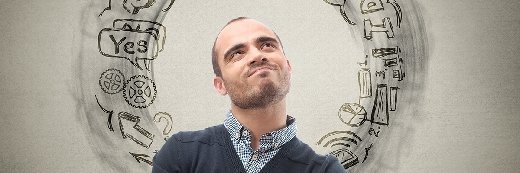
Kirill Kedrinski - Fotolia
Do you need a data warehouse for business intelligence?
Some organizations have moved away from using data warehouses in their business intelligence strategies. Read on to find out how approaches to data storage for BI are changing.
A data warehouse is a repository of data that's been generated across an organization's different applications and collected into one place. The data in a data warehouse typically has a defined purpose for analysis and has been cleaned and organized.
Because of the way data is cataloged in the repository, using a data warehouse for business intelligence has been common practice for many years. Data that's already been extensively prepared is easier for analysts to glean insights.
However, the analytics pipeline has changed in recent years. This has led to some data teams exploring options outside of the data warehouse.
Cloud and your data warehouse
Data warehouses once represented an organization's entire universe of data. And for some companies, that's still the case. What's more common is using a hybrid environment that combines private and public cloud instead of relying on an on-site data warehouse for business intelligence. These hybrid strategies are changing as well, as companies move more data and build new cloud-native applications.
SPR Consulting, a digital transformation company, has a client that insisted on keeping everything in a private cloud, but it cost the company millions of dollars to build out everything they thought they might need.
"It's interesting how some clients will use cost as an excuse [not to move to public cloud], but if you really look at it you pay more," said Erik Gfesser, principal architect at SPR.
Organizations look at how public cloud fees are charged, but they don't always consider all the factors, such as how much AWS costs per minute, Gfesser said.
Public clouds now have services for integrating on-premises and cloud data. Cloud vendors want as much of their customers' data in their cloud so they can sell those clients other services. Customers benefit too, not only from having their data and analytics in the cloud, but also by taking advantage of new services such as machine learning that give them a competitive edge.
"The right architecture for you is the one that reliably produces the information you need in a timely manner, is scalable and secure," said Kevin Wentzel, BI leader and COO at software development company Kopis. "Some organizations only need nightly refreshes or can wait two minutes for a report to run, while others require near-real-time visibility and sub-10 second visualization updates."
Nelson Ford, founder and principal solutions architect at AWS consulting firm Pilotcore Systems, said other considerations include cost, security, scalability, durability, high availability and ease of use and maintenance.
The data center's shrinking role
It's not that a data warehouse for business intelligence isn't important. The problem is the amount of data being collected, stored and analyzed is exploding, and traditional architectures can't keep up. No IT department has unlimited budgets for equipment and staff, and managing everything in-house can eventually become unwieldy and unnecessarily expensive.
"On-premises data centers are playing a diminishing role in BI due to the high costs and risks associated with building, maintaining, securing and scaling big data storage and analytics on physical servers," Ford said. "Storage is expensive, hardware can -- and eventually will -- fail, and the maintenance and security require specialized skills and constant attention."
In the hybrid cloud model, data residing on premises can be analyzed with cloud-based tools such as Amazon QuickSight or ETL tools such as AWS Glue.
Many organizations have adopted data lakes as a way of coping with the volume, variety and velocity of data, instead of using a data warehouse for business intelligence.
"The difference between a traditional data warehouse and a data lake is that you have to define everything before you load your data," Wentzel said. "There's no loading in a data lake. You're defining how to interpret that data on top of data that's already there."
Rather than building a data warehouse based on the questions a company wants answered, the trend has been toward using a data lake for data exploration.
Then there's third-party data. Companies want to be able to access the data they need regardless of where it resides.
"Organizations need to consider how they are designing and implementing data pipelines in order to fuel BI with the most current and context-aware data," said Joe DosSantos, chief data officer at Qlik.
Data warehouses aren't the only way the analytics pipeline has changed to provide better data and insights.
"Organizations are applying a variety of technologies like change data capture, data catalogs and data orchestration layers to make sure the data that's coming from these various sources is vetted, governed and trusted ahead of any analysis," DosSantos said.
On-premises vs. SaaS analytics platform options
When considering the role of the data warehouse in business intelligence, it's also important to consider where the analytics platform resides. Business intelligence vendors offer both on-premises and SaaS options.
Vendors often encourage customers to adopt SaaS versions of their products because cloud migration is a fact of life, but more importantly it tends to be a win-win for the vendor and the customer. Some SaaS vendors even include data warehousing in the cloud-based deployment.
"Unless you operate in an industry that has strict data regulations [which] limit your ability to leverage the cloud for analysis, there is no reason a new customer should not leverage SaaS," DosSantos said. "This allows you to [avoid] the headaches of hardware and operations management [and] SaaS is up and running in minutes rather than the weeks and months that most on-prem solutions require."
For existing customers, when to migrate to SaaS depends on the customer's business needs and data strategy. The migration may include a hybrid step in which some analytics are run on premises and some in the SaaS product.
"On premises can be leveraged as part of a private cloud if the customer prefers, but honestly, if they are using the cloud, even in a private model, SaaS makes much more sense to match the scalability and cost savings you're looking to achieve with the cloud model," DosSantos said.