduce the economic, aesthetic, installation and maintenance barriers by reducing the cost and complexity of deploying and maintaining an activity-sensing infrastructure, Patel said.
One key to making this work lies in taking high-resolution recordings from the infrastructure. While power meters quantify usage in 15-minute increments, these sensors would record minute variations at 1,000 Hz intervals to measure signals unique to each appliance. Researchers also spent considerable effort creating new machine learning algorithms to convert these signals into events corresponding to power usage of each appliance.
False start to commercialization
Single-point sensing worked well on test homes engineered for the research, but the initial research didn't lead to any commercial products immediately. The core technology was baked into a new startup, called Zensi, which was sold to Belkin in 2010 as part of its home gateway division. Belkin improved the core algorithms, and it even sponsored a competition on data science community website Kaggle to provide developers with incentives to craft more accurate algorithms. But Belkin has not launched any commercial products based on infrastructure-mediated sensing.
The Energy Detective, a competitor that makes a power monitoring device, pursued a similar single-point sensing approach before declaring individual appliance monitoring from a centralized point is a myth. According to a company FAQ, "While it worked well for large appliances like an HVAC/water heater, it was extremely inconsistent and frustrating for smaller appliances." The company ultimately pursued an alternative approach to measuring each outlet from the utility box.
New entrants move single-point sensing forward
More recently, interest in infrastructure-mediated sensing began making a comeback, in part, due to better algorithms, improved machine learning infrastructure in the cloud and simplicity. The initial infrastructure-mediated sensing algorithms were optimized using neural networks or machine learning algorithms running individual PCs. But the development of AI toolchains by Google -- and later others -- has made it much easier to implement massive neural networks in the cloud. Furthermore, the growth of IoT devices and better communications protocols make it much easier to gather more data from consumer and business users to further improve these algorithms.
Startup Neurio developed the Neurio Home Energy Monitor and associated software to monitor energy usage in the home from the breaker panel. The company took a much less aggressive approach in only characterizing energy usage patterns of more power-hungry appliances, like air conditioning, heaters and dryers. The electrical signals of large appliances tend to be much easier to decipher, and there are fewer of them. These represent the low-hanging fruit in terms of measurement, but they also have a more significant impact on energy consumption than individual appliances or light.
Another startup, home-monitoring company Sense, has taken a much more aggressive approach in creating an app to quantify energy usage at the appliance level in the home. The Sense module plugs clip onto the circuit breaker box in a user's home. The device sits in the background, learning the behavior of someone's appliances. When it sees an electrical signature for a device it doesn't recognize, it simply records it. Users can then name it after the fact, and it will continue to track the usage of this device over time.
Sense also developed Network Device Identification, a new data collection tool that listens for communication of devices in the home via Bluetooth and Wi-Fi networks sent via Simple Service Discovery Protocol and Universal Plug and Play, Zeroconf and ARP protocols used for IoT communications. These messages are used by devices to broadcast their knowledge about their state. The Sense device also periodically sends out a ping to prompt devices that might not be actively broadcasting their status. This allows Sense to better correlate changes in a device's state with changes in the electrical signals it receives to improve its algorithms.
The pings allow Sense to accurately register that a smart speaker is off, turned on in standby or actively playing music, for example. Sense data scientists can then more easily clarify electrical signals generated by devices silently on in the background. The company plans to use this data to improve device detection for the community of Sense users.
In order to deal with variability, the Sense device needs to collect several examples of a targeted object, like a garage door opener, and of competing objects it doesn't want to confuse with the target. These processes use algorithms such as deep learning or Hidden Markov modeling to reliably recognize an object of interest. Recognition depends on the samples representative of a target class of objects and other potentially confusable objects.
For example, a garage door has a signature, with similar wattage traces and durations representing the different phases of the door opening, sliding against obstructions and the rail, then stopping. In contrast, something like a heater has a much different signature because of the resistive load of its coils. Another load from a large motor would have a different electrical signature caused by the characteristic rhythm of the motor load. The app might recognize a signal is from a heat-oriented device or a motor-oriented device. Humans can then characterize these mystery signals. The data is then fed back into Sense's cloud app to help improve the recognition of similar devices in other homes or offices.
More recently, companies are beginning to use the principles of infrastructure-mediated sensing in other areas, as well. For example, the SwannOne home security system uses single-point sensing via a microphone on each floor to listen for the sound of breaking glass. This provides one more level of security when homeowners have not installed detectors on each window.
Going forward, it is conceivable similar capabilities could be baked into modern home assistants, like Google Home or the Amazon Echo. Developers could craft apps that run on these devices to monitor security events. Algorithms for detecting these events could be improved using machine learning algorithms running in the cloud.
The future of infrastructure-mediated sensing
Another promising aspect of infrastructure-mediated sensing is in extending the abilities of infrastructure-mediated sensing systems for industrial applications using more active sensors. This would build in traditional testing approaches using a kind of radar relevant to the type of infrastructure being monitored. Telephone and network installers have long relied on time-domain reflectometry that sends a signal down a telephone or local area network wire, and water pipes use high-frequency ultrasonic sounds to find leaks and broken faucets in water systems.
Infrastructure-mediated sensing is not a perfect system. While better algorithms can greatly improve the accuracy of single-point sensing, this approach tends to be limited in relying on a single signal of measurement. Going forward, researchers and businesses are going to have to look at how to use information from different types of sensors to significantly increase the accuracy and precision of event measurements from the single sensors used in traditional infrastructure-mediated sensing architectures.