What is AI in networking?
AI in networking involves the application of artificial intelligence technologies, including machine learning, deep learning and advanced data analytics, to automate, optimize and secure network operations.
Instead of relying on manual configuration and troubleshooting, AI in networking enables teams to sustain operational efficiency, fortify security and stay ahead in an increasingly competitive landscape.
How does AI in networking work?
Network devices produce vast amounts of data, including logs, traffic patterns and security alerts. By applying machine learning techniques to both historical and real-time data, AI systems can learn to recognize the typical patterns within a network and anticipate potential problems before they lead to disruptions.
This predictive capability enables AI to identify threats and hardware failures that might otherwise go unnoticed by human operators. As a result, network teams gain more time and the flexibility to concentrate on complex tasks that require human expertise, rather than spending time on routine monitoring with complex workflows.
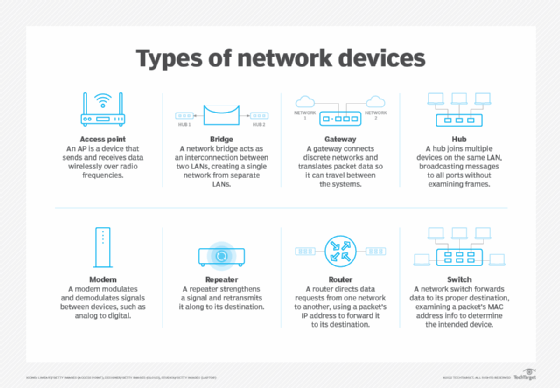
Key benefits of AI in networking
AI helps network teams boost agility and minimize repetitive, manual tasks. The following are some key benefits of incorporating AI into networking:
- Faster troubleshooting. AI quickly analyzes and resolves common issues, provides solutions, automates fixes and enables faster time to resolution.
- Scalability and agility. AI empowers networks to scale seamlessly by automating IT processes and responding dynamically to shifting requirements.
- Virtual network assistants. AI-driven VNAs support IT teams by diagnosing network problems, suggesting potential fixes, streamlining access to documentation and enhancing overall efficiency.
- Predictive maintenance. AI studies past and current network activity to anticipate potential failures. This enables teams to take preventive action in advance, reducing system downtime and ensuring smooth operations.
- Improved security. AI helps network teams by continuously monitoring traffic, detecting anomalies in real time and helping to identify potential security threats, strengthening the network's overall cybersecurity posture.
Key challenges of AI in networking
As networks become larger and more complex, engineers must manage diverse workflows and evolving demands. While AI can automate certain tasks efficiently, the following challenges still exist:
- Security and privacy concerns. Networks produce vast amounts of data every day, and incorporating AI into these systems can introduce security risks. This integration might create new vulnerabilities that attackers could exploit, potentially leading to data breaches and exposure of confidential information.
- Reliability and trust issues. AI is continually evolving and can sometimes produce inaccurate recommendations that might result in security missteps and costly errors within network environments.
- Data quality. AI models rely on data to operate with precision and reliability. When the quality or quantity of data is lacking, the system's ability to detect threats accurately or perform optimally can be significantly reduced.
- Security of AI models. AI models undergo frequent fine-tuning, but they require stronger safeguards to prevent tampering and unauthorized use and to ensure they aren't exploited for harmful activities.
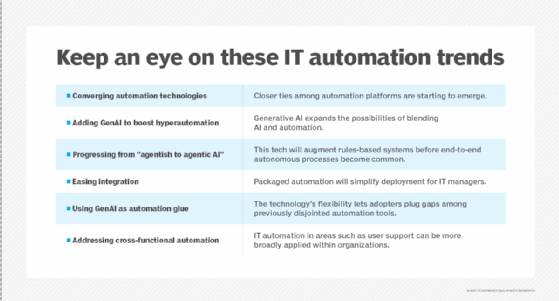
AI in networking use cases and examples
As AI reshapes how networks are built and managed, it's opening numerous real-world applications and use cases. By exploring the following examples, networking professionals can gain a strategic perspective on the industry's direction.
- VNAs. Numerous tools integrated with OpenAI APIs make it possible to work with assistants that can troubleshoot network issues and provide suggestions. For example, ChatGPT helps network teams analyze logs, provide troubleshooting steps and even write code to automate tasks.
- Network performance optimization. AI streamlines routing decisions by automating tasks such as load balancing, bandwidth allocation and quality of service management. Instead of relying on manual configurations, AI analyzes both historical patterns and real-time network data to make informed, adaptive decisions.
- Network planning and design. Network engineers can use AI to simulate various configurations and assess network performance across different scenarios. Platforms such as Mesh AI enable predictive analysis by modeling potential network setups and forecasting their behavior in different conditions.
- Log analysis. Modern networks generate millions of logs, making manual analysis impractical for network teams. AI can sift through this data, uncover patterns from historical logs and highlight opportunities for predictive maintenance, presenting the insights through clear visualizations.
- Packet analysis. Wireshark is still the go-to tool for capturing and analyzing network traffic. However, the sheer volume of data in a typical capture can make analysis overwhelming. Thanks to AI advancements, tools such as Selector Software's Packet Copilot let users interact with packet data conversationally, making the analysis process more intuitive and efficient.
- Real-time threat detection. AI can examine traffic patterns and user activity to detect unusual behavior and potential threats.
AI networking tools for businesses
The days when network teams relied solely on memorizing complex CLI commands and countless networking protocols are practically over. AI is reshaping how networks are built and managed, opening new opportunities. Examples of tools that use AI to help empower network teams include the following:
- Cisco ThousandEyes. This network monitoring tool uses AI to detect global internet disruptions and helps businesses pinpoint whether issues stem from internal systems or external providers.
- Selector Packet Copilot. Using a conversational interface powered by natural language processing to simplify packet analysis, this tool enables users to chat with an uploaded PCAP file directly through a graphical interface.
- Cisco AI Defense. This AI-driven cybersecurity tool supports network teams by identifying and mitigating attacks, regardless of their origin.
- Nokia Deepfield. This network intelligence and analytics platform provides real-time visibility into IP network traffic, security threats and performance.
AI in networking is significantly affecting industries. Businesses must actively understand and align AI with their short- and long-term objectives to achieve meaningful results, rather than passively observing its evolution.