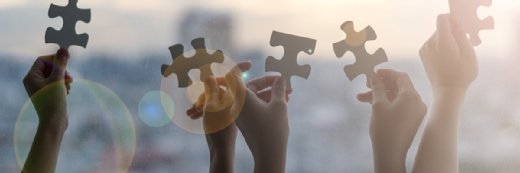
Khunatorn - stock.adobe.com
Collaborative analytics model boosts decision-making
Organizations are adopting a collaborative analytics model to tap the full potential of their workforces and increase data sharing and decision-making through collaboration.
The rise in remote work and efforts to democratize data science are driving organizations to consider a collaborative analytics model that improves cooperation during the decision-making process.
It is still early as organizations work through some of the data sharing, security and workflow challenges of analytics collaboration. However, popular analytics tools are adding support for better collaboration features, and enterprises are beginning to connect the dots between data sources, analytics tools and collaboration platforms.
"To be really data-driven, an organization must tap into the knowledge, perspectives and goals of all their employees, not just those with data modeling or technological skills," said Eric Madariaga, CMO at CData Software.
Organizations can only gain actionable insights into their business by leveraging departmental stakeholders' expertise on their data.
"Firms that are reaping the greatest benefits from data-driven decision-making have deployed collaborative analytics and cultivated an open data-driven culture," Madariaga said.
What is a collaborative analytics model?
A collaborative analytics model changes the way organizations approach their BI initiatives by opening data-driven analysis and decision-making to more members before the process concludes. This allows organizations to do more than simply react to what's already happened.
"The traditional approach to business intelligence has too often reported on what happened rather than what's happening or likely to happen," said Satya Sachdeva, vice president of insights and data at Sogeti, part of Capgemini.
This can enable organizations to peek into the future with predictive and prescriptive analytics. Predictive and prescriptive analytics are advanced practices that weave together data from across the enterprise. Collaborative analytics can help organizations optimize business functions, discover new business models and create new products and services to monetize data.
Predictive analytics attempts to predict what happened based on models trained on past data. Prescriptive analytics goes a step further to recommend actions. This can be as simple as a product recommendation engine or as complex as a better supply chain plan considering new circumstances.
A vital aspect of both disciplines is taking advantage of more contextual data than required with traditional BI. Various types of subject matter experts can provide insight into the appropriate context for various aspects of the model.
For example, a better recommendation engine might involve collaboration between product marketing experts, customer service reps, data scientists and UI experts. Collaborative analytics tools can help streamline communication across different experts so each person can focus on the relevant detail without getting bogged down in file formats, data schemas and other details.
"Analytics collaboration is the ability for organizations and business users to collaborate on discovering insights and taking actions to create better business outcomes," Sachdeva said.
Types of collaborative analytics
One of the most essential practices for collaborative analytics is creating a business intelligence competency center. This can facilitate collaboration among IT, organizational change management and business functions to evangelize analytics, foster innovation, monitor results and continuously enhance analytical capabilities, Sachdeva said. Some examples of analytics collaboration include the following:
- Collaboration between IT and business users to discover new data sets, use cases and define requirements.
- Visual collaboration to model data and insights among various business functions -- such as product design, sales, marketing and service -- to find avenues to monetize data for new products and services.
- Collaborative workflows among business users to save and reuse data sets and insights.
- Chats and comments among different user groups to make comments, ask questions and annotate various aspects of analytics.
How can organizations use a collaborative analytics model?
With collaborative analytics, companies will be able to shift from their traditional approach, focusing on accurate reporting, to making critical strategic and operational decisions.
This is becoming increasingly important as black swan events -- random or unexpected incidents with widespread ramifications -- are increasing in frequency. For example, all organizations most recently had to quickly adapt to the effects of the COVID-19 pandemic.
A collaborative analytics model can help organizations adapt to these situations by involving more members of an organization earlier in the process. Analysis isn't just conducted at the end, once dashboards and reports have been developed. Instead, this approach enables decision-making earlier than what was previously possible because information is more readily available and shared throughout the process.
"Few business decisions are made independently, and the more collaboration that can take place throughout the analytics lifecycle, the better the data-driven decision-making," said Elif Tutuk, vice president of innovation and design at Qlik.
Collaborative analytics represents a spectrum of depth and breadth of collaboration. At one end, organizations may make it easier for end users to build and share analytics products. On the other, collaborative analytics can make it easier for managers, employees and users to build on each other's insights.
"With a collaborative approach, an organization can be truly data-driven as data flows between the departments more easily, and different teams can use it for their gain," said Maciej Zawadzinski, CEO of Piwik Pro.
Each department's insights are then shared, so other parts of the organization can benefit from them. For example, employees might give context to the data and analytics by adding in-software notes to specific dates in the reports, such as when significant changes to the website were made or a new campaign launched. This lets everyone in the organization better understand the changes in the data.
The government of the Netherlands uses Piwik to monitor and optimize the performance of their websites across different types of expertise, for example. Using a collaborative analytics model, the board of directors evaluate the overall page performance, and copywriters use the data to optimize content.
Options for collaboration tools
All the major analytics platforms are adding various capabilities to facilitate collaborative analytics. Qlik has a major initiative focused on active intelligence, which combines a real-time data pipeline with action-oriented analytics capabilities that help drive immediate action. Qlik Sense builds on this with intelligent alerting, collaboration and embedded analytics to help people refine automated triggers and responses.
Tableau is improving analytics collaboration capabilities with features such as a "collections" tool to simplify analytics content creation by allowing users to curate content and easily share it with colleagues. It is also democratizing analytics with natural language processing (NLP) through offerings such as Ask Data and Explain Data, bringing analytics to less sophisticated users. Its latest release supports tighter integration with the Salesforce platform, which aims to streamline the analytics development and sharing of insight. For example, it recently added a new integration to help people collaborate on sustainability analytics on the Salesforce NetZero Cloud.
Microsoft Goals is a new tool to help teams curate metrics and share the process of analytics projects. And ThoughtSpot uses NLP to make sharing and finding analytics across the organization easier using simple search queries.
Beware of collaboration security concerns
Down the road, organizations would like to achieve the same ability to build on each other's work as they do with different types of productivity and content collaboration platforms. Content collaboration platforms are starting to implement some of the rich collaborative and linking capabilities envisioned by the original memex.
But collaborative analytics must contend with more nuanced data security concerns. Some of an organization's most valuable analytics insights are built on data that needs protection from rogue employees, hackers and carelessness. Businesses also have to contend with regulations such as HIPAA, GDPR and CCPA.
"It is important for companies to strike the appropriate balance of protective, restrictive cybersecurity policies when developing their analytics collaboration strategies," Sachdeva said.
These policies may seemingly impede collaborative efforts if applied with a broad brush, but they can also help to prevent data security issues that quickly arise when granting multiple parties access to confidential data.
Some organizations are turning to role-based security policies to help manage analytics collaboration, Sachdeva said. This safeguard allows only the necessary business users to have access to underlying data sets to help prevent potential breaches.
Larger organizations can also grant access via single sign-on infrastructure, Zawadzinski said. This policy makes it easier for users to log in and reduces the risk that an account is forgotten during employee offboarding.