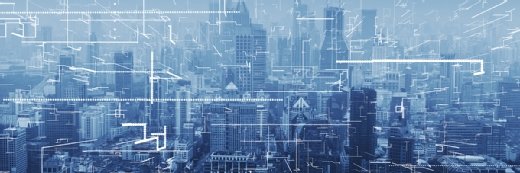
Getty Images/iStockphoto
How edge computing can ease IoT adoption
IoT brings helpful insights to organizations, despite concerns around ROI, skill availability and upgrades. Edge computing offerings are one way to address these challenges.
A lot has been written about the IoT revolution and how the technology has the capability to revolutionize industries, transform productivity and unlock new levels of insight. But, for those intrigued by the possibilities and looking to dip their toe in the water, the potential myths of high price point, infrastructure and connectivity challenges, as well as the required skill set, can be significant hurdles that seem insurmountable.
When it comes to the reality of industrial IoT (IIoT), many organizations must consider the cost, time and disruption that goes into a new facility. The prospect of having to rip and replace new infrastructure to support IoT isn't a viable option for many businesses.
Address IoT implementation challenges
Edge IoT and analytics can provide a powerful mechanism for translating complex data sources into a streamlined, lower-cost platform with faster ROI and higher value. However, there are five key challenges businesses face when considering an IoT implementation.
1. Investment
The transformational potential of IoT across multiple industries is staggering and much has been discussed about its power to revolutionize business models. But, while the possibilities for market sectors are hugely exciting, the reality of many of these industry IoT offerings is that they are designed for vast use cases -- the setups are intricate and complex, with incredibly powerful networking capabilities that require significant investment and skill to execute.
The major players in the IoT space, including AWS and Microsoft, require huge upfront investment into IoT stacks and other hardware integrated into the data center, as well as personnel who can code the solution, write it and build it -- that's potentially hundreds of thousands of dollars even before an organization gains potential data or insight.
ROI is something that the IoT space lacks, causing proof of concepts to fail. One early use case for IoT -- smart meters -- is an instance where it's simple to work out the ROI as organizations don't have to send meter readers to sites and there is an immediate cost benefit.
But, with IIoT, it's much more than that. Perhaps, it exposes some savings, and maybe less machine maintenance is required. Savings are harder to identify at the outset; therefore, large upfront investment in that kind of solution is difficult to justify under those circumstances.
2. Rip and replace
In a lot of industrial cases, the existing machinery that requires monitoring includes large, complex and expensive structures. These machines are suited and built for the task at hand, and for that reason, they should be monitored in a noninvasive way.
Many facilities have been designed and built at the cost of billions of dollars, and organizations can't start ripping and replacing components because cloud-enabled technology provides a benefit that hasn't been quantified yet.
Conversely, many of the IoT offerings out there from the market depend on IoT being built into infrastructure from the start -- a concept that could result in significant business disruption and downtime.
3. Skill sets
The skill set that is required to manage these types of complex setups is also a significant hurdle for many organizations. A high proportion of IoT customers in manufacturing are not necessarily IT-savvy in the way that traditional database users are. With many providers that require someone that can effectively manage these platforms, this is an issue that is damaging the chances of adoption in that sector.
Businesses need a way to get data out of the IoT devices without the complex ecosystem that surrounds them through a streamlined platform that only needs a browser to access. This means that organizations must figure out if they can afford to hire a dedicated IoT professional and how that role can provide value.
4. Infrastructure
Another stumbling block for many IoT projects is the infrastructure not being developed if the location is in an inconvenient place without reliable Wi-Fi -- the only clouds available are the ones floating in the sky. In this case, having an IoT solution that collects all the data, analyzes it at the collection point, and enables rapid and reliable visibility of what's going on can make all the difference and is a much more pragmatic solution, both in large factories and distant locations. That's the difference between the original vision of IoT and what it is in practice.
5. IoT at the edge
The vision of IoT and reality are substantially different. A sensor's yes or no response is different than deciding if a complex piece of machinery is acting as it should and at optimum efficiency levels. It's not only about the opportunity to collect data, but also having the capability to modify that data collection and to add additional sensors to expand the data gathered even further.
For example, it could be that the setup monitors temperature and speed but then must measure vibration. This requires another sensor, so the platform must be adaptable and scalable. In the current industrial sector environment, IT teams must be flexible and ready to change in scale, both in terms of size and complexity of gathered data.
As edge computing, which analyzes data at its creation point, is gaining momentum, organizations are discovering how they can quickly access only the most valuable data in real time that proves mission-critical to their business.
Coming back to the smart meter example -- this type of IoT deployment involves millions of identical devices with the same data and a single purpose. It's still an investment, but the principle is simply connecting multiple homogeneous devices together. This is unlike today's industrial environment, where there could be a handful or even tens of thousands of different devices, all doing slightly different tasks in different ways.
This specialized equipment, therefore, requires an IoT edge solution that can accurately translate, measure and analyze different data formats as the data arrives without having to rip and replace the machinery's internal electronics.
Edge enables data processing to be performed on the edge nodes prior to transmitting only the aggregated data to the central server. Instead of transmitting huge volumes of data every minute, this could be reduced to a couple of messages every five minutes, depending on the measurement use case.
This results in a massive bandwidth reduction, so the cellular network becomes cost-effective, which then reduces infrastructure costs and creates quicker ROI and value.
For businesses that decide to start with IoT, edge computing negates the need for a massively complex and costly deployment. Edge computing can provide a way to get a project up and running, deliver data points and give insight into how a business can capitalize on IoT further with a data-led strategy.
Conclusion
The vast capabilities of IoT deployments are widely publicized. Many businesses are unfamiliar with the availability of simple, affordable, entry IoT capabilities to provide data analysis down at the edge, where only the most valuable data collected is shared in real time, which makes the process more cost-effective.
Solutions for businesses like AWS and Microsoft have their place, but most businesses that don't have the huge use cases to warrant dedicated attention and support from the major players are left to their own devices. Instead, a small-scale offering that incorporates big data, edge and IoT within a small footprint will have a significant impact, which is also easily scalable without the need to overhaul existing infrastructure.
About the author
Peter Ruffley is founder of Zizo Software and has over 40 years of broad experience in the IT industry, including working with some of the biggest data technologies, such as Oracle, IBM and Ingres. With a keen interest in cloud analytics technologies, he understood the move to cloud analytics was underway and assembled a team to create a new type of technology, suited to deliver big data analytics and pattern database services at scale in the cloud.