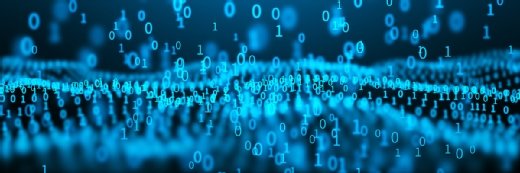
Getty Images/iStockphoto
Understanding the benefits of a data quality strategy
A data quality strategy can improve an organization's ability to generate value from data, but determining quality depends on the processes and use cases.
Data quality is essential to enabling businesses to see returns on data collection and analysis investments.
Data quality is the degree to which a data set and the process that produces it is fit for its purpose. Accuracy is one significant component of data quality and reflects the degree to which the data conforms to the correct value of a standard.
It is essential to keep in mind that data quality is highly application and use case specific. A data set collected as part of one process may or may not reflect the appropriate data quality and accuracy for another use case. Organizations can start by understanding the benefits of data quality and considering their unique needs.
Why is data quality important?
Data is now universally recognized as a value multiplier that drives growth and innovation, according to Marco Vernocchi, global chief data officer at EY, a professional services network.
Data quality attains greater significance in this context, especially as organizations increasingly collect, organize, analyze and commercialize their data assets for a competitive advantage. For organizations, data quality is as much a process as a measure.
"Data quality is a core data management discipline that employs processes and capabilities to ensure fitness of data for its intended use," Vernocchi said.
When transforming into a data-driven organization, it is critical that these capabilities are standard, but still customizable for greater adoption across the enterprise. One of the most important aspects of data quality is that clean, consistent and accurate data instills greater trust in the organization and enables informed decision-making with high confidence.
How do you define data quality?
Every organization defines data quality differently depending on its goals, its tolerance for errors and the potential upside of a given use case. For example, a healthcare facility has a much lower tolerance for errors than an e-commerce business.
Understanding what the data is and how it might evolve are key to monitoring and benefiting from data quality. It also depends on how data is being used. Is directionally accurate data good enough or is more specific data required? Are there fault tolerances to consider? For example, in an IoT situation, consider whether actual valves might blow or portions of the grid might drop.
"Businesses should apply a pragmatic approach to data quality that will align with their core business goals," Vernocchi said.
6 dimensions of data quality
To see the benefits of a data quality strategy, organizations must start by defining a robust framework that lays out a foundational set of data quality dimensions. This typically includes accuracy, completeness, consistency, conformity, integrity and timeliness. The importance of these dimensions can vary depending on the data entity, use case and investment budget under consideration.
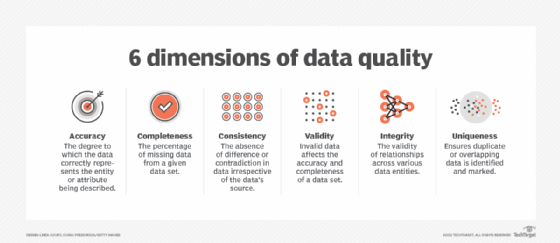
For instance, all of these elements might be critically important in an emergency room application for capturing health telemetry data. However, a gaming company developing different options based on gamer telemetry might see good results even if some of the data were missing -- particularly if it would require significant investment to chase down the missing data.
Organizations must evaluate their industry and data needs to adequately determine the requirements for the six dimensions of data quality and the best application for each unique use case.
Building a flexible methodology to assess the data quality confidence scores against these dimensions is also essential. For example, businesses should try to avoid the trap of zero tolerance for data errors.
"Though relevant in specific scenarios, a zero-tolerance approach often provides diminishing returns beyond a certain data quality threshold," Vernocchi said.
Each organization must also determine if its data is fit for the purposes and the context for which it is used. The best team to discern a data element's quality is one with the greatest familiarity with the context in which the data was collected, how it was collected and what it was trying to accomplish.
"Data users will always be the best judges of data accuracy," said JP Romero, technical manager of the enterprise information management practice at Kalypso, a digital technology and consulting company.
Focusing appropriate efforts
It is helpful to measure data quality issues as noise, which can help characterize the system's ability to adapt.
"Prioritize where data has to be pristine and where some noise is acceptable, and be upfront about the levels of risk you're willing to accept," said Alicia Frame, director of graph data science at Neo4j, a graph database company.
It is essential to know and document when pristine data is needed, and be clear when sorting through an ocean of data to find the desired information. For example, if critical investment decisions are based on 10 data points, those data points must be correct. If an organization is trying to draw conclusions from 10 billion data points, it is OK for some of them to be noise.
Be honest about the uncertainty and gaps in the data, Frame said. It is better to report a margin of error rather than overcommit to a single number.
One helpful strategy is to align data quality standards and approaches with the business value and goals for any given business process, said Satya Sachdeva, vice president of insights and data at Sogeti, an IT consulting company and part of Capgemini. Organizations need to set distinct data quality goals for each data category and focus efforts accordingly to achieve complete data quality.
For example, a financial organization calculating vested balance for retirement plans must have accurate hire date and date of birth data. Similarly, marketing teams need accurate contact information for mail campaigns to help reduce waste -- and thus cost.
"The best way to begin modernizing an organization's data management is to begin with modernizing how it aggregates and standardizes the data that needs to be managed, mastered, quality checked and governed," said Faisal Alam, technology consulting markets leader at EY.