The differences between a data warehouse vs. data mart
Data marts and data warehouses both play key roles in the BI and analytics process. Here's how they differ and how they can be used to help drive business decisions.
During the early stages of my IT career, there was no concept of organizational data sharing or an understanding of data's global value to the enterprise. IT departments primarily designed and administered applications that focused on day-to-day business operations. Although IT produced reports to facilitate business decision-making, data was only offloaded to separate environments when queries began to negatively affect the performance of operational systems.
As reporting systems matured and their popularity skyrocketed among business units, enterprises realized that the real value of all the data they generated was its ability to help drive business decision-making. Once disparate departmental data stores came to be seen as strategic assets that would provide intrinsic benefits at an organizational level, IT teams began to combine their contents in data warehouses -- and eventually data marts.
Both data warehouses and data marts are special-purpose platforms used to ingest, store and process data for BI and analytics applications. The primary difference between them is data warehouses are centralized repositories that typically store data from multiple business units and subject areas, while data marts are built for individual units or groups of users. In their purest form, data warehouses support decision-making at an enterprise level and data marts do so at a departmental level.
The challenge with attempting to define and compare a data warehouse vs. data mart is the criteria used to categorize them can be somewhat fluid. There are departmental platforms that contain large amounts of data from different source systems. Although they might meet the data mart criteria of providing decision-making information to a specific department, their size and the high level of detailed data they hold could also categorize them as data warehouses.
But to help make the differences between the two approaches to storing analytical data clearer, let's look more closely at the characteristics and attributes that generally set data marts and data warehouses apart and how they separately fit into an overall data management strategy.
Data warehouses
As mentioned above, the main goal of a data warehouse is to provide a centralized data repository that enables more informed and insightful decisions at an enterprise level. From C-level executives to business managers, business analysts, operational workers and others, data warehouses serve a wide and varied user base.
Virtually any data the organization creates or collects could potentially be ingested into a data warehouse. Data managers, data warehouse analysts and other IT specialists often perform a high level of analysis to identify and evaluate potential data sources and then work to integrate, consolidate and cleanse the data sets being ingested.
A key benefit of data warehouses for BI and analytics uses is their ability to provide a global view of customers, suppliers, service providers and business partners that have relationships spanning multiple lines of business.
Data marts
The primary use case of a data mart is to meet the needs of users requiring access to more granular data sets in a particular subject area. The goal is to provide those users with fast access to the data that is most relevant to their business and information needs.
A good example is an organization's sales department. The department manager needs to see data on products, customers and the sales team's performance metrics. The amount of time it would take to access and analyze the data in an enterprise data warehouse is longer and less efficient than using a repository purposely designed to meet the unit's specific business needs.
In addition, data marts often differ from data warehouses in the type of data they store. Many contain summary data to accelerate analysis and reporting, as opposed to the full detailed data sets. In such cases, the data is refined and customized to meet the specific needs of the target audience. Data mart administrators also build additional logical and physical constructs to speed data access performance.
One of the traditional sources for a data mart is a centralized data warehouse. Because data warehouses contain data at an enterprise level, they're excellent sources for feeding data marts. But data marts often also take feeds from other decision-support data stores and from operational systems.
Data mart vs. data warehouse
To help determine whether a data warehouse or a data mart -- or some combination of the two approaches -- best meets your organization's needs, here's a more detailed comparison of how they differ and what each one offers to users:
- Size/data volume. A data warehouse typically contains at least 100 GB of data, and many have terabytes or more -- often much more. Data marts can also hold terabytes of data but are usually smaller than data warehouses. One exception is that a data mart in a large organization might well be bigger than a data warehouse in a smaller one.
- Focus and scale. An enterprise data warehouse provides an enterprise-wide view of an organization's business operations, while a data mart delivers a more granular view of a specific business unit, subject area or other aspect of operations. In many cases, a data mart is a subset of the data warehouse in an organization.
- Data sources. Data warehouses commonly store data from various business applications and systems throughout an organization. A data mart has a more limited number of source systems related to its specific focus, or it might be fed directly by a data warehouse. Both types of repositories can also store external data sets needed for analytics uses.
- Ownership and control. A centralized data warehouse is funded, deployed and managed at the enterprise level. Business units might still own their data, but IT or a central data management team oversees the data warehouse, and the CIO or chief data officer usually is responsible for it. Data marts generally are controlled by the department or business unit they're built for, although central IT or data management staffers might help manage and support a data mart.
- Ease of user access. Access to a data warehouse tends to be tightly controlled because of its enterprise nature, with users limited to data sets that are relevant to their roles. In addition, using a data warehouse can be more suited to skilled analytics professionals than business users. A data mart is generally designed for easier access and use by business analysts and other end users in a business unit, as well as BI and data analysts assigned to the unit.
- Decision-making use cases. Both data warehouses and data marts enable BI and analytics applications that can help organizations make better tactical and strategic business decisions. But a data warehouse can be used to support decision-making for individual business units and an organization as a whole, while the use of a data mart is usually limited to a single unit. Data marts are also more suited to aiding in operational decision-making than data warehouses.
- Speed of decision-making. The size of data warehouses and the breadth of the data sets they contain complicate the data analysis process. It can take longer to run queries, analyze data and create reports before the results are available for use by decision-makers. Because data marts are more narrowly focused, they tend to enable a shorter lead time on decision-making uses.
- Startup and support costs. Not surprisingly, a data warehouse likely will have higher development, deployment and support costs than a data mart. That applies to both on-premises and cloud-based platforms. However, if an organization has various data marts for different business units, the combined cost of deploying and supporting them can add up.
- Development and build time. Building a data warehouse is often a big-budget, multiyear project. A data mart is more likely to take months or maybe just weeks to build. Again, though, creating a series of data marts for different units in an organization can be a longer process.
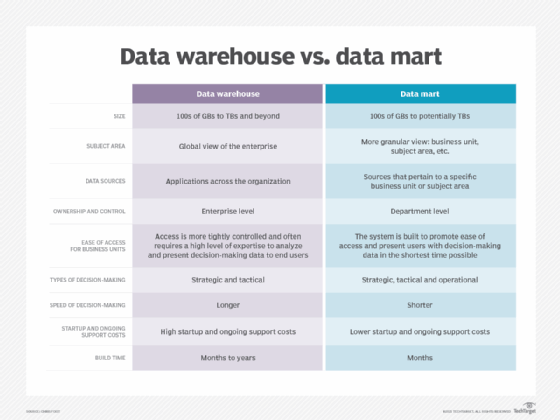
Justifying data warehouse and data mart deployments
At this point, most senior management teams have a basic understanding of data warehouses and the business benefits they provide. But it can still be a big challenge for IT and data management leaders to justify the initial deployment and ongoing support costs for a data warehouse. In doing so, you'll need to incorporate not only back-end infrastructure and administration costs, but also the cost of continuously identifying and integrating new data sources from across the enterprise and refining existing data sets to support new applications.
If your organization already has a data warehouse, justifying the costs of building and supporting additional data marts can also be challenging. The first question business executives usually ask is why you can't just use the data warehouse for all BI and analytics applications. Your team should be prepared to describe the differences between the two types of data stores and educate the execs on how a data mart's more granular focus can provide additional benefits by meeting the specific information needs of a business unit or a group of users.