6 top predictive analytics tools for 2024
Predictive analytics tools are evolving. Enhanced with AI, easier to use and geared to both data scientists and business users, they're more business-critical than ever.
The evolution of predictive analytics tools has made them more useful and essential to businesses than ever before. Here we profile six top tools for 2024. But, first, some important background on how predictive analytics tools have changed and best practices for choosing the right tool for the job.
What are predictive analytics tools?
The early days of analytics were dominated by analytics that helped enterprises understand what happened in the past: descriptive analytics and diagnostic analytics. Developers commonly used various BI tools to develop these models.
Predictive analytics is a complementary field aimed at forecasting what could happen in the future by analyzing patterns and trends in past and current data.
Traditionally, predictive analytics was restricted to a small team of data analysts or data scientists. Predictive modeling was a complex process that could require weeks or months of experimentation with different data sets, exploration of different hypotheses and validations of different prototypes to find a model that showed value.
This is now starting to change with dramatic improvements in the capability of tools designed for both data analytics experts and regular business users, said Carlie Idoine, research director at Gartner.
The terms used to describe the various tools for building predictive models have also evolved over the years. Today, they are commonly referred to as data science and machine learning (ML) tools. These tools are used to develop a variety of analytics and AI models used for descriptive, diagnostic, predictive and prescriptive analytics.
Predictive analytics is just one aspect of these tools. In practice, users might not even directly refer to the term when applying predictive analytics to use cases. For example, a sales manager cares about a better lead scoring algorithm, a marketing manager wants a better click-through rate and the finance team wants to reduce fraud.
Here are some other important aspects of modern predictive analytics tools to consider.
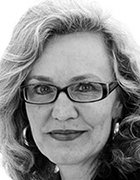
Easier to implement
The major shift in these tools is that it is getting easier to tune existing predictive modeling services or create new ones. Idoine cited as an example her early (pre-Gartner) work in building predictive models to improve logistics. Her work then required deep knowledge of how the algorithms worked and also how to code them. Today, enterprises and vendors are exploring various types of augmentation to reduce the expertise and time required for many of the steps in this process.
"You don't have an be an expert to go in and use these tools anymore," Idoine said. What used to require weeks of writing code can now be accomplished with a few mouse clicks and a lot of automation on the back end. The new capabilities of automated ML (AutoML) for short, reduce the need to deeply understand how the variables affect each other, automatically choosing the best combination of algorithms for a given task.
"It's a much more automated and augmented process, so it is more accessible," she said.
Finding the right tool for the right job
In choosing the right predictive analytics tools for the job, it is essential to identify the enterprise, business or functional needs of your use cases. It might be the case that existing tools for business intelligence, analytics or CRM already support your needs.
For example, through its Tableau acquisition, Salesforce has been developing various ways to simplify the use of predictive analytics in CRM workflows. In early 2021, it introduced business science, a new class of AI-powered analytics that surfaced predictive analytics within existing business apps. Similarly, ERP leader SAP consolidated various predictive analytics capabilities into its Predictive Analytics service back in 2015.
It might also be worth investigating new data science or industry-specific tools that might have a better set of capabilities for your industry or business area. Some predictive analytics tools focus on more generic capabilities that can be applied across all industries, and other predictive analytics tools are specific to an industry or functional area.
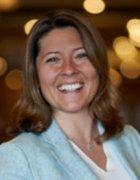
Analytics vendors are also starting to improve tools for no-code AutoML, which automates creating and testing various models from scratch for both analytics experts and business users.
"AutoML enables organizations to respond rapidly to changing requirements and constraints by expanding the ability for data and analytics teams to leverage advanced augmented analytics capabilities," said Elif Tutuk, global head of product at AtScale.
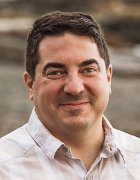
Growing economic uncertainty might also drive adoption of predictive analytics to navigate the rapid pace of change. Josh Good, vice president of strategy and marketing development at Qlik, said visual AutoML tools and the increased use of low-code/no-code cloud automations could deliver prescriptive analytics directly into workflows.
Prebuilt models, regression-based tools, ML tools
It is also worth investigating the marketplace of prebuilt models, templates and toolkits that come with the platforms, Idoine advised. These products can dramatically simplify predictive analytics for industry-specific problems using best practices culled from years of experience.
"It is one thing to have technology that's accessible but even better to know how to apply that technology to specific problems within an industry or a functional area of the organization," she explained.
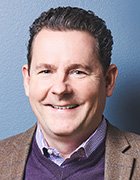
Donncha Carroll, partner at Lotis Blue Consulting -- formerly Axiom Consulting Partners -- underscored the importance of knowing the difference between traditional regression-based predictive analytics tools and ML-based tools.
Regression models essentially represent or encapsulate a mathematical equation that approximates the interactions between the different variables being modeled. ML models use and train on a combination of input and output data, and they use new data to predict the output.
Experts or collaborators?
Finally, it's important to know who will be using these tools, Idoine said. Traditionally, Gartner considered all data science and machine learning platforms as a single market. But Idoine sees a bifurcation in the data science and ML market driven by the needs of hardcore analytics programmers versus citizen data scientists. Hardcore analytics programmers are looking for tools to augment data discovery, data preparation and model development. Citizen data scientists are looking for tools that provide guardrails for common business requirements and support collaborative development across teams. Gartner classifies these two types of tools as "multipersona data science and machine learning platforms" and "data science and machine learning engineering platforms."
Predictive analytics tools for 2024
In alphabetical order, here are six of the most popular predictive analytics tools to consider.
Editor's note: This unranked list is based on input from Gartner, Forrester Research and other industry experts.
1. Altair AI Studio
The Altair RapidMiner platform includes a comprehensive set of predictive analytics tooling around its core data mining and text mining strengths, with Altair AI Studio supporting model development by data scientists. The platform's core capabilities simplify extracting data from a diverse set of sources, cleaning it and incorporating it into various predictive modeling workflows. Altair offers free trials of Altair AI Studio and the platform's other core products that help anyone get started and learn the basics. A notebooks feature simplifies the development of predictive analytics models for both novices and experts alike. The company also provides various augmented capabilities for data preparation with Turbo Prep, model generation with Auto Model and model operations for deployment, monitoring and management of machine learning models. A generative AI extension enables users to build their own large language models and access open source LLMs on the Hugging Face community. The platform also supports various explainability and governance features when required.
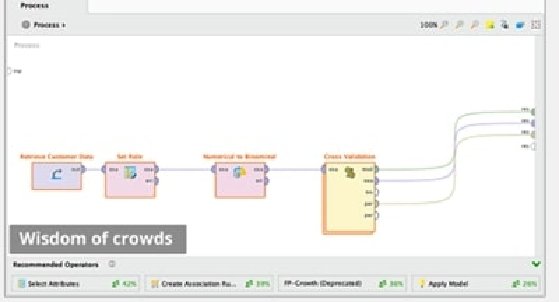
2. H2O Driverless AI
A relative newcomer to predictive analytics, H2O gained traction with a popular open source offering. The company's H2O Driverless AI simplifies AI development and predictive analytics for both experts and citizen data scientists through open source and custom recipes. Of note are various automated and augmented capabilities for feature engineering, model selection, parameter tuning, natural language process, and semantic analysis. The company also offers a variety of capabilities to simplify development of explainability into predictive analytics models using causal graphs, local interpretable model-agnostic explanations, the Shapley value and decision tree surrogate methods.
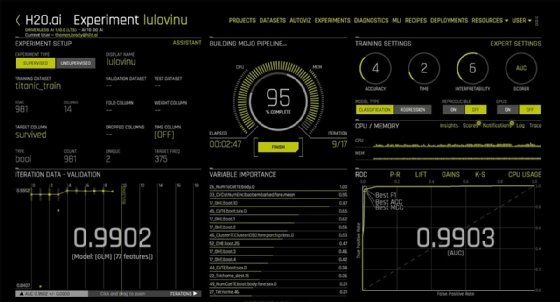
3. IBM Watson Studio
IBM became a leading predictive analytics tools vendor with the acquisition of Statistical Package for the Social Sciences (SPSS) in 2009. SPSS was founded in 1975 and grew into one of the top statistical and analytics packages over the years. IBM continued to innovate the vendor's core capabilities and integrated them into its more modern Watson Studio on the IBM Cloud Pak for Data platform. This consolidated offering combines a broad range of descriptive, diagnostic, predictive and prescriptive analytics functions. The platform simplifies predictive analytics for expert data scientists and improves collaborative data science for business users. The platform also includes various features to enhance responsible and explainable predictive models.
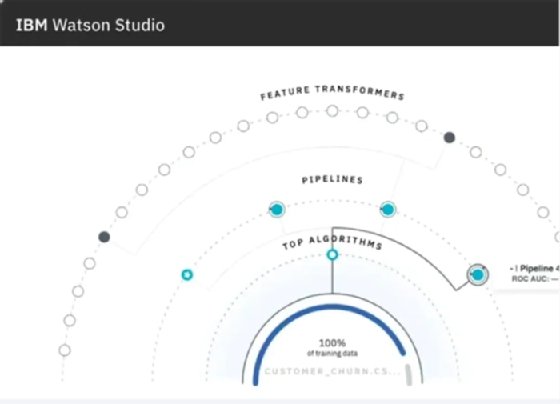
4. Microsoft Azure Machine Learning
Microsoft has long been a leader in various analytics capabilities through its Power BI analytics platform and Excel, which has become the analytics front end of choice for most business users. The company's Azure Machine Learning complements these core tools with capabilities for managing the complete predictive analytics lifecycle. Supporting tools include Azure Data Catalog, Azure Data Factory and Azure HDInsight.
The company supports all types of users, from expert data scientists to business subject matter experts. It also provides strong integration with its various application development and RPA tooling, which makes it easier to deploy predictive analytics capabilities directly into applications and business workflows.
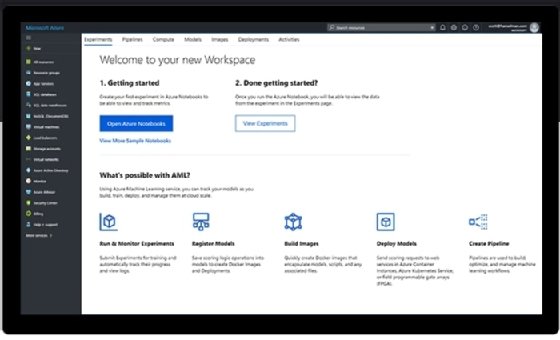
5. SAP Predictive Analytics
SAP Predictive Analytics is a good example of how enterprise application platforms can extend their core offerings to support predictive analytics workflows. The tool is a good choice for enterprises with an extensive SAP deployment, particularly those looking to create predictive analytics for logistics, supply chain and inventory management use cases. The current offering was released in 2015 and built on two prior tools first released in 2012. The tool supports advanced and business users through various features that simplify data aggregating, predictive modeling and model analysis across separate user interfaces. Automated analytics helps business users with data preparation, modeling, social graph analysis, recommendation and predictions. Expert analytics helps experts explore various statistical techniques, visualizations and code applications using the R programming language.
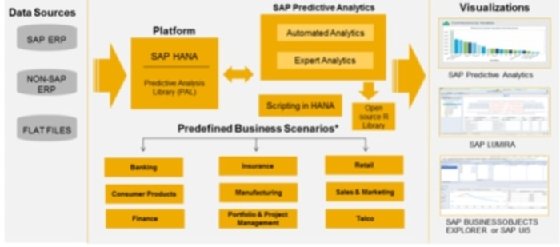
6. SAS
SAS Institute is one of the oldest statistical analytics tool vendors. The first version of the company's tools started in 1966 as part of a U.S. government initiative to improve data analysis for healthcare. The company was officially launched in 1972 after its government contract ran out. It has continued to innovate various tools used by statisticians and data scientists. The company is a clear leader in all kinds of analytics tools and techniques, including predictive analytics.
More recently, the company has modernized its core tool sets with various data science and machine learning workflows that take advantage of modern data stacks, augmented workflows and simplified deployment. The company has hundreds of tools for various domains. Core offerings for predictive analytics include SAS Visual Data Science, SAS Data Science Programming, SAS Visual Data Decisioning and SAS Visual Machine Learning. The company also maintains strong relationships with leading cloud providers and enterprise software platforms to simplify predictive analytics development and deployment across various workflows.
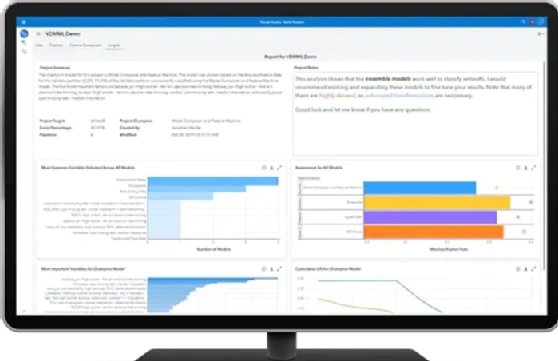
George Lawton is a journalist based in London. Over the last 30 years, he has written more than 3,000 stories about computers, communications, knowledge management, business, health and other areas that interest him.